In today's digital age, call centers play a pivotal role in enhancing customer satisfaction and building strong relationships between organizations and their customers. However, understanding and managing customer sentiments during interactions has become increasingly challenging due to the sheer volume of communication. This is where Call Center Text Sentiment Analytics comes into play, offering a powerful solution to decode customer emotions and optimize call center operations.
In this blog post, we will delve deep into what Call Center Text Sentiment Analytics is, how it works, its importance, and its impact on call centers at various levels.
Make your Business a success with Convin
What is Call Center Text Sentiment Analytics?

Call Center Text Sentiment Analytics, often referred to simply as sentiment analysis, is a sophisticated Natural Language Processing (NLP) technique used to understand and interpret the emotional tone, opinions, and attitudes expressed within text data generated in a call center environment.
This technology is particularly valuable for businesses and organizations that interact with customers through various communication channels, such as phone calls, emails, chat messages, social media, and surveys. It enables them to gain actionable insights from this textual data, ultimately leading to improvements in customer service, operational efficiency, and decision-making processes.
Suggestive Read: Best Practices for Sentiment Analysis
Understanding Sentiment Analysis!
Sentiment analysis is a subfield of NLP that focuses on determining the sentiment or emotional context within a piece of text. It involves analyzing words, phrases, and expressions to categorize the sentiment into one or more of the following categories:
- Positive: Indicates a favorable sentiment, satisfaction, or happiness.
- Negative: This signifies dissatisfaction, frustration, or anger.
- Neutral: Represents a lack of emotional tone or sentiment.
Sentiment analysis can be fine-tuned to recognize more nuanced emotions or even specific emotions like joy, sadness, fear, or surprise. The primary goal is to gauge the emotional tone of text data accurately.
After understanding what sentiment analysis is exactly, let’s shift our focus to how call center sentiment analysis in business works.
How Call Center Text Sentiment Analytics Works?
Sentiment analysis methods involve several steps to understand and classify text data into different sentiment categories.
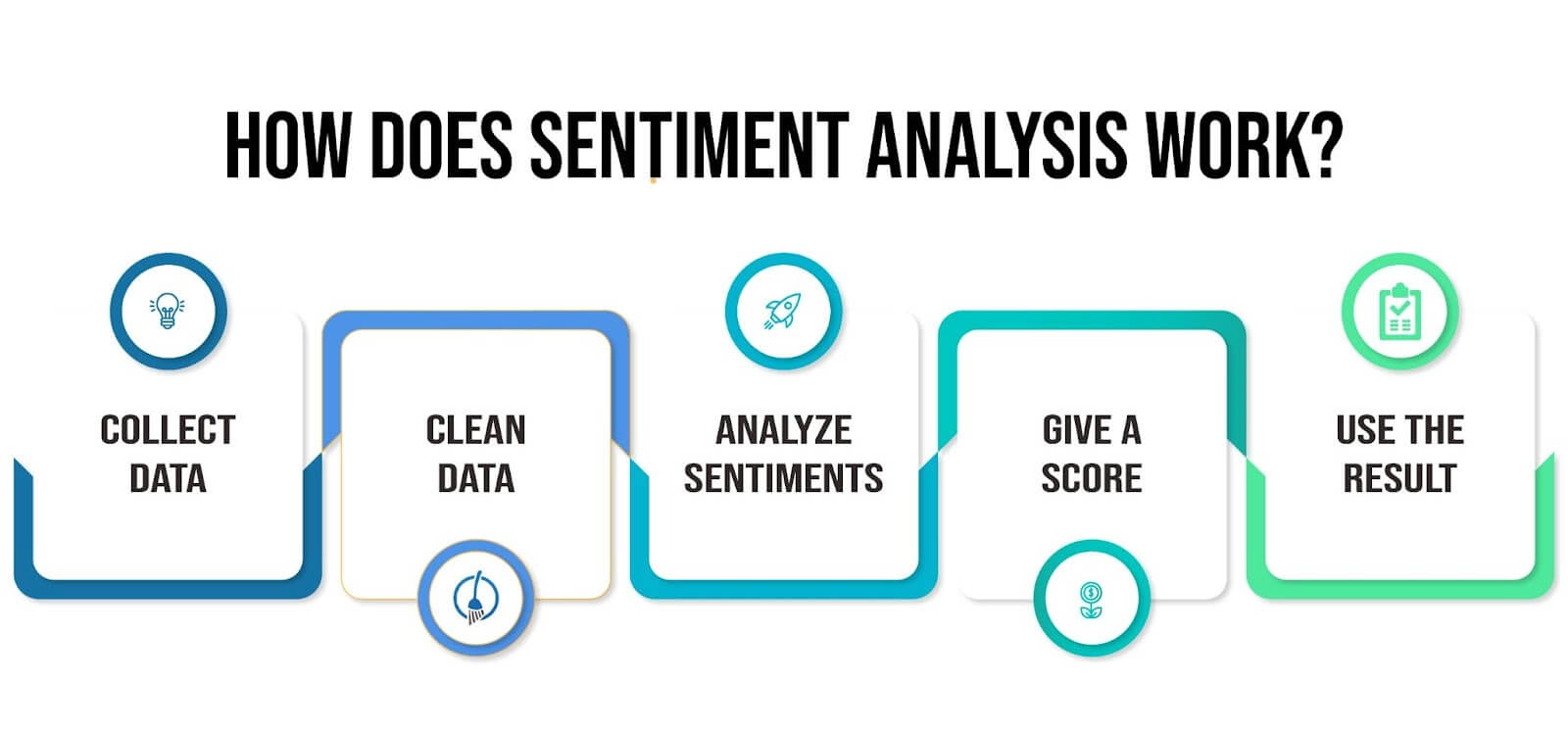
Here's a simplified breakdown of how it works.
1. Data Collection: The first step in implementing sentiment analysis in a call center is to gather the textual data generated during customer interactions. This data can come from various sources, including transcripts of phone conversations, chat logs, emails, social media comments, and customer surveys.
2. Text Preprocessing: Raw text data is often noisy and contains irrelevant information. Preprocessing involves cleaning and structuring the data by removing special characters, punctuation, numbers, and stopwords (common words like "the," "and," "is") to make it more suitable for analysis.
3. Tokenization: The text is then divided into smaller units called tokens, usually words or phrases. Tokenization helps the sentiment analysis model break down the text into digestible parts for analysis.
4. Sentiment Classification: At the core of sentiment analysis is a machine learning model or algorithm that classifies each token, sentence, or document into a sentiment category (positive, negative, or neutral). This classification is based on the words used, their context, and the overall structure of the text.
5. Sentiment Scoring: Beyond simple categorization, sentiment analysis often assigns a sentiment score to each piece of text. These scores can range from -1 (very negative) to +1 (very positive), with 0 representing neutrality. This scoring provides a more granular view of sentiment intensity.
6. Aggregation and Analysis: Sentiment analysis tools and sentiment analysis software aggregate sentiment scores across various interactions, time periods, or channels. This aggregated data can be analyzed to identify trends, patterns, and anomalies in customer sentiment.
7. Reporting and Visualization: The results of sentiment analysis are typically presented in the form of reports, dashboards, or visualizations that make it easy for call center managers and decision-makers to understand the sentiment trends and take appropriate actions.
Now that we've explored how Call Center Text Sentiment Analytics works, let's dive deeper into the importance of this technology and its profound impact on call centers. Understanding the mechanics of sentiment analysis is crucial, but recognizing why it matters can shed light on the transformative potential it holds for customer service and operational excellence.
This blog is just the start.
Unlock the power of Convin’s AI with a live demo.

Importance of Call Center Text Sentiment Analytics
In today's fast-paced and highly competitive business landscape, understanding and meeting customer expectations is paramount. Call Center Text Sentiment Analytics has emerged as a game-changing technology that empowers organizations to not only listen to their customers but also decipher the intricate nuances of their emotions and opinions.
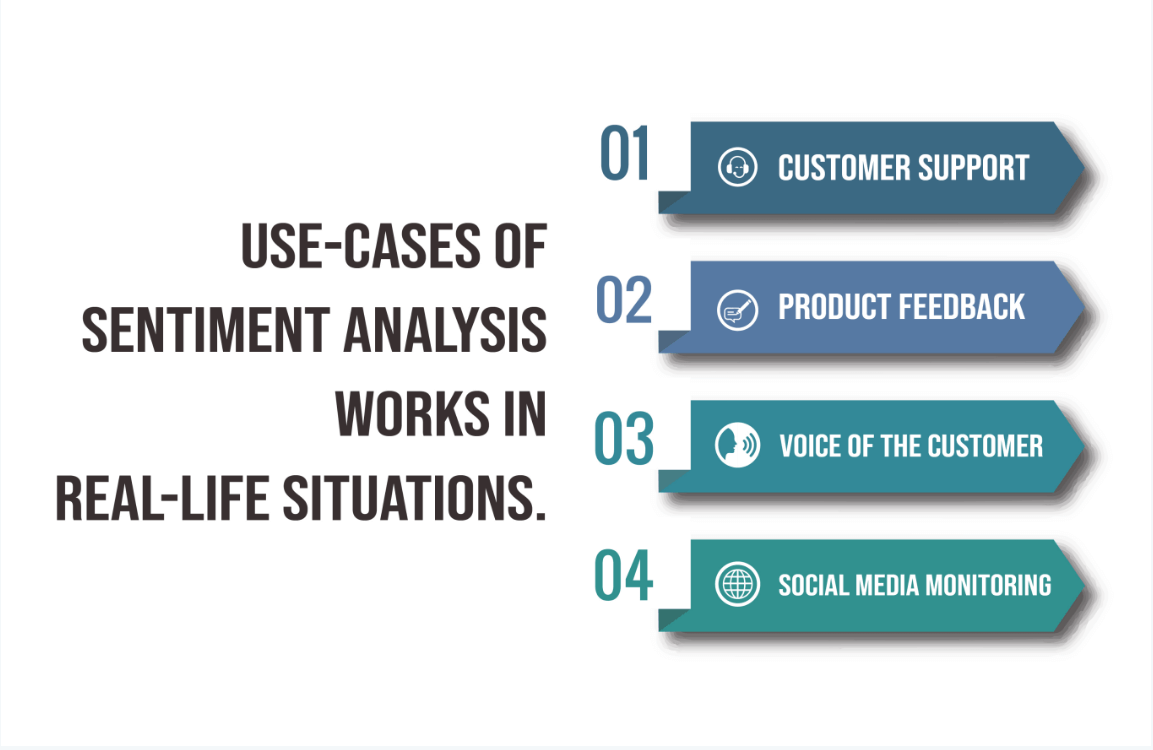
Here are 4 important factors of call center text sentiment analyses
1. Enhancing Customer Experience
Call Center Text Sentiment Analytics empowers organizations to understand customer sentiments in real-time. By identifying and addressing customer issues promptly, businesses can enhance the overall customer experience and build stronger relationships.
2. Agent Performance Improvement
At the agent level, sentiment analysis can provide valuable feedback and coaching opportunities. Agents can receive insights into customer satisfaction, allowing them to adjust their communication and problem-solving skills accordingly.
3. Organizational Decision-Making
Sentiment analysis provides organizations with actionable insights to improve processes, products, and services. By identifying recurring issues and trends, businesses can make informed decisions to drive operational excellence.
4. Proactive Issue Resolution
By detecting negative sentiments early, call centers can proactively address customer issues before they escalate. This can help reduce customer churn and maintain customer satisfaction.
Now that we've established the crucial role of Call Center Text Sentiment Analytics. Let's shift our focus to its tangible impact on call centers, exploring how sentiment analysis can make a significant difference at the agent level, customer level, and organizational level. Understanding the importance of this technology sets the stage for comprehending the transformative influence it can wield in the world of customer service and support.
Impact of Text Sentiment Analysis on Call Centers
The impact of Text Sentiment Analysis on Call Centers extends far beyond the boundaries of technology—it reaches deep into the heart of customer interactions and operational excellence. At the agent, organizational, and customer levels, the transformative power of sentiment analysis is evident in the way it shapes customer experiences, empowers agents, and drives strategic decision-making.
1. Agent Level
- Agents can receive real-time feedback on their interactions, allowing them to adjust their approach to meet customer expectations.
- Sentiment analysis can be used to route specific customer inquiries to agents with the right skill set to handle them effectively.
- Agents can identify opportunities for upselling or cross-selling based on positive sentiments expressed by customers.
2. Organizational Level
- Call centers can optimize resource allocation and staffing based on call volume and sentiment trends.
- Organizations can identify training needs and improve agent performance through targeted coaching.
- Sentiment analysis can help in developing and refining marketing strategies based on customer feedback and market sentiment.
3. Customer Level
- Customers benefit from faster issue resolution and improved service quality.
- Businesses can tailor their communication to better align with customer preferences and sentiments.
- Customers are more likely to remain loyal to brands that actively address their concerns and feedback.
Transitioning from understanding its effects on agents, organizations, and customers, we will now explore the critical steps required to integrate sentiment analysis into call center operations successfully. These steps are pivotal in harnessing the full potential of sentiment analysis and reaping its benefits for your call center and the overall customer experience.
How to Successfully Implement Text Sentiment Analysis?
Implementing Text Sentiment Analysis is not merely a technological endeavor; it's a strategic initiative that can revolutionize the way organizations engage with their customers and manage their operations.
Implementing sentiment analysis in a call center involves the following key steps:
1. Selecting the Right Tools
The success of any sentiment analysis implementation begins with choosing the right tools or sentiment analysis software that aligns with your organization's specific needs and objectives.
Here are some key considerations
- Feature Set: Evaluate the features offered by sentiment analysis tools. Look for capabilities such as sentiment scoring, text preprocessing, real-time analysis, and integration with your existing systems.
- Scalability: Consider the scalability of the tool. Ensure it can handle the volume of text data generated by your call center, especially during peak periods.
- Accuracy: Assess the accuracy of the sentiment analysis model. Look for tools that offer high precision and recall rates in sentiment classification.
- Customization: Check if the tool allows you to customize the sentiment analysis model to recognize industry-specific terminology, slang, or unique customer expressions.
- Cost: Evaluate the cost of the tool, including licensing fees, maintenance, and support. Ensure it fits within your budget.
2. Data Integration
Once you've selected the right sentiment analysis tool, the next step is to integrate it with your call center's data sources. This involves the following:
- Data Sources Identification: Identify the sources of textual data that you want to analyze. This can include call recordings, chat logs, email transcripts, social media interactions, and customer surveys.
- Data Collection: Extract and collect the relevant textual data from these sources. Ensure that the data is stored in a structured format that can be easily processed by the sentiment analysis tool.
- Data Cleaning: Before integration, it's essential to clean and preprocess the data. Remove noise, such as special characters, punctuation, and irrelevant information, to ensure the data is in a consistent format for analysis.
- Integration Process: Work with your IT department or technical team to integrate the sentiment analysis tool with your data sources. This may involve setting up APIs, connectors, or batch processes to feed data into the tool.
3. Training and Customization
To make sentiment analysis effective in your call center, it's crucial to train and customize the sentiment analysis model to understand the unique characteristics of your industry, customer base, and communication style:
- Data Annotation: Create a labeled dataset with examples of text data categorized into sentiment classes (positive, negative, neutral). These annotated samples are used to train the model.
- Training the Model: Utilize machine learning techniques to train the sentiment analysis model on your annotated dataset. The model learns to recognize patterns and sentiment indicators specific to your domain.
- Fine-tuning: Continuously fine-tune the model as new data becomes available. This helps the model adapt to changing customer sentiments and evolving industry terminology.
- Industry-Specific Knowledge: Incorporate domain-specific knowledge into the model. For example, if your call center deals with healthcare queries, ensure the model understands medical terminology and context.
4. Feedback Loop
The implementation process doesn't end with integration and training. It's essential to establish a feedback loop for ongoing improvement:
- Continuous Monitoring: Regularly monitor the performance of the sentiment analysis system. Identify areas where it may misclassify sentiment or miss important nuances.
- Feedback Collection: Gather feedback from call center agents and supervisors regarding the accuracy of sentiment analysis results. This feedback can be invaluable in making improvements.
- Model Updates: Based on feedback and performance metrics, update and retrain the sentiment analysis model as necessary. This ensures that it remains accurate and relevant over time.
- Benchmarking: Compare the results of sentiment analysis with customer feedback and satisfaction metrics to gauge its effectiveness in enhancing the customer experience.
By following these steps meticulously, organizations can ensure a successful implementation of Text Sentiment Analysis in their call centers. This technology, when integrated effectively, can lead to better customer service, enhanced agent performance, and data-driven decision-making, ultimately driving business success.
Let Convin Take the First Step for You!
Convin offers products that can assist in text sentiment analysis by providing tools and solutions to understand and analyze customer sentiments within text data. Here are some key ways in which Convin products can help with text sentiment analysis:
1. Text Analytics Dashboard: Convin provides a user-friendly dashboard that allows you to visualize sentiment analysis results. It offers easy-to-understand visualizations and reports, making it convenient to track sentiment trends and patterns over time.
2. Real-time Sentiment Analysis: Convin's products offer real-time sentiment analysis capabilities, allowing you to monitor and respond to customer sentiments as they happen. This is crucial for addressing issues promptly and providing immediate feedback.
3. Omni-Channel Support: Convin's products are designed to handle text data from various channels, including call recordings, chat logs, emails, and social media interactions. This comprehensive approach enables you to capture sentiment across all customer touchpoints.
4. Natural Language Processing (NLP): Convin leverages NLP techniques to enhance the accuracy of sentiment analysis. This enables the system to understand the context, sarcasm, and nuances within text data.
By leveraging Convin's products, organizations can streamline their text sentiment analysis processes, gain deeper insights into customer sentiments, and take proactive steps to improve customer service, agent performance, and overall business outcomes.
Its advanced AI capabilities, including natural language understanding, sentiment analysis, and personalized interactions, make it an invaluable tool for enhancing customer relationships and delivering exceptional service.
Empathy is the practice of understanding people - their feelings as well as their perspectives - and it's an essential tool for a healthy relationship.
Are you curious? Get on a quick call to know more!
FAQ
1. What is text sentiment analysis?
Text Sentiment Analysis is the process of using natural language processing (NLP) techniques to determine the emotional tone or sentiment expressed in a piece of text, such as positive, negative, or neutral.
2. How is the sentiment of a text measured?
The sentiment of a text is typically measured by assigning a sentiment score or label based on the words, phrases, and context within the text. Common sentiment scoring ranges from -1 (very negative) to +1 (very positive), with 0 indicating neutrality.
3. How do you quantify sentiment analysis?
Sentiment analysis is quantified by analyzing a collection of texts and calculating aggregated sentiment scores, sentiment distribution, or sentiment percentages to understand the overall sentiment trends within the data.
4. What is a good sentiment analysis score?
A good sentiment analysis score depends on the context and industry, but generally, a score close to +1 indicates a positive sentiment, close to -1 signifies a negative sentiment, and around 0 represents neutrality.
5. What is an example of sentiment analysis?
Example of sentiment analysis: Analyzing customer reviews of a product to determine if they express positive, negative, or neutral opinions about it.
6. What are good sentiment analysis models?
Good sentiment analysis models include BERT, GPT-3, VADER, and LSTM-based models. These models offer high accuracy and can capture complex sentiment nuances in text data.