In the dynamic environment of call and contact centers, understanding customer emotions and sentiments is crucial for delivering exceptional service. Sentiment analysis, a powerful tool in the realm of customer interaction, plays a pivotal role in achieving this understanding.Â
This blog post explains what is sentiment analysis and the best ways to utilize it in call centers, highlighting the role of advanced tools like Convin.ai in enhancing the customer experience.
Explore sentiment analysis to decipher emotion for valuable customer insightsÂ
Introduction to Sentiment Analysis in Contact Center
Sentiment analysis involves evaluating the communication, whether spoken or written, to determine the emotional tone behind it. In call centers, this means analyzing customer interactions to gauge their satisfaction, frustration, or any other emotional state.
- Text Sentiment Analysis
Text sentiment analysis focuses on written communications like emails, chat messages, and social media posts. It helps in understanding customer sentiments expressed through text.
- Sentiment Analysis in Calls
Sentiment analysis in voice calls goes beyond words, analyzing tone, pitch, and speech patterns to capture the customer's emotional state.
Application of Sentiment Analysis
Sentiment analysis is used in various ways across different industries to understand and analyze emotions and opinions expressed in text or speech.Â
Here are some key applications:
- Customer Feedback Analysis: Businesses use sentiment analysis to evaluate customer reviews, surveys, and feedback, helping them understand customer satisfaction and preferences.
- Social Media Monitoring: Companies monitor social media platforms to gauge public sentiment about their brand, products, or services and to respond to customer concerns proactively.
- Market Research: Sentiment analysis is used in market research to analyze consumer attitudes and trends, which can inform marketing strategies and product development.
- Brand Reputation Management: By analyzing sentiments expressed online, and businesses can manage and improve their brand reputation, addressing negative sentiments and leveraging positive ones.
- Customer Service: In call centers, sentiment analysis helps in assessing the emotional tone of customer interactions, enabling better service and response strategies.
- Content Analysis: The media and publishing industries use sentiment analysis to understand the emotional tone of content and its potential impact on audiences.
- Financial Markets: Sentiment analysis is used to gauge market sentiment, analyzing news, social media, and financial reports to make informed investment decisions.
Overall, sentiment analysis is a powerful tool for businesses and organizations to extract meaningful insights from vast amounts of unstructured data, helping them make data-driven decisions and improve customer experiences.
This blog is just the start.
Unlock the power of Convin’s AI with a live demo.

Process of Sentiment Analysis
Sentiment analysis works by using natural language processing (NLP), text analysis, and computational linguistics to identify and extract subjective information from source materials.Â
Here's a basic overview of how it functions:
- Data Collection: The process begins with collecting relevant data, which could be text from social media posts, customer reviews, survey responses, or transcripts of spoken conversations.
- Preprocessing the Data: The collected data is then preprocessed. This step may include removing noise and irrelevant information, standardizing text, and correcting spelling errors to prepare the data for analysis.
- Natural Language Processing (NLP): NLP techniques are applied to understand the context and nuances of the language used in the text. This involves parsing sentences, identifying parts of speech, and understanding the structure and meaning of sentences.
- Sentiment Identification: The core of sentiment analysis involves identifying the sentiments expressed in the text. This is typically done by analyzing the words and phrases used and their context. Sentiments are generally categorized as positive, negative, or neutral.
- Use of Machine Learning: Many sentiment analysis tools use machine learning algorithms. These algorithms are trained on large datasets to recognize patterns and nuances in language that indicate sentiment.
- Sentiment Scoring: The text is often given a sentiment score, which quantifies the sentiment on a scale (e.g., -1 to 1, where -1 is very negative, 0 is neutral, and 1 is very positive).
- Contextual Analysis: Advanced sentiment analysis tools also consider the context in which words are used, as the same word can have different sentiments in different contexts.
- Output and Interpretation: The final step is the output, where the analyzed data is presented in a format that allows users to understand the sentiment trends in the text. This can be in the form of scores, visualizations, or summary reports.
Sentiment analysis tools are continually evolving, becoming more sophisticated in understanding and interpreting human language and emotions.

Accuracy of Sentiment Analysis Tools
The accuracy of sentiment analysis can vary based on several factors, and while it has improved significantly with advancements in technology, there are still limitations to consider:
- Quality of the Algorithms: The accuracy largely depends on the quality of the natural language processing (NLP) algorithms and machine learning models used. More advanced models trained on larger, more diverse datasets are more accurate.
- Context and Nuance: Sentiment analysis can struggle with understanding context, sarcasm, irony, and subtle nuances of language. Misinterpreting these elements can lead to inaccuracies.
- Language and Cultural Variations: Idioms unique to a language and variations in articulation across cultures can have an impact on accuracy. Models trained predominantly on data from one language or culture may not perform as well on others.
- Domain-Specific Language: Sentiment analysis tools can be less accurate in industries with specialized jargon or technical language unless they are specifically trained for those domains.
- Data Quality: The quality and representativeness of the input data also impact accuracy. Poorly structured, noisy, or biased data can lead to less reliable results.
- Continuous Learning and Updates: Accuracy improves when systems are regularly updated and retrained to adapt to new linguistic patterns and changes in language use.
In general, while sentiment analysis provides valuable insights, its results should be interpreted as part of a broader analysis, considering potential sources of error and the context of the data.
Benefits and Limitations of Sentiment Analysis in Contact Centers
Sentiment analysis in contact centers offers several benefits but also comes with certain limitations.
Benefits:
- Enhanced Customer Understanding: Sentiment analysis provides deeper insights into customer emotions and attitudes, helping contact centers understand how customers feel about their products or services.
- Improved Service Quality: By analyzing customer sentiments, contact centers can identify areas needing improvement, leading to enhanced service quality and customer satisfaction.
- Agent Performance Evaluation: Sentiment analysis can help evaluate and improve agent performance by providing feedback on how well they handle customer interactions.
- Proactive Issue Resolution: Analyzing sentiments in real-time allows contact centers to identify and address potential issues before they escalate, improving the customer experience.
- Data-Driven Decision Making: Sentiment analysis provides quantitative data on customer feedback, supporting more informed and strategic decision-making.
Limitations:
- Contextual Understanding: Sentiment analysis tools may struggle with understanding context, sarcasm, and nuances in language, leading to a potential misinterpretation of customer sentiments.
- Cultural and Linguistic Variations: Different cultures and languages express sentiments in various ways, which can be challenging for sentiment analysis tools to capture accurately.
- Dependence on Data Quality: The accuracy of sentiment analysis is heavily reliant on the quality of the data. Poorly structured or biased data can lead to inaccurate analysis.
- Technical Limitations: The complexity of natural language processing and machine learning models means that sentiment analysis tools may require significant resources and expertise to implement effectively.
- Privacy and Ethical Concerns: Recording and analyzing customer interactions raises privacy concerns. It's crucial to ensure compliance with data protection laws and ethical standards.
In a simple context, while sentiment analysis in contact centers can provide valuable insights and aid in improving customer service, it's important to be aware of its limitations and use it as part of a broader strategy for customer experience management.

Implementing Sentiment Analysis in Contact Centers
Implementing sentiment analysis in contact centers involves integrating AI-driven tools to analyze customer interactions across multiple channels, enabling real-time identification of emotions and thereby enhancing service quality and customer experiences through proactive insights and tailored responses.
1. Utilize Sentiment Analysis Tools
Leverage advanced sentiment analysis tools and software to analyze customer interactions automatically. These tools can process large volumes of data, providing real-time insights.
2. Incorporate into Training
Use sentiment analysis data to train customer service representatives. Understanding customer emotions can guide agents in handling calls more empathetically and effectively.
3. Enhance Customer Experience
Apply insights from sentiment analysis to tailor customer interactions, address pain points, and improve the overall customer experience.
4. Monitor Agent Performance
Sentiment analysis can also be used to evaluate and improve agent performance, ensuring they respond appropriately to customer emotions.
5. Predictive Analysis
Use sentiment analysis to predict potential customer issues or trends, allowing proactive measures to enhance service quality.
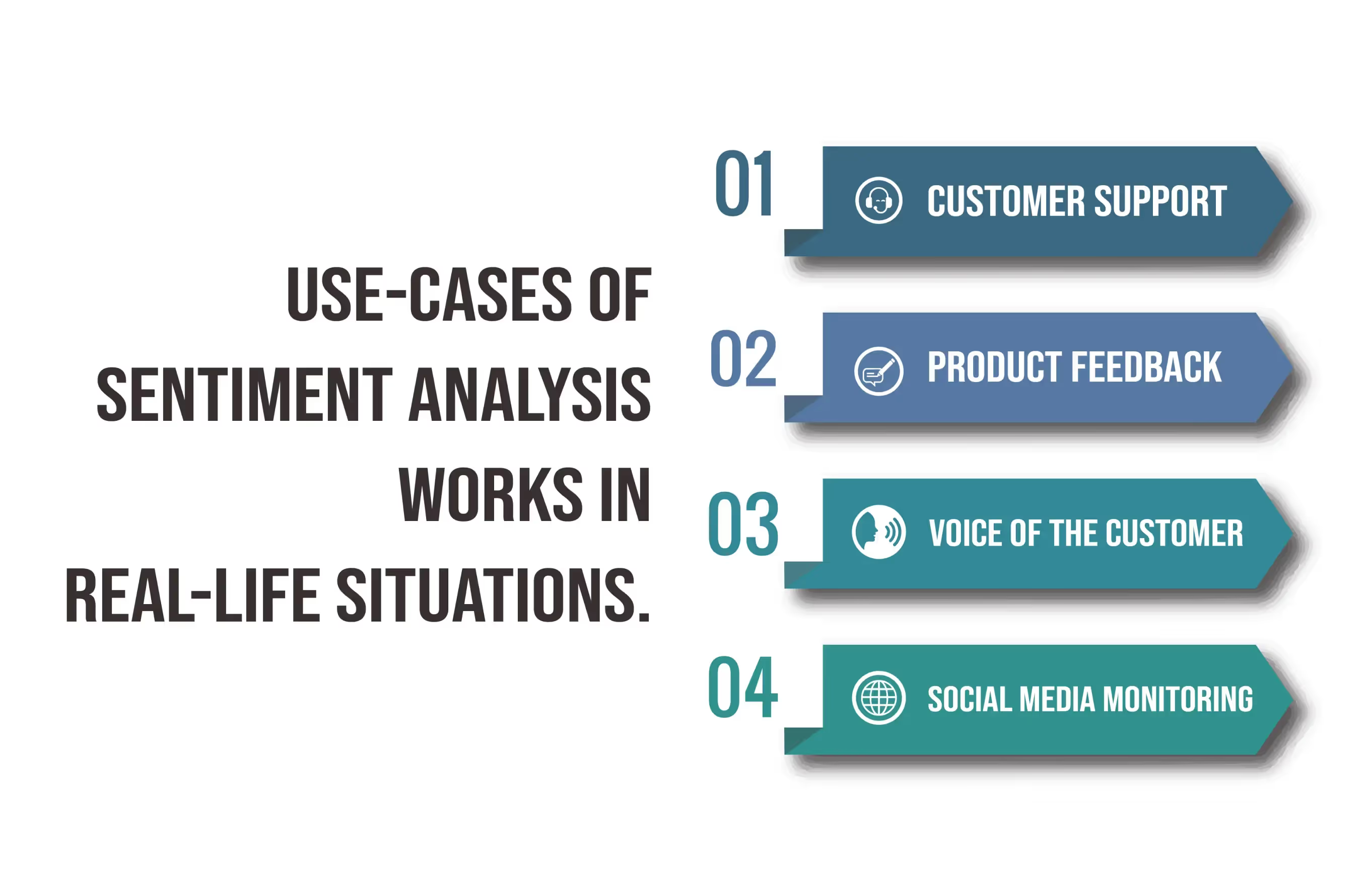
A Perpetual Solution for Sentiment Analysis
Convin emerges as a robust solution in this context, offering sophisticated sentiment analysis capabilities tailored for call and contact centers.
Role of Convin in Sentiment Analysis
- Real-Time Analysis: Convin provides real-time sentiment analysis, offering immediate insights into customer emotions during interactions.
- Comprehensive Data Set: It utilizes a vast sentiment analysis data set, ensuring an accurate and nuanced understanding of customer sentiments.
- Actionable Insights: Convin not only analyzes sentiments but also provides actionable insights, helping call centers improve their service strategies.
- Integration with Existing Systems: It seamlessly integrates with existing call center systems, enhancing the value of sentiment analysis without disrupting current workflows.
To conclude, sentiment analysis is an indispensable resource for contemporary call and contact centers.Â
By leveraging advanced solutions like Convin, businesses can gain a deeper understanding of their customers, leading to improved service, enhanced customer satisfaction, and, ultimately, a stronger brand reputation.Â
Embrace the power of sentiment analysis with Convin and transform your call center into a hub of customer empathy and satisfaction. Walk through the demo today.
FAQs
1. How can I improve my sentiment in a call center?
Train agents in empathy and active listening, use sentiment analysis tools for real-time feedback and consistently act on customer feedback to improve sentiment.
2. What is the best way to do sentiment analysis?
The best way is to use advanced sentiment analysis tools that utilize natural language processing and machine learning to interpret and analyze emotions in text or speech accurately.
3. Which approach is best for sentiment analysis?
A combination of machine learning algorithms for large-scale analysis and human oversight for context and nuanced interpretation is often the most effective approach.
4. What is sentiment in call center?
In a call center, sentiment refers to the emotional tone and attitude of both customers and agents during interactions, which can significantly impact the customer experience and satisfaction.