In the fast-evolving customer service landscape, the integration of machine learning analytics has become a game-changer. This revolutionary technology harnesses the power of data analytics and machine learning to transform customer interactions and improve service quality.
By employing advanced techniques like sentiment analysis and predictive analytics, businesses can gain deep insights into customer behavior and preferences, enhancing customer satisfaction and loyalty.
Several tools and techniques are utilized in machine learning analytics for customer service. These include sentiment analysis, which gauges customer emotions and attitudes from text or speech data, and machine learning data analysis examples, which illustrate practical applications of these technologies.
By leveraging machine learning analytics tools, companies can streamline operations, enhance agent performance, and deliver a superior customer experience.
Knock Knock! Convin’s ML solutions are here to improve your customer service!
What is Machine Learning Analytics?
Machine learning analytics is an advanced analytical method that employs machine learning algorithms to analyze vast amounts of data, uncover patterns, and make predictions. This technique combines the computational power of machine learning with the systematic approach of data analytics to provide actionable insights that drive decision-making processes across various domains.
Machine Learning Analytics in Customer Service
Machine learning analytics is a sophisticated approach that combines machine learning and data analysis to extract meaningful patterns and insights from vast datasets. In customer service, this involves analyzing customer interactions across various channels, such as calls, emails, and chats, to identify trends, detect issues, and optimize service delivery.
For instance, machine learning predictive analytics can forecast customer needs and preferences, allowing businesses to address concerns and personalize interactions proactively.
Importance of Machine Learning in Enhancing Customer Service
The importance of machine learning in enhancing customer service cannot be overstated. By integrating machine learning analytics, businesses can achieve several key benefits:
1. Improved Customer Insights: Machine learning analytics deeply understands customer preferences and behavior. This allows businesses to tailor their services to meet individual needs effectively.
2. Enhanced Predictive Capabilities: With machine learning predictive analytics, companies can anticipate and address customer issues before they escalate. This proactive approach helps in reducing churn rates and improving customer retention.
3. Increased Efficiency: Automation through machine learning reduces the burden on customer service agents, allowing them to focus on complex queries that require human intervention. This boosts overall productivity and efficiency.
4. Real-Time Decision Making: Machine learning analytics enables real-time monitoring and analysis of customer interactions. This facilitates immediate responses to customer inquiries and enhances the quality of service provided.

5. Personalized Customer Experience: Businesses can offer personalized recommendations and solutions by analyzing past interactions and predicting future behavior, leading to a more satisfying customer experience.
How Machine Learning Analytics Transforms Customer Service?
Machine learning analytics revolutionizes customer service by providing deeper insights, enabling predictive capabilities, and enhancing operational efficiency. Businesses adopting these advanced analytics techniques can expect significant improvements in customer satisfaction, retention, and overall service quality.
1. Enhanced Understanding of Customer Sentiment: Through sentiment analysis machine learning, businesses can gauge customer emotions by analyzing text data from customer reviews, social media, and support interactions.
2. Predictive Insights: Machine learning predictive analytics empowers businesses to foresee customer behavior and trends. For example, predictive analytics can forecast which customers will likely churn, enabling proactive retention strategies.
3. Personalization: Machine learning data analytics enables personalized customer experiences by analyzing individual customer data and tailoring recommendations based on past behavior and preferences.
4. Operational Efficiency: By utilizing machine learning analytics tools, businesses can automate routine tasks such as ticket classification and routing, freeing human agents to focus on more complex issues.
5. Real-Time Decision Making: Machine learning analytics facilitates real-time data analysis, allowing businesses to make instant decisions that improve customer interactions.
6. Continuous Improvement: Machine learning's iterative nature ensures that customer service processes continuously improve. A machine learning data analysis example includes models that evolve with new data, refining their accuracy and relevance over time.
Critical Components of Machine Learning Analytics in Customer Service
Machine learning analytics in customer service comprises several vital components that enhance the overall customer experience. These components include:
1. Data Collection and Integration: The first step involves gathering data from customer touchpoints such as emails, chats, social media, and phone calls. This data is then integrated into a centralized system for analysis.
2. Data Preprocessing: The collected data must be cleaned and formatted before analysis. This step involves removing inconsistencies, filling in missing values, and transforming the data into a suitable format for machine learning algorithms.
3. Algorithm Selection and Training: Machine learning algorithms are selected based on the specific needs of the customer service operation. These algorithms are then trained on historical data to learn patterns and relationships.
4. Model Deployment and Monitoring: The machine learning models are deployed to process real-time customer data once trained. Continuous monitoring ensures the models perform accurately and make necessary adjustments based on new data.
5. Feedback Loop and Continuous Improvement: Machine learning models improve over time by incorporating feedback from their performance. This iterative process helps in refining the models for better accuracy and efficiency.
Sentiment Analysis in Machine Learning
Sentiment analysis in machine learning identifies and categorizes opinions expressed in text data to determine the writer's attitude toward a particular topic, product, or service. It involves several steps.
1. Text Preprocessing: This step is crucial for preparing the data for analysis. The text data is cleaned by removing stop words, punctuation, and special characters.
2. Feature Extraction: Techniques like tokenization, stemming, and lemmatization extract features from the text. These features are then used to train machine learning models.
3. Model Training: Sentiment analysis models, such as Naive Bayes, Support Vector Machines, or deep learning models like LSTM, are trained on labeled data where the sentiment is already known.
4. Prediction and Analysis: The trained models are used to predict the sentiment of new text data. This helps businesses understand customer emotions and adjust their strategies accordingly.
Predictive Analytics and its Applications
Predictive analytics uses statistical techniques and machine learning algorithms to analyze historical data and predict future events. It can be applied in various ways in customer service.
1. Churn Prediction: Predictive analytics can analyze past customer behavior to identify customers likely to churn, allowing businesses to take proactive measures to retain them.
2. Demand Forecasting: Predictive models can forecast customer demand, helping businesses manage resources efficiently and meet customer expectations.
3. Personalized Recommendations: Predictive analytics can analyze customer preferences and behavior to provide personalized product or service recommendations, enhancing the customer experience.
4. Operational Efficiency: Predictive analytics can optimize workforce management by forecasting peak times for customer inquiries ensuring adequate staffing levels.
This blog is just the start.
Unlock the power of Convin’s AI with a live demo.

Comparison: Predictive Analytics vs. Machine Learning
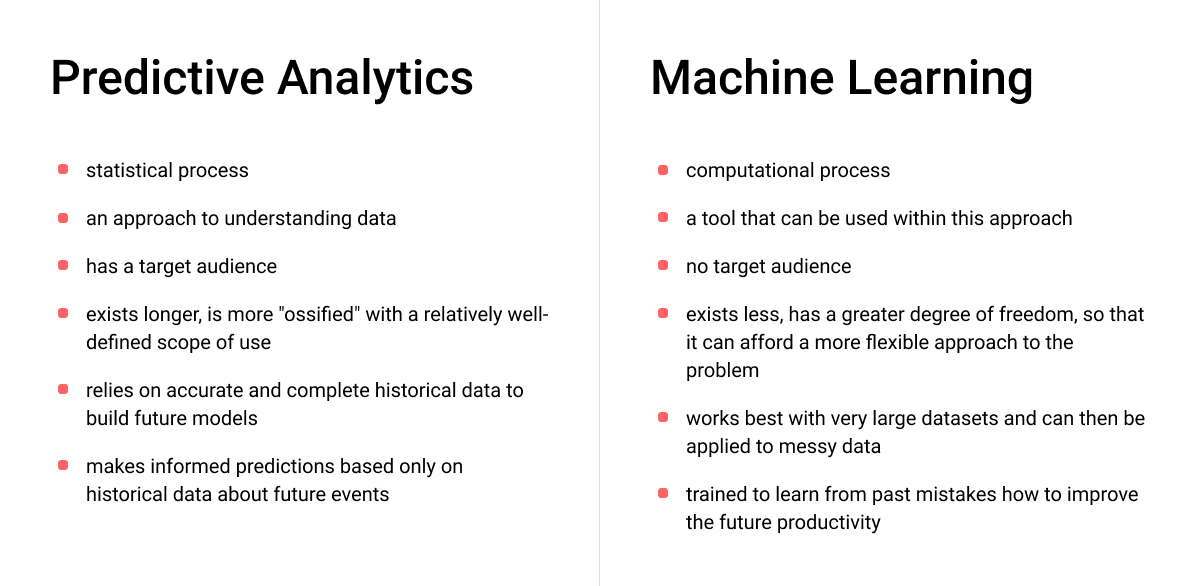
The terms predictive analytics and machine learning are often used interchangeably, but they have distinct differences:
- Predictive Analytics is a broader field encompassing various statistical and analytical techniques, including machine learning, to predict future events. It uses historical data to forecast future outcomes and is typically used for specific business problems like demand forecasting or risk assessment.
- Machine Learning is a subset of artificial intelligence that involves developing algorithms to learn from and make predictions based on data. Machine learning focuses more on building models that can automatically improve over time as they are exposed to more data.
Key Differences
- Scope: Predictive analytics includes many techniques, while machine learning is specifically about developing algorithms that learn from data.
- Approach: Predictive analytics often uses machine learning as a tool but also relies on traditional statistical methods. Machine learning is centered on creating models that adapt and improve automatically.
- Application: Predictive analytics is generally used for specific forecasting purposes, while machine learning can be applied to a broader range of tasks, including image recognition, natural language processing, and more.
Machine Learning Analytics Tools and Techniques
Machine learning analytics tools are essential for processing large datasets, extracting meaningful insights, and driving decision-making in customer service. These tools leverage machine learning techniques to automate and enhance the analytical process, providing businesses with powerful capabilities to understand and predict customer behavior.
Popular Tools Used in Customer Service
1. TensorFlow: Google's open-source machine learning framework, TensorFlow, is widely used for building and deploying machine learning models. It supports a range of tasks, from sentiment analysis to predictive analytics.
2. Scikit-Learn: A Python library for machine learning, Scikit-Learn is known for its simplicity and efficiency. It offers various data mining and analysis tools, making it ideal for predictive analytics and machine learning data analytics.
3. IBM Watson: IBM Watson provides a suite of AI and machine learning tools that enable businesses to analyze customer interactions and derive actionable insights. It is particularly effective for natural language processing and sentiment analysis machine learning.
4. Amazon SageMaker: This fully managed service by AWS allows developers to build, train, and deploy machine learning models at scale. It is commonly used for customer service data analytics and machine learning tasks.
5. H2O.ai: An open-source platform for AI and machine learning, H2O.ai offers tools for predictive analytics, machine learning data analysis, and real-time data processing.
Applications of Machine Learning Analytics in Customer Service
Machine learning analytics transforms customer service by providing insights that drive better decision-making and improve customer experiences. Here are some real-world applications and case studies:
Real-World Examples and Case Studies
1. Sentiment Analysis: Companies use sentiment analysis machine learning to gauge customer emotions from text data in reviews, social media posts, and support tickets.
For example, a telecommunications company implemented sentiment analysis to identify dissatisfied customers and proactively address their issues, resulting in a 20% increase in customer satisfaction.
2. Predictive Analytics: Predictive analytics helps businesses forecast customer behavior and trends. An e-commerce company used machine learning predictive analytics to predict which products customers would likely buy next, leading to a 15% increase in sales through personalized recommendations.
3. Automated Support: Machine learning analytics tools enable automated customer support by classifying and routing customer inquiries to the appropriate department. A software company reduced its average response time by 30% using machine learning models to automate initial support triage.
How Convin Uses Machine Learning for Data Analytics?
Convin, a leading AI-backed contact center software, leverages machine learning analytics to enhance customer service through several critical applications
1. Conversation Intelligence: Convin records, transcribes, and analyzes customer conversations using machine learning models. This process highlights performance insights and identifies areas where agents can improve, leading to higher customer satisfaction.
2. Sentiment Analysis: Convin can assess customer emotions during interactions by applying sentiment analysis. This enables agents to respond empathetically and effectively, improving customer experience and loyalty.
3. Predictive Insights: Convin's machine learning predictive analytics provides insights into customer behavior, helping businesses anticipate customer needs and proactively address potential issues. This predictive capability enhances customer retention and reduces churn rates.
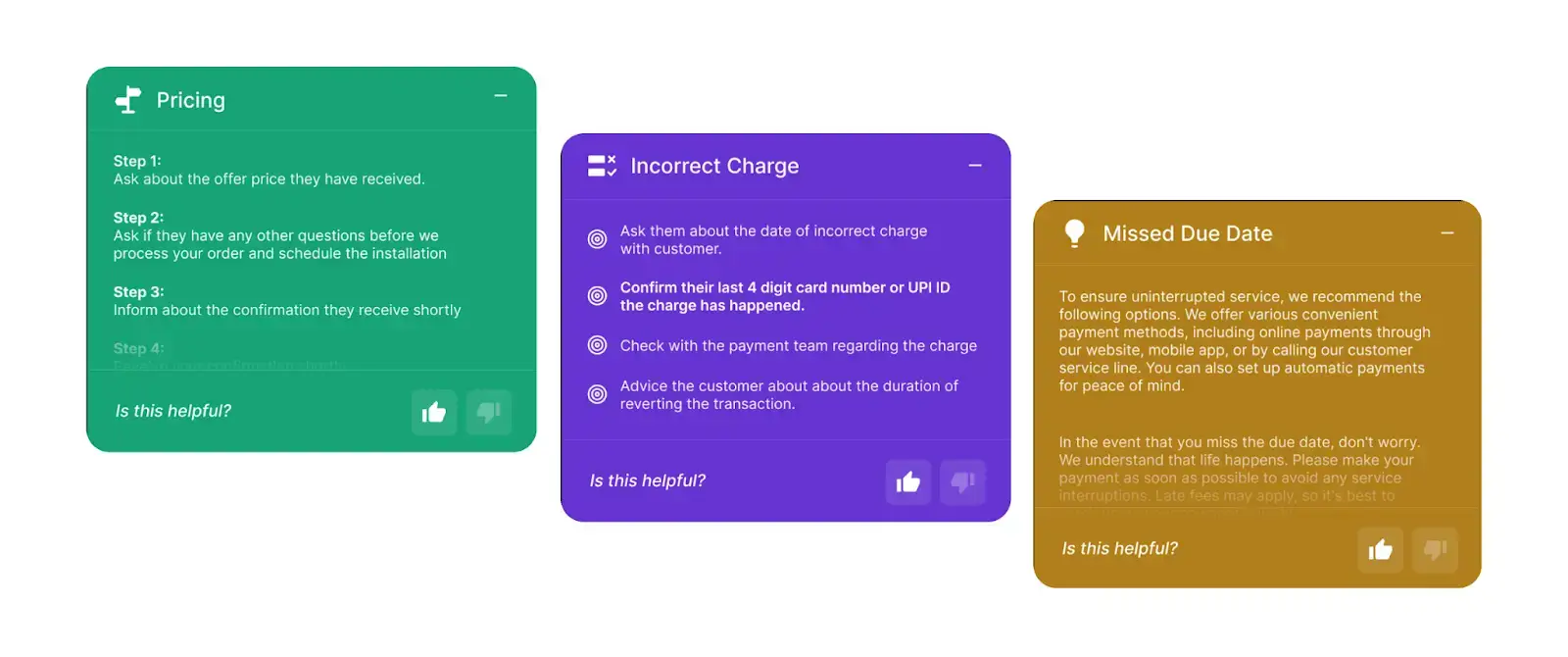
4. Real-Time Agent Assistance: Convin's Agent Assist feature uses machine learning to provide real-time guidance and suggestions during customer interactions, improving agent performance and reducing response times.
Benefits and ROI of Implementing Machine Learning Analytics
Implementing machine learning analytics in customer service offers numerous benefits and delivers a significant return on investment (ROI)
1. Enhanced Customer Satisfaction: Businesses can better understand and meet customer needs by leveraging sentiment analysis and predictive analytics, increasing satisfaction and loyalty.
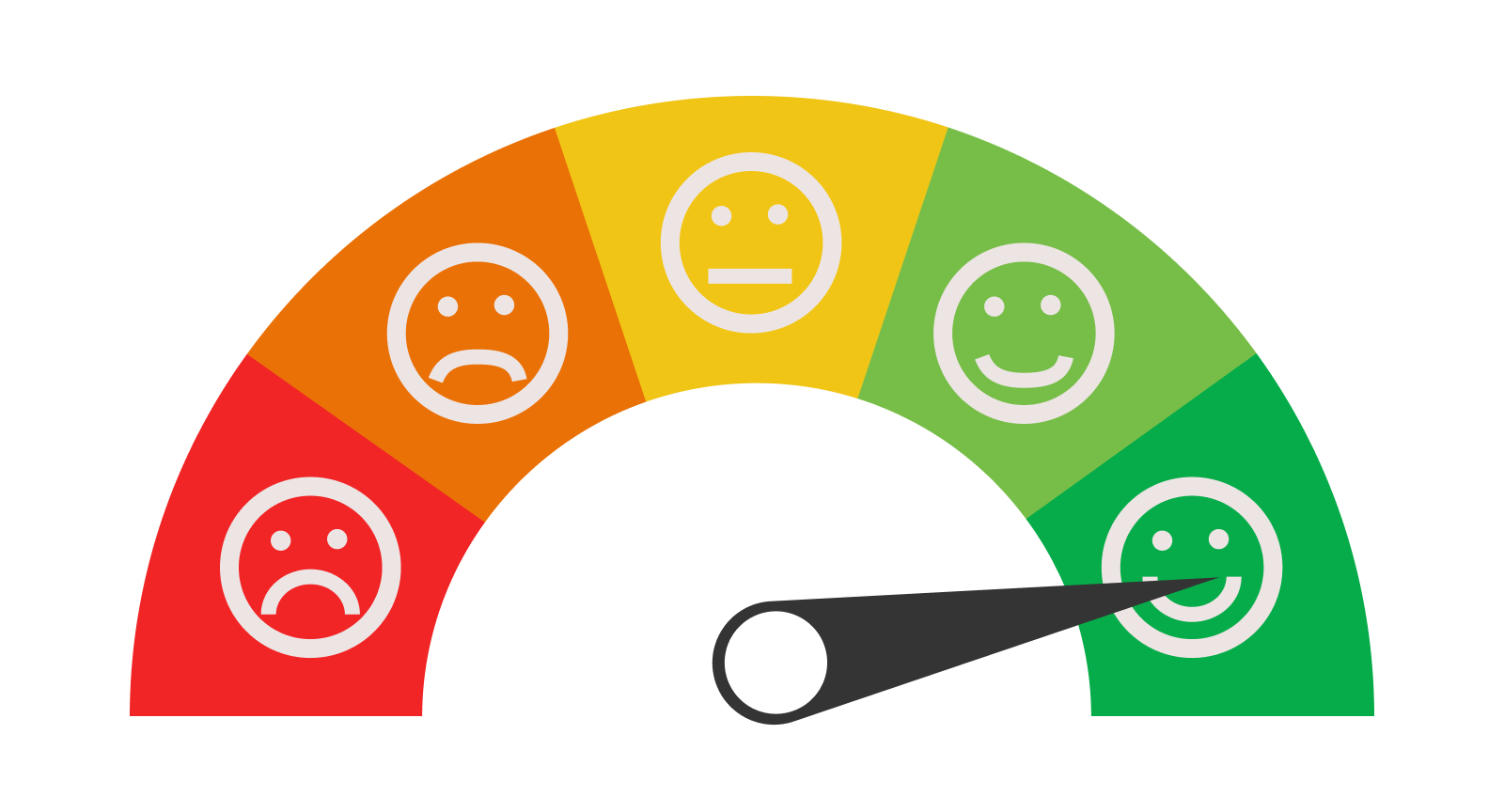
2. Operational Efficiency: Automating routine tasks through machine learning analytics tools frees customer service agents to handle more complex inquiries, improving overall efficiency and reducing operational costs.
3. Improved Decision-Making: Machine learning data analytics provides actionable insights that drive informed decision-making, helping businesses optimize customer service strategies and operations.
4. Increased Revenue: Personalized recommendations and proactive customer engagement driven by predictive analytics can boost sales and retention rates, contributing to higher revenue.
5. Real-Time Insights: Machine learning analytics enables real-time monitoring and analysis of customer interactions, allowing businesses to respond promptly and effectively to customer needs.
In conclusion, machine learning analytics is a powerful tool for transforming customer service. The applications are vast and impactful, from sentiment analysis and predictive analytics to real-time agent assistance.
How Convin Leverages Machine Learning Analytics to Transform Customer Service

Convin leverages machine learning analytics to revolutionize customer service by recording, transcribing, and analyzing customer interactions across various channels. Using advanced machine learning models, Convin provides deep insights into agent performance and customer sentiment, enabling personalized coaching and real-time assistance.
This results in improved agent efficiency, enhanced customer satisfaction, and proactive resolution of customer issues, ultimately driving higher customer retention and increased business revenue.
Companies like Convin are at the forefront of leveraging these technologies to deliver exceptional customer experiences and drive business success. For those looking to master these technologies, a machine learning analytics course can provide the necessary skills and knowledge to harness the full potential of these tools.
Applying Machine Learning Analytics to Revolutionize Customer Service
Machine learning analytics revolutionizes customer service by providing powerful tools and techniques to understand and predict customer behavior. By leveraging machine learning data analytics, businesses can enhance customer service operations, improving customer satisfaction, loyalty, and overall business performance.
Critical components of machine learning analytics, such as data collection, preprocessing, algorithm selection, and continuous improvement, ensure that businesses can extract valuable insights from their data.
Techniques like sentiment analysis, machine learning, and predictive analytics allow companies to gauge customer emotions and anticipate their needs, leading to proactive and personalized customer engagement.
FAQs
1. How is machine learning used in customer service?
Machine learning in customer service analyzes customer interactions, predicts behavior, automates routine tasks, and provides real-time support. It helps in sentiment analysis, personalizes customer experiences, and enhances agent performance through predictive analytics and automated coaching, improving customer satisfaction and operational efficiency.
2. What is machine learning analytics?
Machine learning analytics combines machine learning algorithms with data analysis techniques to extract meaningful insights from large datasets. It involves training models on historical data to identify patterns, predict future trends, and make data-driven decisions, enhancing various business processes, including customer service, marketing, and operations.
3. What is machine learning in CRM?
Machine learning in Customer Relationship Management (CRM) automates data analysis to enhance customer interactions. It predicts customer needs, personalizes marketing efforts, and identifies sales opportunities. Machine learning helps businesses build stronger relationships, improve customer satisfaction, and increase retention rates by analyzing customer behavior and preferences.
4. What is the role of machine learning in data analytics?
Machine learning enhances data analytics by automating the analysis process, identifying complex patterns, and making accurate predictions. It enables real-time data processing and decision-making, provides insights that traditional methods might miss, and continuously improves model accuracy through learning from new data, thus driving more informed business strategies.