
Machine learning contact centers have become a cornerstone in enhancing customer service experiences in the rapidly evolving digital landscape. By integrating machine learning(ML), businesses streamline their operations and deliver personalized and efficient solutions to their customers.
This blog post delves deep into the application of machine learning in customer service, particularly in call centers, exploring its mechanisms, benefits, and implementation strategies.
Knock Knock! Convin’s ML solutions are here to improve your customer service!
What is Machine Learning (ML)?
Machine learning (ML) is a subset of artificial intelligence (AI) that enables systems to learn and improve from experience without being explicitly programmed. It involves developing algorithms that can process and learn from data, making decisions or predictions based on that information. ML is a game-changer in customer service, offering innovative ways to enhance the customer experience.
Incorporating AI machine learning data science into contact center operations offers transformative potential, enabling more efficient, personalized, and proactive customer service. As the industry continues to evolve, leveraging ML's capabilities will be vital to staying competitive and meeting customers' ever-changing expectations.
How is Machine Learning Used in Customer Service?
Machine learning (ML) is revolutionizing customer service, particularly in contact centers, by enhancing efficiency, personalization, and predictive capabilities.
Using insights from Convin's application in this field, let's dive deeper into how ML transforms customer service.
1. Automated Customer Interactions
a. Chatbots and Virtual Assistants
- Learning and Adaptation: ML-powered chatbots and virtual assistants continuously improve by learning from each interaction and adapting their responses based on what has been successful in past conversations.
- Handling Complex Queries: They can handle a broader range of queries over time, understanding context and nuances in customer requests.
- Customer Behavior Insights: These tools gain insights into customer preferences and behaviors by analyzing interaction data, further refining their response mechanisms.
b. Real-Time Assistance with Convin's Agent Assist
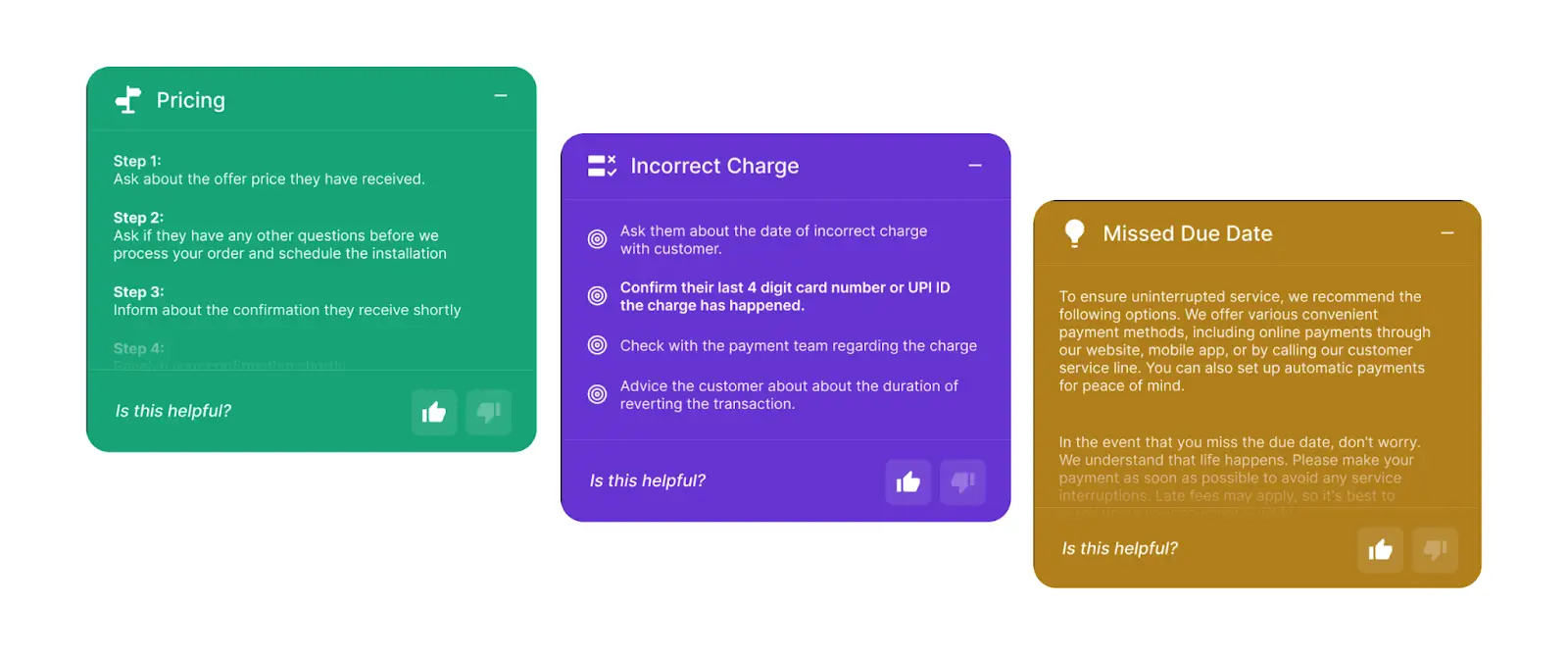
- Real-Time Guidance: Convin's Agent Assist provides instant, real-time suggestions to agents during customer interactions, ensuring that agents swiftly make the most appropriate and effective decisions.
- Guided Script and Proactive Alerts: It offers a guided script and sends proactive alerts to agents, assisting them in navigating complex customer interactions and preventing potential issues before they escalate.
- Dynamic Battlecards: Agent Assist employs dynamic battlecards that offer context-sensitive prompts, guiding agents through intricate scenarios and improving issue resolution.
c. Continuous Learning
- Data-Driven Improvements: These AI-driven systems continually evolve by analyzing new interaction data, which helps refine their algorithms and enhance their effectiveness in dealing with customer queries.
- Performance Optimization: The continuous learning process allows for optimizing responses and strategies based on real-time analysis, ensuring that customer service is constantly improving.
- Knowledge Base Integration: With integrated knowledge bases, like ConvinGPT, agents have access to a wealth of information in real-time, aiding in providing accurate and effective responses to customer queries.
These detailed aspects of automated customer interactions highlight the depth and sophistication of machine learning applications in enhancing customer service, making interactions more efficient, personalized, and contextually relevant.
2. Personalization in Customer Service through Machine Learning
Machine learning significantly enhances personalization in customer service, offering a more tailored and engaging experience for customers.
a. Data-Driven Insights
- Comprehensive Customer Profiles: ML algorithms sift through vast datasets to create detailed customer profiles, capturing preferences, past interactions, and behavior patterns.
- Service Tailoring: These insights enable businesses to customize their services, aligning offerings with individual customer expectations and needs.
- Dynamic Adaptation: ML systems dynamically adapt to changing customer data, ensuring that personalization strategies evolve with customer preferences, enhancing relevance and effectiveness over time.
b. Behavior Prediction
- Predictive Modeling: ML employs predictive modeling to anticipate customer needs and preferences based on historical data and real-time interactions.
- Anticipatory Service: This foresight allows businesses to proactively offer solutions, recommendations, or information that aligns with the customer's current context or upcoming needs, enhancing satisfaction and engagement.
- Enhanced Engagement: Predictive insights enable businesses to engage customers at opportune moments, with relevant offers or information, thereby increasing engagement and the likelihood of positive responses.
c. Contextual Understanding

- Sentiment and Intent Analysis: ML algorithms can analyze textual or spoken communication to understand customer sentiment and intent, providing a nuanced context for personalization.
- Customized Interactions: This contextual understanding allows customer service agents or automated systems to tailor their communication style, recommendations, and solutions to fit the customer's mood and intent, fostering a more empathetic and effective interaction.
d. Continuous Learning and Improvement
- Feedback Loops: Machine Learning(ML) systems incorporate feedback from customer interactions to refine personalization algorithms continuously, ensuring that the personalization becomes more accurate and effective over time.
- A/B Testing: Machine learning can also facilitate A/B testing of different personalization strategies, allowing businesses to identify the most effective approaches and continuously optimize their customer interactions.
Use Case: Convin's Automated Quality Management and Coaching
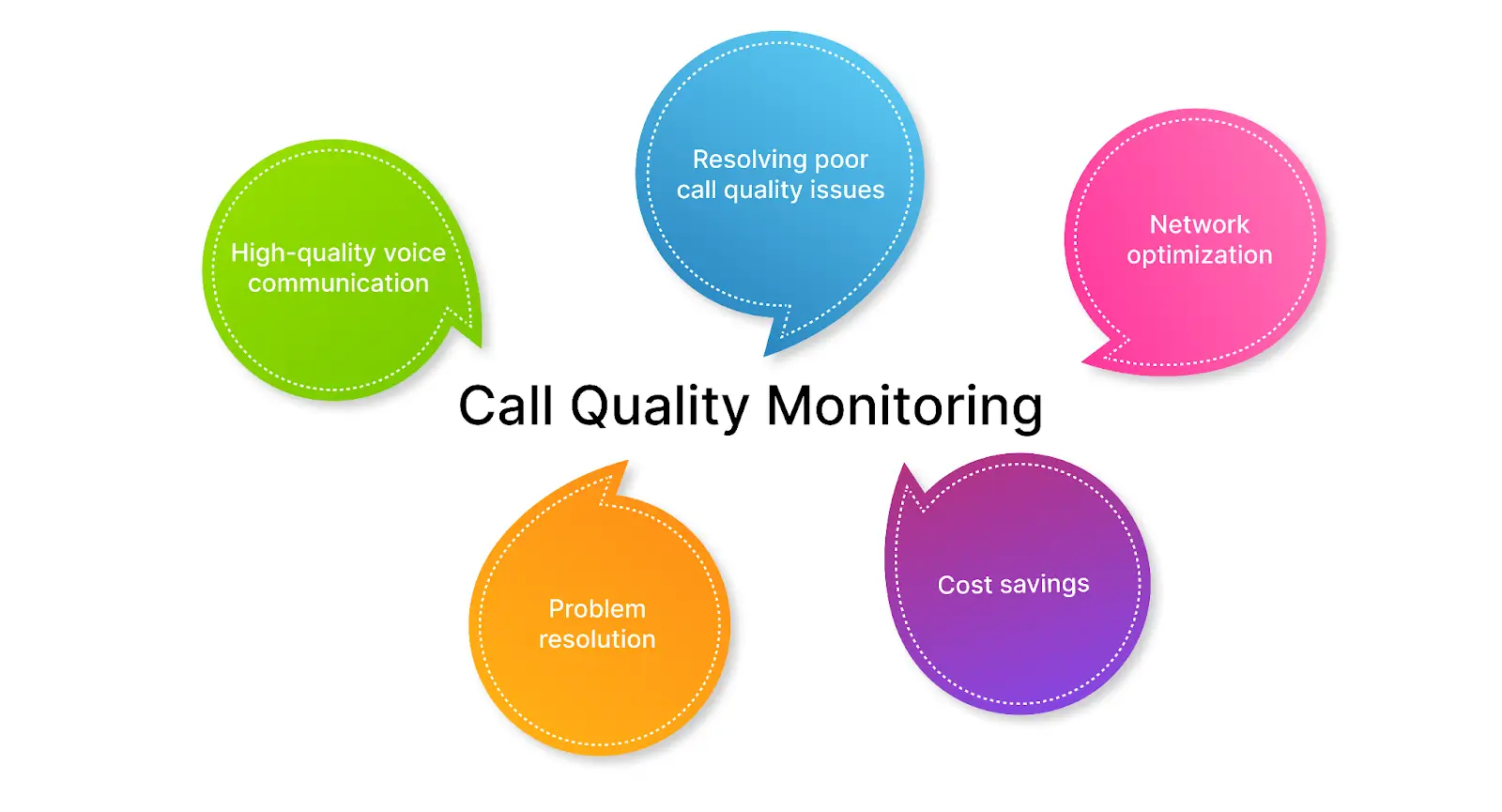
- Automated Quality Management: Convin's tools analyze 100% of customer interactions across various channels, using custom auditing templates to identify performance blockers and customer dissatisfaction points.
- Tailored Coaching: Based on the insights gathered, Convin's system identifies coaching opportunities, allowing personalized agent training. This addresses the individual development needs of each agent and directly impacts customer satisfaction by improving interaction quality.
- Proactive Measures: Utilizing customer intelligence and custom-tracking, Convin can identify parameters that drive positive and negative outcomes. This proactive approach allows businesses to tailor their strategies and interactions based on predicted customer behaviors, significantly enhancing the personalization aspect of customer service.
This use case illustrates how machine learning facilitates a nuanced understanding of customer interactions, enabling a more personalized and predictive approach to customer service. By leveraging these insights, businesses can meet and exceed customer expectations, fostering loyalty and driving business success.
3. Predictive Analytics: In-Depth Use Case with Convin's Application
Predictive analytics in customer service, particularly through machine learning, offers a robust framework for understanding and preempting customer needs and behaviors.
Convin's implementation of predictive analytics provides a tangible example of these capabilities in action.
a. Behavior Forecasting
- Convin's system utilizes machine learning to analyze historical interaction data, allowing it to forecast customer behavior and service requirements.
- By identifying trends and patterns, Convin can predict when a customer is likely to contact support, the nature of the inquiry, and the optimal resolution strategy, enhancing proactive customer service.
b. Enhanced Decision-Making
- The insights derived from predictive analytics enable Convin to make informed decisions about resource allocation, agent training, and customer engagement strategies.
- For example, if predictive analytics indicate a spike in call volume, Convin can allocate additional agents in advance to manage the influx, ensuring that customer service levels are maintained.
Practical Use Case
a. Predictive Customer Segmentation
By analyzing past customer interactions, Convin can segment customers based on their behavior and preferences. This segmentation allows for tailored communication and services, improving customer satisfaction and loyalty.
b. Proactive Service
Utilizing predictive analytics, Convin can anticipate common issues before they escalate. For instance, if the data suggests a recurring product issue, Convin can proactively reach out to customers likely to be affected, offering solutions or workarounds, thereby reducing the volume of incoming support requests.
c. Optimized Agent Training
Predictive analytics can identify which topics or issues are becoming more prevalent in customer interactions. This insight allows Convin to adjust its training programs, ensuring that agents are well-equipped to handle these emerging trends.
d. Customer Experience Improvement
By understanding the patterns and predicting future customer behavior, Convin can refine its interaction strategies to offer more personalized and timely support, significantly enhancing the overall customer experience.
Through these use cases, it's evident how predictive analytics, powered by machine learning, plays a pivotal role in transforming customer service, offering a proactive, data-driven approach that significantly benefits both the service provider and the customers.
4. Sentiment Analysis Use Case
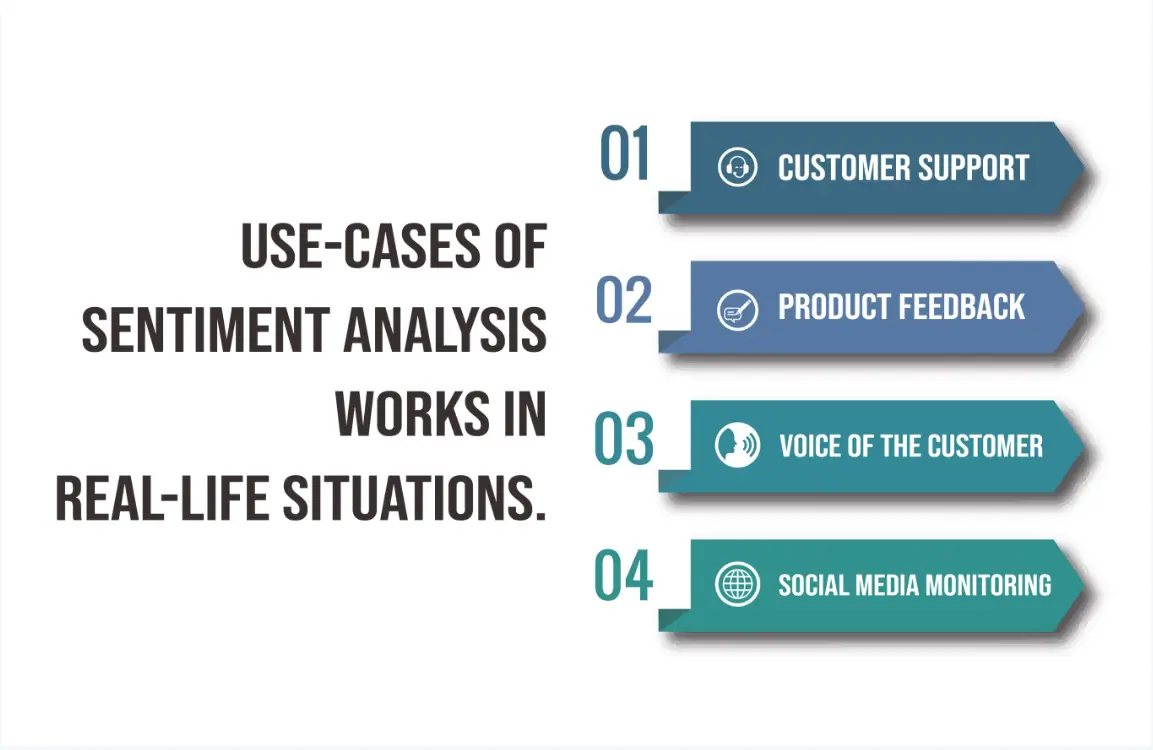
Let's explore a detailed use case of sentiment analysis in customer service, particularly how Convin utilizes this technology to enhance contact center operations.
a. Emotion Detection and Analysis
- Convin's machine learning algorithms are adept at analyzing various customer interactions, including calls, chats, and emails.
- These algorithms assess the tone, word choice, and context to determine the customer's emotional state: satisfaction, frustration, or neutrality.
Download Our Free Empathy Statements Checklist for Authentic Customer Engagement!
b. Real-Time Feedback for Agents
- During live interactions, Convin's Agent Assist uses sentiment analysis to provide real-time feedback to agents.
- If a conversation takes an adverse turn, the system can alert the agent and suggest strategies to steer the conversation back to a positive outcome, ensuring immediate corrective actions are taken.
c. Automated Quality Management
- Convin's automated quality management system uses sentiment analysis to review customer interactions across all channels.
- This system identifies blockers in agent performance and unhappy customer conversations, enabling managers to address these issues proactively.
d. Data-Driven Coaching and Improvement
- Sentiment analysis results pinpoint areas where agents can improve, guiding personalized coaching sessions.
- Managers can develop targeted training programs to enhance customer interaction skills by understanding common sentiment trends.
e. Enhancing Customer Experience and Satisfaction
- Convin helps businesses better understand their customers by monitoring and analyzing sentiment in real-time, leading to improved customer experiences.
- Positive sentiment trends can highlight successful strategies or agent behaviors, which can be replicated or reinforced across the team.
f. Strategic Business Insights
- Sentiment analysis provides valuable insights beyond individual interactions, offering a broader view of customer satisfaction and service quality over time.
- These insights can inform strategic decisions, helping businesses to refine their service offerings, adjust their communication strategies, and improve overall customer satisfaction.
By integrating sentiment analysis into their customer service framework, Convin significantly enhances the ability of contact centers to respond to customer emotions effectively, tailor interactions to individual needs, and continuously improve service quality based on real-time feedback and comprehensive data analysis.
Results first, payment later.
Machine Learning in Call Center: An In-Depth Analysis
1. Advanced Analytics and Machine Learning
a. Data-driven decision-making: Advanced analytics machine learning enable call centers to make informed decisions by analyzing historical and real-time data.
Example: A financial services firm uses ML to categorize calls based on urgency and topic, ensuring high-priority issues are addressed first, thus improving customer satisfaction and operational efficiency.
b. Agent Performance Optimization: ML helps identify patterns that indicate successful interactions by analyzing call data, allowing managers to tailor coaching and training programs.
Example: A healthcare provider utilizes ML to assess agent performance metrics against customer feedback, identifying areas where agents can improve communication skills or product knowledge.
2. AI Machine Learning Models
a. Customer Inquiry Prediction: ML models can predict the nature of customer inquiries, allowing for better resource allocation and faster response times.
Example: An airline employs ML models to predict common inquiries during weather disruptions, enabling proactive communication and efficient issue resolution.
b. Automated Response Generation: These models can automate responses to common queries, reducing wait times and freeing up agents for more complex issues.
Example: A utility company uses AI machine learning models to automatically respond to routine billing questions, enhancing efficiency and customer satisfaction.
3. Use Case Examples
a. Telecom Call Pattern Analysis
A telecom company analyzes call patterns using ML to identify peak times, common issues, and caller sentiment, optimizing staffing and improving issue resolution strategies.
b. E-commerce Chatbot Interaction
An e-commerce platform utilizes chatbots, powered by ML, to handle routine product inquiries and order status questions, improving response times and customer engagement.
c. Financial Services Customer Segmentation
A bank integrates ML to segment customers based on their interaction history and product usage, enabling personalized service and targeted product offers.
d. Healthcare Provider Sentiment Analysis
A healthcare provider uses ML for sentiment analysis on patient calls, identifying dissatisfaction trends and areas for service improvement.
By leveraging machine learning, call centers and customer service departments can transform their operations, offering personalized, efficient, and proactive services that significantly enhance the customer experience.
How to Implement Call Center Machine Learning?
Implementing machine learning (ML) in call centers involves a series of structured steps to enhance customer service experiences through automation, analysis, and intelligent assistance. Here's an in-depth look at how to effectively integrate ML into call center operations, illustrated with use cases and examples.
1. Data Collection and Analysis
- Gather Comprehensive Data: Collect various types of data, such as call transcripts, customer feedback, and interaction outcomes, to provide a rich dataset for ML models.
- Perform Descriptive Analytics: Use statistical analysis to understand past performance and identify trends and patterns in call center operations.
- Identify Improvement Areas: Analyze historical data to pinpoint common issues or bottlenecks in customer service processes that ML could address.
- Use Case: A retail company analyzes call data to identify recurring customer complaints about product availability. By understanding these patterns, the company can improve inventory management and reduce related customer service inquiries.
2. Choosing the Right ML Model
- Evaluate Business Needs: Determine the specific objectives, such as reducing response times or improving customer satisfaction scores.
- Select Model Types: Choose from various ML models, like supervised learning for predictive analytics or unsupervised learning for clustering customer queries.
- Customize Models: Tailor ML models to address the unique challenges and goals of the call center.
- Use Case: An insurance firm selects a supervised learning model to predict customer inquiry types based on historical data, enabling better call routing and quicker resolutions.
3. Training and Testing
- Training: Use historical data to train the ML model, ensuring it learns from past interactions to make accurate predictions or decisions.
- Validation: Implement cross-validation techniques to assess the model's performance on unseen data.
- Testing: Evaluate the model's effectiveness in a controlled environment to ensure it meets the desired outcomes.
- Use Case: A telecom company trains an ML model to predict customer churn based on interaction history and tests its accuracy in identifying at-risk customers.
4. Integration
- System Compatibility: Ensure the ML model integrates seamlessly with existing call center software and databases.
- Workflow Integration: Incorporate ML insights into the call center's operational workflows, such as routing calls based on predicted inquiry complexity.
- User Training: Train staff on using and benefiting from ML-enhanced systems, emphasizing how ML outputs aid decision-making and efficiency.
- Use Case: A bank integrates an ML model into its call center system to analyze customer sentiment in real-time, guiding agents on handling calls empathetically.
5. Monitoring and Optimization
- Continuous Monitoring: Regularly assess the ML model's performance and impact on call center metrics like average handle time (AHT) and customer satisfaction (CSAT).
- Feedback Loops: Establish mechanisms for feedback on the model's outputs, enabling ongoing learning and adaptation.
- Iterative Improvement: Use monitoring insights to refine and enhance the ML model, ensuring it evolves with changing customer service needs.
- Use Case: An e-commerce platform continuously monitors its chatbot's performance, using customer interactions to optimize its responses and improve engagement rates.
By following these steps, call centers can harness the power of machine learning to transform their customer service operations, making them more responsive, efficient, and attuned to customer needs.
Revolutionizing Customer Service: The Power of Machine Learning!
Integrating machine learning (ML) in customer service, particularly within call centers, signifies a monumental shift in how businesses engage with their clientele. This technological advancement isn't just about automating processes; it's about transforming the core of customer service into a more efficient, personalized, and predictive experience.
Machine learning streamlines call center operations by automating routine tasks, enabling agents to focus on more complex customer needs. ML algorithms can predict call volumes, allocate resources effectively, and even route calls to the most suitable agent, thereby reducing wait times and improving overall service efficiency.
The synergy of machine learning and Convin's innovative solutions is setting a new benchmark in customer service. By embracing this technology, businesses can not only meet their customers' evolving expectations but also stay ahead in the competitive landscape, ensuring long-term success and customer loyalty.
Don't just meet expectations; exceed them! Book your demo with Convin today and step into the future of customer service excellence!
FAQs
1. What is ML in a call center?
ML in a call center refers to using machine learning algorithms to analyze data, predict outcomes, and optimize interactions, enhancing efficiency and customer experience.
2. How is AI used in call centers?
AI in call centers automates responses, guides agents in real time, analyzes customer sentiment, and improves operational efficiency through intelligent insights.
3. What is the use of machine learning in the hospitality industry?
In the hospitality industry, machine learning is used to personalize guest experiences, optimize pricing, forecast demand, and improve operational efficiency.
4. How is machine learning used in the telecom industry?
In the telecom industry, machine learning is applied to predict network failures, optimize service delivery, personalize customer offerings, and enhance predictive maintenance.