
Two terms that frequently surface today are Generative AI and Large Language Models (LLMs). Both have transformed how businesses interact with data and users, offering innovative solutions to complex problems.
Call centers, the frontline of customer interaction for many businesses, stand to benefit significantly from these technologies. By integrating Generative AI and LLMs, call centers can enhance customer service, streamline operations, and improve efficiency.
This blog post will dissect the differences between Generative AI and LLMs, delve into their unique use cases and examples, and explore their impact on industries, particularly call centers.
Unlock Your Call Center's Future: Tap into the Transformative Power of AI – Click to Embark on the Journey!
Difference Between LLM and Generative AI in the Context of Call Center Enhancement!
Generative AI, a frontier in AI research, refers to algorithms that can generate new content, be it text, images, or even code, based on the data they have been trained on. This technology can transform customer service by creating personalized and dynamic interactions, automating responses, and providing predictive insights.
On the other hand, Large Language Models like GPT (Generative Pretrained Transformer) have made headlines for their ability to understand and generate human-like text, opening new avenues for enhancing customer service through chatbots, automated support, and real-time interaction analysis.
Generative AI and LLMs, while both under the AI umbrella, serve distinct purposes and operate differently. Below is a comparative analysis presented in a tabular format.

Understanding the nuances between Generative AI and Large Language Models (LLMs) is crucial for businesses and developers aiming to leverage AI technologies effectively. By recognizing their distinct roles, capabilities, and applications, organizations can make informed decisions on how best to integrate these advanced AI systems into their operations, ensuring they harness the full potential of AI to drive innovation, enhance efficiency, and deliver exceptional customer experiences.
How are Generative AI and LLM helping call centers enhance customer service?
1. Automated Customer Support
LLMs and Generative AI are instrumental in developing sophisticated chatbots and virtual assistants to handle various customer inquiries. These AI-powered systems can understand and process natural language, providing accurate and relevant customer responses. This reduces the need for human intervention in standard queries, streamlining customer service operations and reducing wait times.
Examples and Use Cases
- Software Industry: AI chatbots can assist users in troubleshooting common software issues, providing step-by-step guidance without human intervention.
- Healthcare: Virtual assistants can offer preliminary support, schedule appointments, and provide general health advice, enhancing patient engagement and support.
- Edtech: Chatbots can offer students personalized learning resources, answer FAQs, and provide administrative support, like enrollment guidance and course information.
- Customer Service: Across industries, AI-powered systems can handle routine customer queries, allowing human agents to focus on more complex issues, increasing efficiency and customer satisfaction.
- Fintech: Virtual assistants can guide users through financial services, like account setup, transaction inquiries, and basic financial advice, ensuring round-the-clock customer support.
2. Personalization
LLMs can analyze extensive customer interaction data to understand preferences and history, enabling them to offer highly personalized responses. Generative AI can take this further by creating customized content, such as personalized recommendations, responses, or even marketing messages, enhancing the customer experience.
Examples and Use Cases
- Software: Tailored support and product recommendations based on the user's interaction history and preferences.
- Healthcare: Personalized patient engagement, including follow-up messages, tailored health advice, and personalized treatment information.
- Edtech: Customized learning pathways and resources based on the student's progress, interests, and performance.
- Fintech: Personalized financial advice, product recommendations, and services based on the customer's financial behavior and goals.
3. Efficiency
By automating routine and repetitive tasks, generative AI and LLMs allow human agents to concentrate on complex and nuanced customer interactions. This speeds up response times and enhances customer service quality, as agents can devote more time and attention to individual cases.
Examples and Use Cases
Automated handling of standard queries and issues, like password resets, order tracking, and basic troubleshooting, across various sectors, significantly reducing the workload on human agents.
4. Training
Generative AI can create realistic and diverse customer interaction scenarios for training purposes. These scenarios help agents practice their skills in a controlled environment, improving their problem-solving abilities and preparing them for various customer interactions.
Examples and Use Cases
- Software: Simulation of software troubleshooting scenarios to train agents on complex problem-solving.
- Healthcare: Creating patient interaction scenarios for training healthcare providers in communication, empathy, and problem-solving in patient care.
- Edtech: Developing scenarios to train customer service agents in handling various student inquiries, from technical issues to course guidance.
- Fintech: Simulating financial inquiries and scenarios to train agents in providing accurate and practical financial advice and support.
Through these applications, generative AI and LLMs significantly enhance the efficiency, personalization, and overall quality of customer service in call centers across various industries.
Results first, payment later
This blog is just the start.
Unlock the power of Convin’s AI with a live demo.

How do you Implement Generative AI and LLM Successfully in Call Centers?
A detailed and strategic approach is required to successfully implement Generative AI and Large Language Models (LLMs) in call centers.
1. Identify Objectives
- Define clear, measurable goals for AI integration, such as reducing average handling time (AHT), enhancing customer satisfaction scores, or improving resolution rates.
- For example, in healthcare, the objective might be to reduce patient wait times and provide accurate, instant responses to common inquiries.
2. Data Preparation
- Gather and clean historical interaction data, ensuring it's representative and free of biases.
- In the software industry, this could involve compiling a diverse range of bug reports and customer feedback to train models that can assist in troubleshooting.
3. Choose the Right Model
- Select models based on the specific needs of your industry. For instance, GPT-3 is ideal for customer service chatbots, while a different generative model is better for creating promotional content.
- In fintech, choosing a model that can understand financial jargon and accurately respond to queries.
4. Integration
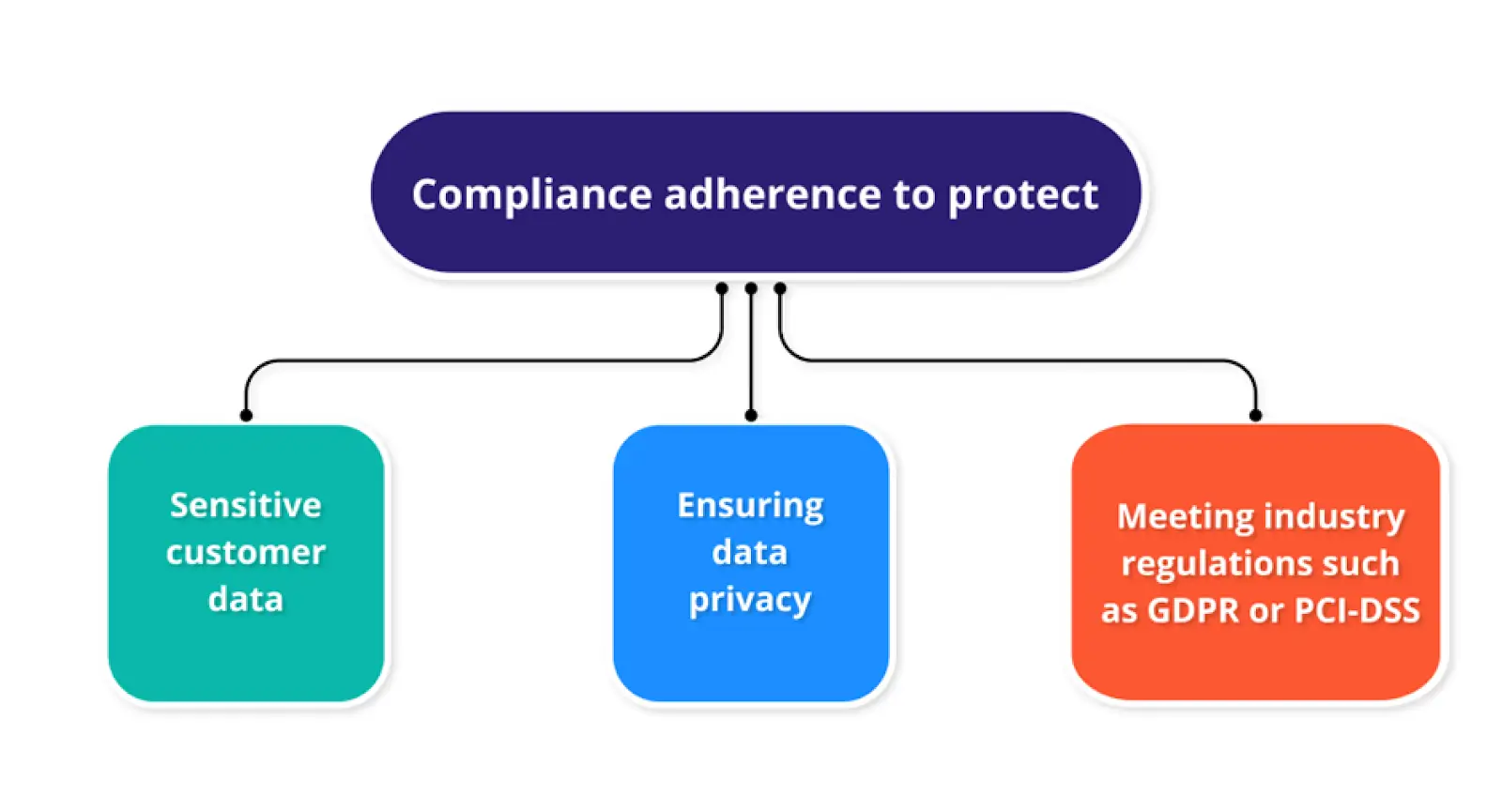
- Integrate AI systems in a way that enhances the capabilities of human agents. For example, in edtech, AI could provide real-time suggestions to tutors on student queries.
- Ensure the integration follows best practices in generative AI security, protecting customer data, and ensuring the AI's outputs are reliable and safe.
5. Training and Testing
- Train your AI models on the prepared datasets, then test them extensively to ensure they meet your objectives and perform well in real-world scenarios.
- In customer service, this could involve simulating various customer interactions to refine the AI's response accuracy and relevance.
6. Monitoring and Updating
- Monitor AI performance to ensure it adapts to new data and evolving industry trends. Regular updates help maintain effectiveness and security.
- This might mean updating the AI to understand new medical terminologies or procedures in healthcare.
Examples and Use Cases
- Software Industry: Implementing generative AI for automated code generation, bug detection, and resolution suggestions can enhance developer productivity. LLMs can be used to improve documentation and support chatbots.
- Healthcare: AI can analyze patient inquiries to provide instant, accurate information, reducing the burden on healthcare professionals and improving patient experience.
- EdTech: AI can generate personalized learning content or provide real-time student assistance, enhancing the learning experience.
- Customer Service: Across industries, AI can handle routine inquiries, allowing human agents to focus on more complex issues, thereby improving overall efficiency and customer satisfaction.
- FinTech: AI can assist in interpreting financial queries, providing investment advice, detecting fraudulent activities, and enhancing security and customer trust.
By focusing on these steps and adapting the approach to specific industry needs, call centers can leverage the power of Generative AI and LLMs to significantly enhance their efficiency, customer satisfaction, and operational outcomes.
How Convin Harnesses Generative AI and LLMs for Superior Customer Support?
Convin is leveraging Generative AI and LLMs across its suite of products to enhance customer service significantly in various ways.
Here's an in-depth look at how these technologies are integrated and the benefits they bring to customer interactions:
1. Natural Language Understanding (NLU)
Convin uses Generative AI to power its NLU capabilities, enabling the system to comprehend customer queries and interactions in a human-like manner. This understanding allows for more accurate responses and better customer service, as the system can interpret the intent and context of customer communications.
2. Sentiment Analysis

Through Generative AI, Convin's sentiment analysis tool can interpret and analyze the emotional tone behind customer conversations. This insight helps customer service agents understand their mood and tailor their responses to improve engagement and satisfaction, enhancing the overall customer experience.
3. Knowledge Base
Convin's knowledge base is enriched with Generative AI, which helps in automatically updating and expanding the repository of information. This ensures that customer service agents have access to the most relevant and up-to-date information, enabling them to provide quick and accurate responses to customer inquiries.
4. Call Summary
The platform utilizes Generative AI to generate concise and informative summaries of customer calls. This feature helps agents and managers quickly grasp the key points of each interaction, facilitating better follow-up, training, and quality assurance processes.
5. AI Feedback
AI Feedback in Convin leverages Generative AI to provide real-time suggestions and insights to agents during or after customer interactions. This feedback can include tips on improving communication skills, technical knowledge, or customer engagement strategies, thereby directly contributing to enhancing the quality of customer service.
By combining the predictive power of Generative AI with the interpretative capabilities of LLMs, Convin offers a holistic approach to improving customer service. This dual integration ensures that every customer interaction is informed, efficient, and tailored to the individual’s needs, significantly boosting customer satisfaction and loyalty.
In essence, Convin’s integration of Generative AI and LLMs is a game-changer in the realm of customer service, offering a suite of tools that not only enhance the efficiency and effectiveness of agents but also elevate the overall customer experience.
Discover the Future of Customer Service: Experience firsthand how Convin's cutting-edge use of LLM and Generative AI can revolutionize your call center's efficiency and customer satisfaction.
Click here to schedule your demo and transform your customer service today!
FAQs
1. Are LLM and generative AI the same?
No, LLMs are a subset of generative AI focusing specifically on generating human-like text, while generative AI encompasses a broader range of content creation capabilities across various media.
2. What is the difference between GPT and LLM?
GPT (Generative Pretrained Transformer) is a type of LLM developed by OpenAI, designed to generate text. LLM (Large Language Model) refers to any large-scale model capable of understanding and generating human language.
3. What is the difference between LLM and traditional AI?
Traditional AI often focuses on rule-based or specific task-oriented processes. At the same time, LLMs leverage vast amounts of data to generate and understand human-like text, offering more flexible and generalized applications.
4. What is the difference between LLM and AI agent?
An AI agent is a system capable of autonomous actions in an environment to achieve designated goals, while an LLM is specifically designed to understand and generate human language.
5. Is a chatbot an LLM?
An LLM can power a chatbot if it uses a large language model to understand and generate human-like responses; however, not all chatbots use LLMs, as some operate on simpler, rule-based systems.
6. What does LLM mean in artificial intelligence?
In artificial intelligence, LLM stands for Large Language Model, a type of deep learning model trained on vast datasets to understand, interpret, and generate human language.