
In today's digital era, where customer experience is paramount, deep learning and generative AI are revolutionizing how businesses interact with and understand their customers. These technologies are not just buzzwords but integral tools reshaping the landscape of customer service, marketing, and overall experience.
Let's dive into the world of deep learning and generative AI, unravel their roles in customer experience, and explore how they're transforming this domain.
Discover how Convin utilizes Deep Learning to revolutionize customer experiences in Its innovative products!
Deep Learning and Generative AI: An In-Depth Exploration?
Deep Learning and Generative AI are two of the most groundbreaking advancements in artificial intelligence (AI) and machine learning (ML). They have profound applications in various sectors, especially in enhancing customer experiences. Let's delve deeper into these concepts, using specific examples and relating them to key areas like predictive analytics, personalization, conversational AI, and more.
1. Deep Learning
Deep Learning is a sophisticated subset of machine learning that uses neural networks with many layers (hence 'deep') to process data, make decisions, and to analyze various data factors. It excels in recognizing patterns, processing natural language, and even visual identification tasks.
a. Predictive Analytics
Deep learning algorithms can use massive customer data to predict future behaviors and trends. For example, an online retailer could use deep learning to predict which products a customer will likely buy next based on their browsing and purchase history.
b. Personalization
By understanding customer preferences and behaviors, deep learning enables the creation of highly personalized experiences. A streaming service could use deep learning to personalize movie recommendations for each user.
c. NLP and Language Modeling
Deep learning powers natural language processing to understand and interpret human language, making interactions with AI more natural. Language models like GPT (Generative Pre-trained Transformer) can generate human-like text, enabling better customer service chatbots.
d. Customer Feedback Analysis
Deep learning can analyze unstructured data like customer feedback or reviews to extract insights about customer satisfaction and preferences, aiding in improving products or services.
2. Generative AI
Generative AI refers to AI algorithms that can generate new, original content after learning from existing datasets.
a. Conversational AI
Generative AI can create chatbots that respond to inquiries and engage in more natural, varied conversations with customers, enhancing the customer support experience.
b. Speech Recognition and Voice Analytics
Generative AI is instrumental in advancing speech recognition technologies. In call centers, it can be used for real-time speech transcription, sentiment analysis, and even to provide suggestions to customer service representatives.
c. Language Modeling
Beyond text generation, generative AI can also create realistic voice and speech patterns, improving the human likeness of virtual assistants and AI-driven customer service tools.
d. Call Center Analytics
Generative AI can simulate various customer scenarios, helping in training customer service agents. It can also generate automated responses or suggestions to agents based on ongoing customer interaction.
3. Examples and Use Case Scenarios
a. Deep Learning in e-Retail
- Personalized Recommendations: By analyzing customer data, such as purchase history, browsing patterns, and product preferences, deep learning models can predict which products a customer will likely buy next. This tailoring leads to a more personalized shopping experience.
- Use Case: An online fashion retailer uses deep learning to recommend clothing items. The system suggests sizes, styles, and accessories based on the customer's past purchases and items they've viewed, significantly boosting sales and customer engagement.
b. Generative AI in Healthtech
- Personalized Health Advice: Generative AI models can analyze a patient's medical history, genetic information, and lifestyle habits to generate customized health tips and treatment plans.
- Use Case: A telehealth platform implements generative AI to provide personalized health and wellness advice. After a patient enters their medical history and daily habits, the system generates a tailored health plan, including diet and exercise recommendations.
c. Deep Learning in Fintech
- Fraud Detection: Deep learning models can analyze vast amounts of transaction data to detect anomalous patterns that may indicate fraudulent activity. These models learn from historical fraud data to identify subtle signs of potential fraud in real-time.
- Use Case: A bank employs deep learning algorithms to monitor transactions. The system flags unusual activity, such as large transactions from a rarely used account, alerting the fraud team for immediate investigation, thereby reducing the risk of financial losses.
d. Generative AI in Entertainment
- Content Creation: Generative AI can analyze user preferences, viewing history, and popular trends to generate new entertainment content, such as music, videos, or script ideas.
- Use Case: A music streaming service uses generative AI to create custom playlists and generate new music tracks. The system analyzes the types of songs a user frequently listens to and composes new music aligning with those tastes.
e. Customer Service with Deep Learning and Generative AI
- Advanced Chatbots: Combining deep learning and generative AI, customer service chatbots can understand and respond to a wide range of customer queries with high accuracy. They can personalize responses and adapt to the customer's emotional tone, improving the overall experience.
- Use Case: An e-commerce platform integrates an AI-powered chatbot for customer service. This chatbot responds to queries about orders, shipping, and products and detects customers' frustrations or confusion, adapting its responses to be more empathetic or detailed, leading to higher customer satisfaction and loyalty.
In each of these scenarios, applying deep learning and generative AI significantly enhances the efficiency, personalization, and effectiveness of services, directly translating to improved customer experiences and operational excellence.
As these technologies evolve, their impact on the customer experience space is bound to deepen further.
How is Deep Learning Transforming the Customer Experience Space?
Deep learning, a cutting-edge machine learning (ML) branch, significantly transforms the customer experience. Here's an in-depth look at how this technology is applied across various domains, enhancing customer interactions and driving business growth.
1. Predictive Analytics
- Concept: Deep learning models analyze vast customer data to identify patterns and predict future behaviors or trends.
- Application: E-commerce sites leverage these predictions to stock items likely in demand, reducing overheads and increasing sales.
- Example: Netflix uses predictive analytics to recommend movies and TV shows based on a user's viewing history.
2. Personalization
- Concept: Deep learning algorithms can personalize experiences by learning from customer data.
- Application: Online platforms suggest products, content, or services tailored to users' preferences and behaviors.
- Example: Spotify’s Discover Weekly playlist uses deep learning to curate a personalized playlist for each user.
3. Conversational AI and Speech Recognition
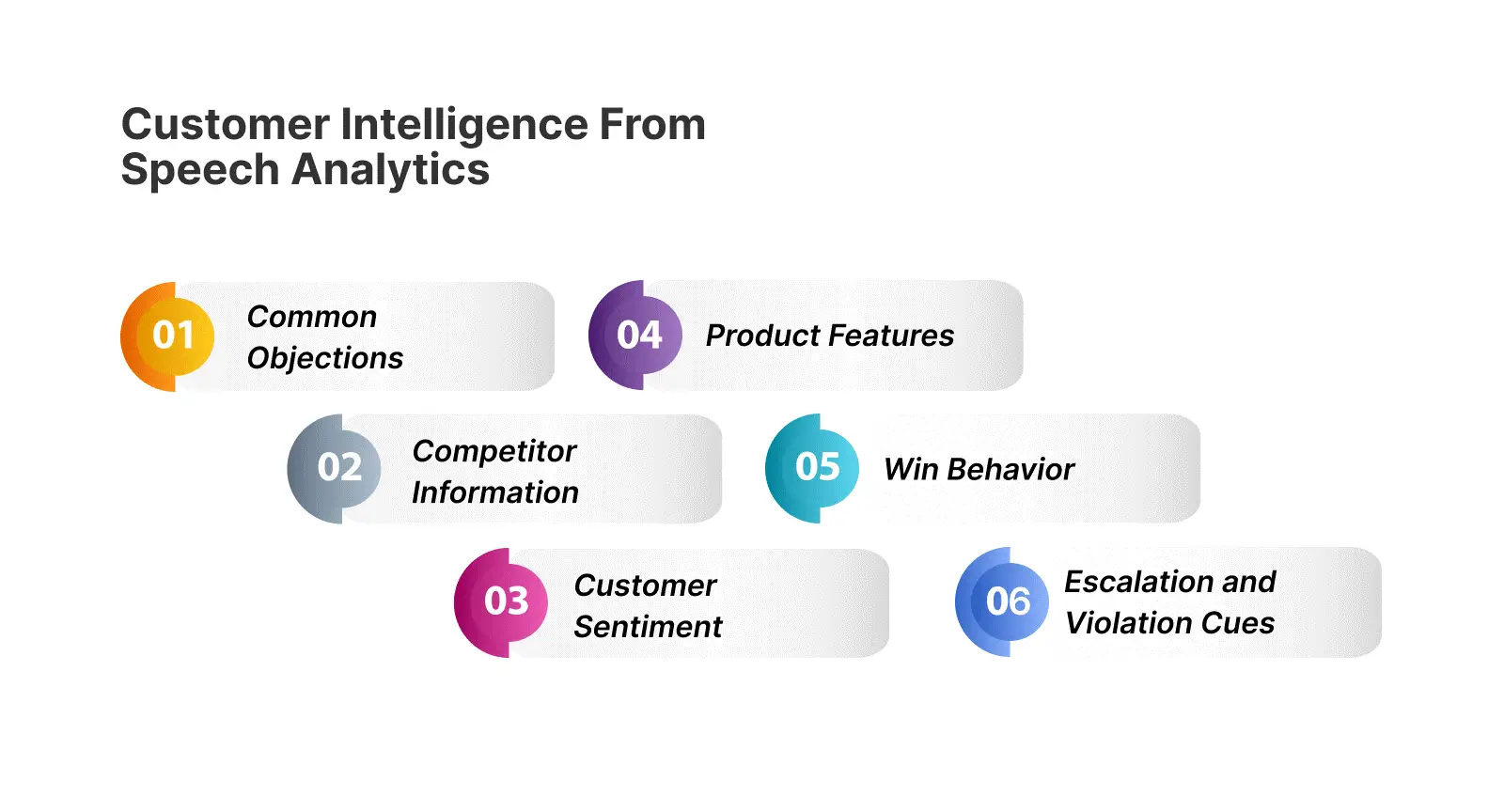
- Concept: These technologies enable machines to understand and respond to human language naturally.
- Application: Virtual assistants and chatbots provide instant, 24/7 customer service.
- Example: Apple's Siri uses speech recognition to understand user queries and NLP to provide relevant answers.
4. Call Center Analytics
- Concept: Deep learning algorithms analyze call center interactions to derive customer satisfaction and agent performance insights.
- Application: Identifying common customer complaints or queries to improve service.
- Example: CallMiner Eureka uses speech analytics to analyze call center conversations for improved customer service.
5. Language Modeling and NLP
- Concept: NLP teaches machines to understand and interpret human language.
- Application: Analyzing customer feedback, emails, or social media posts for sentiment analysis.
- Example: Grammarly uses language modeling to offer writing suggestions.
6. Voice Analytics
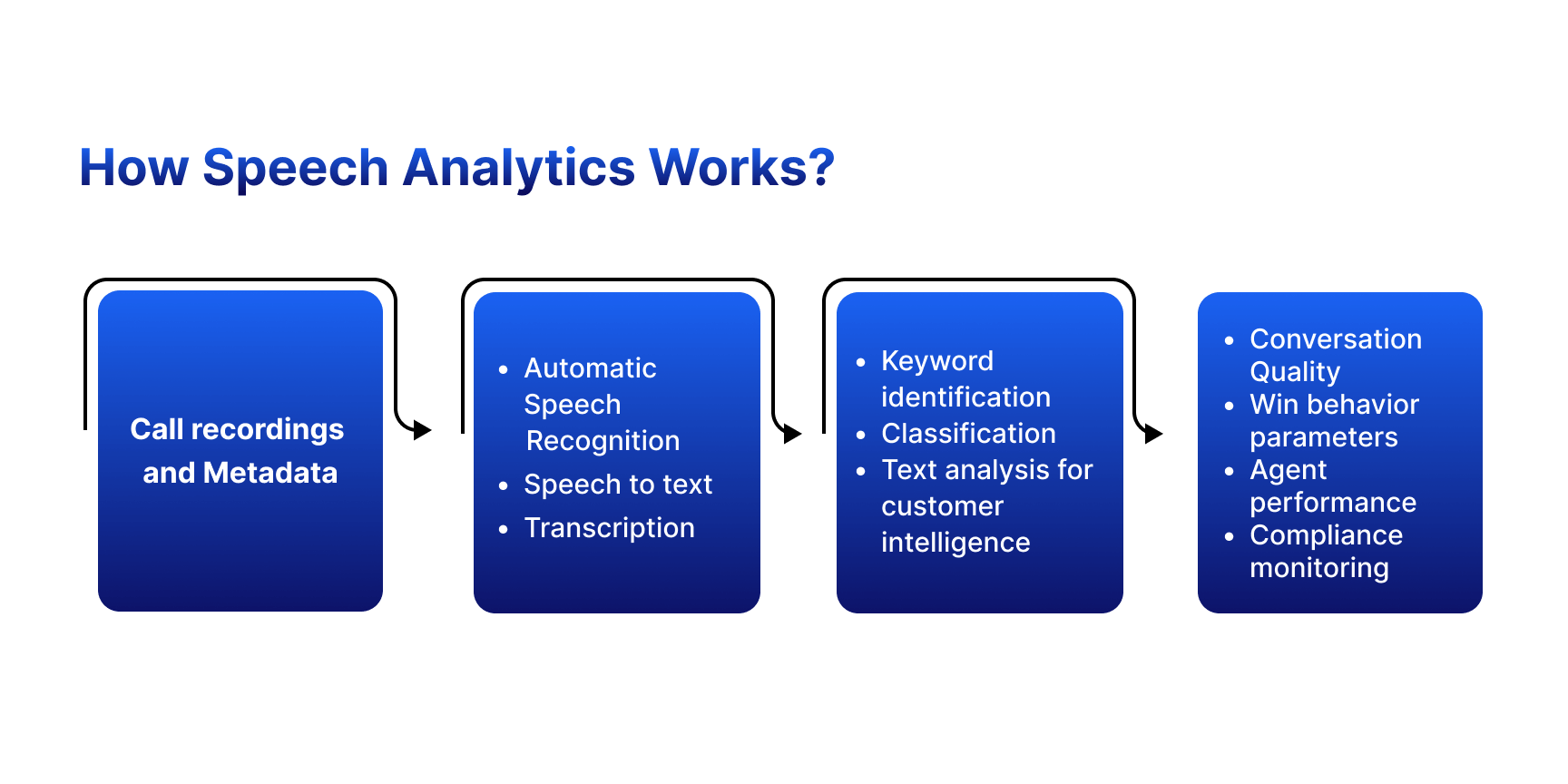
- Concept: Voice analytics uses deep learning to analyze vocal cues for insights into customer emotions and sentiments.
- Application: Assessing customer satisfaction and tailoring responses accordingly.
- Example: Amazon Alexa’s voice profiles personalize responses based on who speaks.
7. Customer Feedback Analysis
- Concept: Deep learning tools analyze feedback from various channels to understand customer satisfaction.
- Application: Companies can quickly adapt to customer needs and improve their products or services.
- Example: Zendesk uses AI to analyze customer service tickets for faster resolution.
8. Generative AI
- Concept: AI creates content based on training data, from text to images.
- Application: Generating automated responses to customer queries that are accurate and contextually relevant.
- Example: GPT-3 by OpenAI can draft emails, create content, and even code based on user inputs.
Deep learning and generative AI are not just enhancing the customer experience; they are revolutionizing it. By leveraging predictive analytics, personalization, advanced NLP, and voice analytics, businesses can offer their customers more tailored, responsive, and engaging experiences. As these technologies evolve, the potential for further innovation in customer experience is vast and promising.
Results first, payment later.
How NLP and Voice Analytics Are Revolutionizing Customer Experience?
Natural Language Processing (NLP) and voice analytics, powered by advancements in deep learning and generative AI, are dramatically changing how businesses understand and interact with customers.
Here's an in-depth look at their impact, with examples and use case scenarios.
1. Enhanced Understanding with Voice Analytics

- Emotion Recognition: Voice analytics can detect subtle nuances in a customer's tone, indicating emotions like frustration or satisfaction. This helps in tailoring responses to suit the customer's emotional state.
- Sentiment Analysis: Businesses can better address their concerns by analyzing the sentiment behind a customer's words.
- Advanced Emotion Detection: Beyond recognizing words, voice analytics now uses deep learning to understand nuances in tone, pace, and volume, offering insights into the customer's emotional state. For example, a customer's hurried speech might indicate urgency or frustration, prompting immediate attention.
- Real-Time Feedback during Calls: Advanced algorithms can provide real-time feedback to customer service agents about the customer's mood, allowing them to adjust their approach on the fly. Imagine a scenario where a system alerts an agent when a customer's tone suggests rising annoyance, guiding the agent to switch to a more soothing approach.
- Voice Biometrics for Personalization: Voice analytics can also be used for voice biometrics, identifying customers by their voice patterns. This enhances security and allows for a personalized customer experience as soon as the customer starts speaking.
- Example: In call centers, voice analytics software can flag calls where a customer sounds particularly distressed, prompting immediate supervisor intervention.
2. NLP in Chatbots for Personalized Interactions
- Context-Aware Responses: NLP enables chatbots to understand the context of customer inquiries, ensuring relevant and personalized responses.
- Language Modeling: Advanced language models can generate human-like text, making interactions with chatbots more natural and effective.
- Conversational Context Understanding: NLP now enables chatbots to understand the context of a conversation over multiple turns of dialogue. For instance, if a customer mentions a problem with a product in one sentence and asks a question in the next, the chatbot can connect these two to provide a coherent response.
- Language Generation for Dynamic Interactions: Generative AI, combined with NLP, allows chatbots to generate dynamic, natural-sounding responses rather than relying on pre-defined scripts. This makes interactions more engaging and less robotic.
- Cross-Platform Consistency: NLP-powered chatbots can maintain consistency in customer interactions across various platforms like email, social media, and messaging apps, ensuring a seamless customer experience.
- Example: A chatbot for an online retailer that helps track orders and recommends products based on the customer's browsing history and previous interactions.
3. Comprehensive Customer Feedback Analysis
- Multi-Platform Analysis: NLP tools can analyze customer feedback from various sources, including social media, surveys, and customer support transcripts.
- Sentiment Analysis Across Channels: NLP tools can analyze sentiment in customer feedback across diverse channels, including voice calls, social media posts, and written reviews. This holistic view enables businesses to form a more complete understanding of customer satisfaction and areas for improvement.
- Predictive Insights for Future Strategies: By analyzing historical customer feedback data, NLP and machine learning algorithms can predict future customer trends and behaviors, aiding in strategic decision-making. For example, analyzing feedback on a product launch can help predict customer response to future updates or new releases.
- Example: A hotel chain using NLP to analyze reviews and feedback across platforms, identifying critical areas for improvement like room service or cleanliness.
4. Speech Recognition for Efficient Service
- Natural Language Understanding in Voice Commands: Speech recognition combined with NLP allows systems to understand and execute complex voice commands. For example, a user could say, "Find Italian restaurants nearby with outdoor seating," the system would understand and execute this multi-faceted request.
- Automated, Intelligent Transcription: Speech-to-text technologies powered by deep learning can accurately transcribe customer calls, including industry-specific jargon. This transcription can then be analyzed for insights into common customer issues and preferences.
- Example: A banking app that allows customers to perform transactions or get information through voice commands.
5. Call Center Analytics for Quality Assurance
- Performance Analysis: Deep learning algorithms can evaluate call center interactions, assessing call duration, resolution rate, and customer satisfaction.
- Training and Feedback: Insights from call center analytics can be used to train customer service representatives, improving overall service quality.
- Example: A telecommunications company analyzes call center data to identify common customer issues and train representatives accordingly.
6. Generative AI for Dynamic Customer Interaction
- Custom Content Creation: Generative AI can create personalized customer responses or content, enhancing engagement.
- Dynamic Interaction Models: AI can simulate various customer scenarios, helping businesses prepare for customer interactions.
- Example: An insurance company using generative AI to create personalized policy recommendations based on individual customer data.
7. Leveraging Machine Learning for Continuous Improvement
- Adaptive Learning: ML algorithms learn from each customer interaction, continuously improving the accuracy and effectiveness of responses.
- Predictive Customer Service: Anticipating customer needs based on historical data and trends.
- Example: An e-commerce platform that continuously refines its customer support chatbot based on user interactions and feedback.
Integrating NLP and voice analytics in customer experience analysis signifies a monumental shift towards more empathetic, efficient, and personalized customer service. By harnessing these technologies, businesses can respond to customer needs more effectively and anticipate and shape future customer interactions. The future of customer service is here, and it's conversational, empathetic, and intelligent.
What are the Benefits of Using Deep Learning and Generative AI to Transform the Customer Experience Space?
In the dynamic realm of customer experience, the advent of deep learning and generative AI marks a revolutionary shift, heralding a new era of enhanced interaction and understanding between businesses and their customers. These cutting-edge technologies are not merely incremental improvements but transformative tools that are reshaping the landscape of customer engagement and satisfaction.
By harnessing the power of deep learning and generative AI, businesses are unlocking unprecedented opportunities to deepen customer understanding, streamline service efficiency, personalize marketing strategies, and garner real-time insights, thereby setting new benchmarks in customer experience excellence.
1. Enhanced Customer Understanding
- Deep Learning for Behavior Analysis: Deep learning algorithms can sift through massive datasets to identify patterns in customer behavior. This can lead to a more nuanced understanding of what drives customer decisions and preferences.
- Example: An online retailer using deep learning to analyze browsing and purchase history, thereby identifying trends and preferences among different customer segments.
- Predictive Analytics for Anticipating Needs: By leveraging historical data, predictive analytics can forecast future customer behaviors and preferences, allowing businesses to meet customer needs proactively.
- Scenario: A streaming service uses predictive analytics to suggest shows and movies based on a user's viewing history, increasing engagement and satisfaction.
2. Improved Customer Service
- Conversational AI for Enhanced Interactions: Chatbots powered by conversational AI can handle customer inquiries efficiently, providing quick and accurate responses, which enhances the overall customer service experience.
- Example: A bank employs an AI chatbot to handle routine customer queries about account balances and transaction histories, freeing up human agents for more complex issues.
- NLP for Understanding Queries: NLP allows systems to understand and respond to customer queries in natural language, making interactions more intuitive and user-friendly.
- Scenario: A travel booking website uses NLP to understand and respond to complex travel queries, such as multi-city flight bookings.
3. Efficiency and Cost Reduction
- Automating Routine Tasks: Machine learning algorithms can automate routine customer service tasks, reducing human intervention and lowering costs.
- Example: A utility company uses ML algorithms to automatically categorize and respond to common customer service requests, like billing inquiries.
- Fast Data Analysis: Deep learning can process and analyze large volumes of data much faster than traditional methods, leading to quicker decision-making.
- Scenario: An e-commerce company processes millions of customer reviews and feedback using deep learning, rapidly identifying areas for improvement.
4. Innovative Marketing
- Personalization: Deep learning can create highly personalized marketing strategies that resonate with individual customers based on their unique preferences and behaviors.
- Example: A fashion retailer uses generative AI to create personalized fashion recommendations for customers based on their past purchases and browsing behavior.
- Language Modeling for Content Creation: Generative AI can create engaging marketing content tailored to the language and style that best suits the target audience.
- Scenario: A marketing agency uses language models to generate creative ad copies for different demographics, increasing engagement and conversion rates.
5. Real-Time Insights
- Voice Analytics in Call Centers: By analyzing voice interactions in real-time, companies can gain immediate insights into customer emotions and satisfaction levels.
- Example: A telecom company uses voice analytics during customer support calls to identify dissatisfaction or confusion, allowing for immediate corrective action.
- Speech Recognition for Instant Feedback: Speech recognition technology can transcribe and analyze customer feedback, providing real-time insights.
- Scenario: A healthcare provider uses speech recognition to transcribe and analyze patient feedback during and after appointments, improving patient care and experience.
By leveraging these technologies and strategies, businesses can significantly enhance the customer experience, leading to increased customer loyalty, improved service quality, and a more decisive competitive edge in the market.
Implementing Deep Learning and Generative AI successfully in the Customer Experience Space!
Implementing deep learning and generative AI to enhance customer experience is multifaceted. Here's an in-depth look at each step, illustrating them with examples and scenarios.
1. Data Collection and Management
- Importance: The foundation of any AI system is data. Quality data is crucial for training accurate and practical models.
- ~Foundation for AI Systems: The efficacy of deep learning and generative AI systems heavily relies on the quality and volume of the data they are trained on. These models learn and make predictions based on the data they are fed, which means the accuracy of their outputs is directly linked to the quality of the input data.
- ~Diverse Data for Generalization: A diverse dataset is essential to build models that can generalize well and function effectively in various scenarios. This diversity includes different types of customer interactions, varying demographics, a range of spoken languages in voice recognition systems, and multiple data formats (text, audio, images).
- ~Data Representativeness: The data must accurately represent the real-world scenarios where the AI system will be deployed. For example, in customer experience, data should encompass the full spectrum of customer interactions, including inquiries, complaints, feedback, and transaction history.
- ~Avoiding Bias: Poor data collection can lead to biased AI models. Biases in data can skew the AI's understanding and predictions, leading to unfair or ineffective outcomes. Ensuring that the data is representative and free from biases is critical to developing equitable AI systems.
- Challenges in Data Collection and Management
- ~Data Privacy and Ethics: With stringent data protection laws like GDPR and concerns about ethical data use, organizations must navigate the complex landscape of legally and ethically collecting data. This involves obtaining Consent, anonymizing personal data, and ensuring transparency in data usage.
- ~Data Quality Over Quantity: While the amount of data is essential, the quality is paramount. Data cleaning and preprocessing are crucial steps to remove inaccuracies, duplicates, and irrelevant information, ensuring high-quality data is fed into AI models.
- ~Real-Time Data Handling: In domains like speech recognition and conversational AI, the ability to process and learn from data in real time can significantly enhance performance. This requires robust data management systems capable of handling large volumes of data swiftly and efficiently.
- Deep Learning & Predictive Analytics: Utilize customer interaction data, purchase history, and browsing behavior to train deep learning models for predictive analytics.
- ~Utilization of Customer Data
- ~~Data Sources: Key data sources include customer interaction records, purchase history, and online browsing behavior. This data is rich in insights about customer preferences, habits, and potential future actions.
- ~~Pattern Recognition: Deep learning algorithms analyze this data to identify patterns and correlations that might not be apparent to human analysts. For instance, subtle browsing patterns could predict a customer's likelihood to purchase a specific product.
- ~Application in Predictive Analytics
- ~~Predicting Future Purchases: By analyzing past purchase history and browsing data, deep learning models can predict what a customer will likely buy next. This prediction can be particular, considering factors like the time of year, price range, and customer's browsing habits.
- ~~Personalization: These predictions enable a high degree of personalization. For example, an online retailer can show customers products they are more likely to be interested in, leading to a more personalized shopping experience.
- ~Challenges and Considerations
- ~~Data Quality and Volume: The effectiveness of deep learning in predictive analytics heavily depends on the quality and quantity of data. Inaccurate or sparse data can lead to poor predictions.
- ~~Model Complexity: Deep learning models can be complex and require significant computational resources. It's essential to balance the complexity of the model with the available computational capacity.
- ~~Privacy and Ethical Concerns: The use of customer data must be balanced with privacy concerns. Businesses must ensure they comply with data protection regulations and use data ethically.
- Example: An online retailer collects customer browsing patterns and purchase history data to predict future buying trends and make personalized product recommendations.
2. Choosing the Right Tools and Platforms
- Relevance to Business Needs: Select tools that align with specific business goals, whether it's enhancing customer support, personalizing marketing strategies, or improving product recommendations.
- ~Understanding Business Objectives
- ~~Businesses must first clearly define their objectives. For example, a retail business might aim to improve customer satisfaction, while a service provider might focus on reducing response times in customer support.
- ~~Objectives should be SMART: Specific, Measurable, Achievable, Relevant, and Time-bound.
- ~Matching AI Tools to Objectives
- ~~For enhancing customer support, tools that specialize in conversational AI and natural language processing (NLP) would be ideal. These tools can power chatbots and virtual assistants to respond quickly and accurately to customer inquiries.
- ~~For personalizing marketing strategies, deep learning algorithms that analyze customer data to identify preferences and patterns are crucial. These insights can then tailor marketing messages and offers to individual customers.
- ~~Improving product recommendations involves using machine learning models that analyze past purchasing behavior, browsing history, and customer feedback to suggest relevant products to customers.
- ~Consideration of Integration and Scalability
- ~~The selected AI tools should integrate seamlessly with existing systems and databases.
- ~~Scalability is crucial. The tools should be able to handle increased loads and evolve as the business grows and its data sets expand.
- ~ROI Measurement
- ~~Businesses must have metrics in place to measure the return on investment (ROI) of the AI tools. This could be increased sales, improved customer satisfaction scores, reduced operational costs, or other relevant metrics.
- Generative AI & Conversational AI: Choose platforms that support generative AI for creating dynamic and adaptive conversational agents.
- ~Definition and Role
- ~~Generative AI: It refers to AI algorithms capable of generating new content (text, speech, etc.) by learning from existing datasets. In the context of conversational AI, it enables the creation of responses that are not pre-scripted but generated in real time based on the learned patterns from vast amounts of conversational data.
- ~~Application in Conversational AI: This technology allows chatbots and virtual assistants to generate natural, contextually relevant, and personalized responses, leading to more engaging and human-like user interactions.
- ~Choosing the Right Platforms
- ~~Learning Capabilities: The platform should have advanced machine learning algorithms capable of understanding and adapting to different conversational contexts.
- ~~Integration of NLP: Natural Language Processing (NLP) is essential for interpreting user intent and generating appropriate responses.
- ~~Support for Multiple Languages and Dialects: The platform should handle various languages and dialects effectively to cater to a global audience.
- ~~Scalability: The ability to scale up as the number of interactions increases is crucial for handling peak loads and growing user bases.
- ~~Customization and Flexibility: The platform should offer customization options to meet specific business needs and industry requirements.
- ~Dynamic and Adaptive Responses
- ~~Personalization: Generative AI enables the agent to learn from previous interactions with each user, allowing it to tailor conversations based on individual preferences and history.
- ~~Contextual Awareness: The agent can understand the context of the conversation and maintain continuity over multiple interactions, making the conversation flow more natural.
- ~~Emotion Recognition: Advanced platforms can analyze sentiment and tone, enabling the conversational agent to respond empathetically, enhancing customer experience.
- Example: A business selects a platform that integrates NLP and speech recognition to develop an AI-powered virtual assistant that can handle customer queries effectively.
3. Integrated Example Scenario
A Retail Company Implementing a Deep Learning-Based Recommendation System
- Data Collection: The company collects data on customer purchases, online browsing patterns, and feedback.
- Tool Selection: Chooses a platform specializing in deep learning and generative AI for personalization.
- Skilled Personnel: Hires data scientists skilled in ML and trains customer service staff in using AI tools.
- Testing and Iteration: Regularly tests the recommendation system, using customer feedback and purchase patterns to refine it. Employs voice analytics to understand verbal feedback in customer service calls.
- Ethical Considerations: Implements strict data privacy measures and uses AI responsibly to ensure customer trust.
The company leverages deep learning for predictive analytics in this scenario, ensuring personalized shopping experiences. Conversational AI and NLP enhance customer interaction online, while voice analytics helps understand and respond to customer needs during support calls. This comprehensive approach ensures a significant improvement in the overall customer experience.
How Convin Can Enhance Customer Service with Deep Learning, and Generative AI?
Convin, as a provider of advanced AI solutions, can significantly enhance customer service through its products that leverage deep learning, generative AI, and Natural Language Processing (NLP). Here's an in-depth look at how these technologies can be utilized:
1. Deep Learning for Predictive Customer Service
Convin's utilization of deep learning for speech-to-text conversion represents a significant advancement in processing and understanding customer interactions.
Deep Learning in Speech-to-Text Conversion
a. Audio Signal Processing
- Initial Processing: Deep learning algorithms first process the raw audio signals, filtering out background noise and normalizing variations in volume and speech speed variations.
- Feature Extraction: The algorithms then extract relevant features from the audio signal. This includes identifying phonemes (basic units of sound), intonation, and rhythm.
b. Neural Network Models
- Acoustic Modeling: Convin likely employs deep neural networks (DNNs) to map the acoustic signals to phonetic units. These models are trained on large datasets of spoken language to understand various accents, dialects, and speech patterns.
- Language Modeling: To convert phonetic representations into coherent text, deep learning models use language modeling. This involves understanding the context and the likelihood of certain words following others, a task for which recurrent neural networks (RNNs) or transformers might be used.
c. Real-Time Processing
- Speed and Efficiency: Deep learning models, once trained, can transcribe speech to text in real time, allowing for immediate analysis and response to customer queries or feedback.
Application in Customer Interaction
In practical terms, this deep learning-powered speech-to-text technology allows Convin to transcribe customer calls, meetings, or audio efficiently. This transcription is not just a verbatim record but also includes insights into customer sentiment and intent, thanks to the advanced processing capabilities of the deep learning models. Such a tool is invaluable in customer service and support, enabling businesses to analyze customer interactions more deeply, respond more effectively, and improve overall service quality.
2. Generative AI for Dynamic Interaction
Convin, a platform that leverages the power of artificial intelligence, utilizes explicitly generative AI to enhance various aspects of customer interaction and management. Here's how it can apply generative AI to lead scoring, CSAT (Customer Satisfaction), and collection scores.
a. Generative AI for Lead Scoring
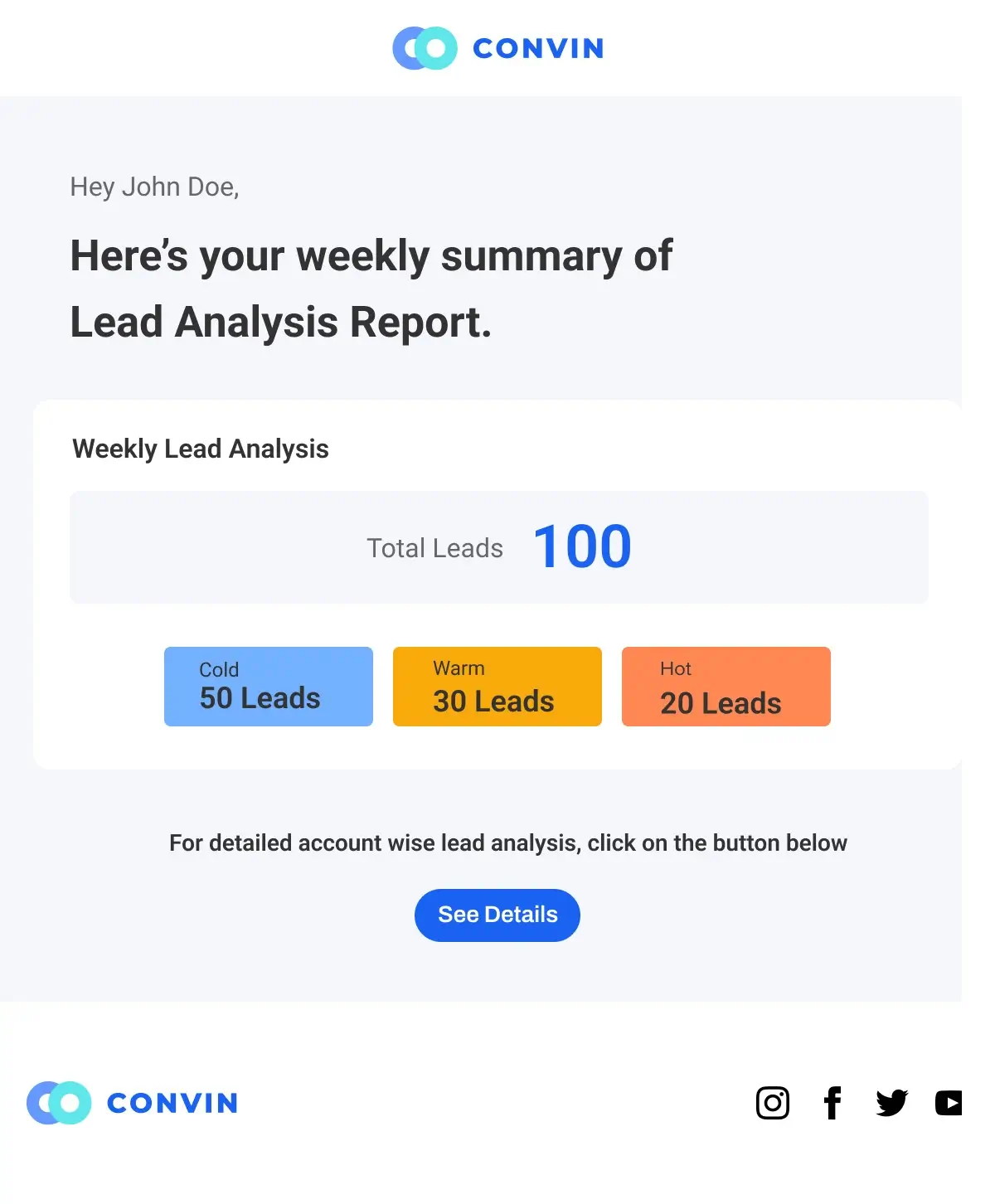
- Automated Analysis of Customer Data: Convin uses generative AI to analyze vast customer interaction data, such as call transcripts, emails, and chat histories. This analysis helps identify patterns and behaviors indicative of a lead's potential.
- Predictive Modeling: Convin can predict which leads are more likely to convert into customers by employing deep learning algorithms. This predictive modeling considers various factors, including past interactions, response times, and customer queries.
- Personalization of Customer Interactions: Generative AI enables personalized communication with leads based on their previous interactions and preferences. This targeted approach can significantly improve conversion rates.
- Continuous Learning and Adaptation: The AI models continually learn from new data, refining the lead-scoring process to become more accurate and efficient.
b. Generative AI for CSAT Score Enhancement
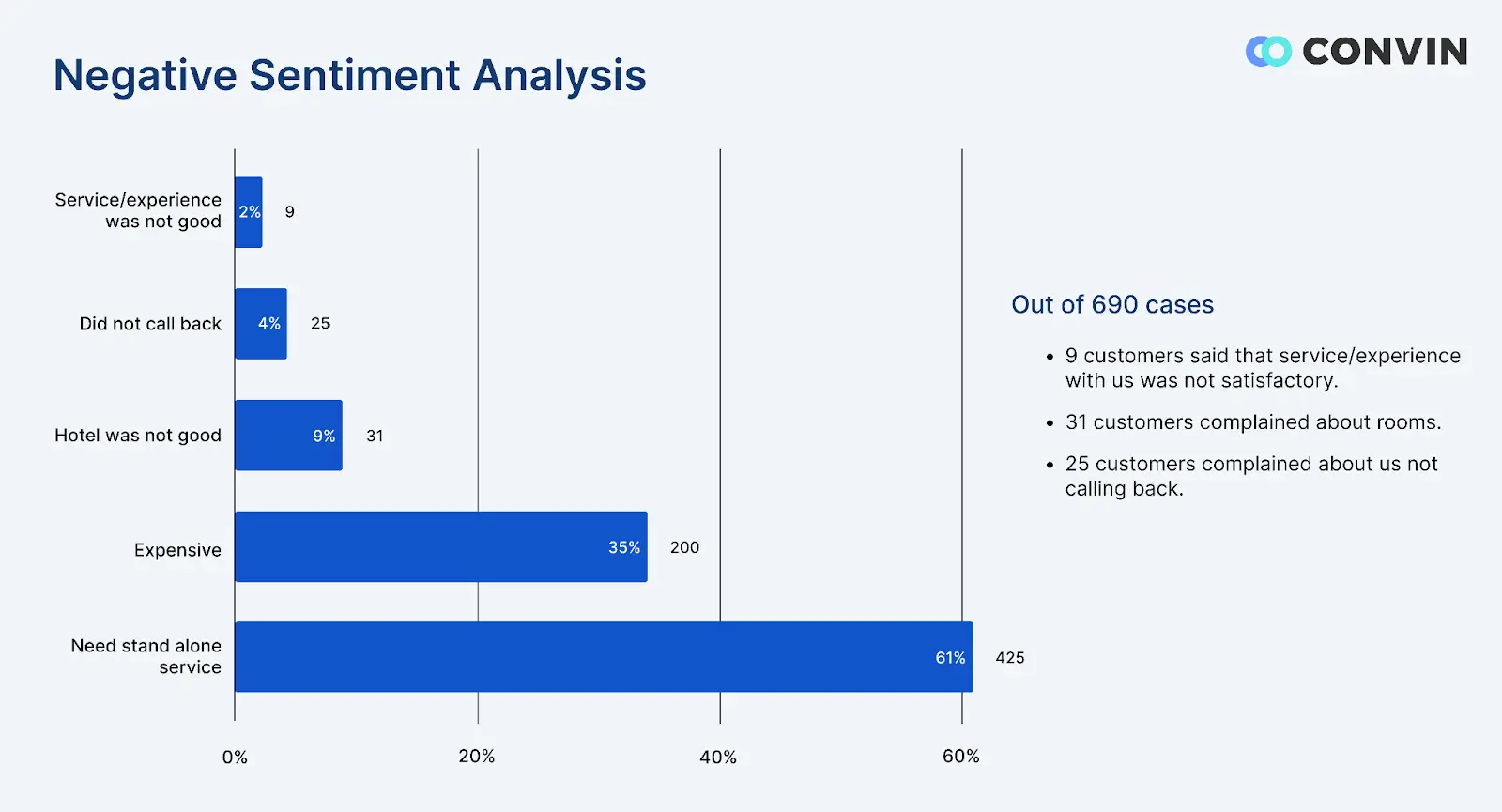
- Sentiment Analysis: Convin can use NLP to analyze customer feedback and interactions, identifying sentiments and emotions. This analysis helps in calculating a more accurate and nuanced CSAT score.
- Predictive CSAT Modeling: The platform can predict future CSAT scores by analyzing trends and patterns in customer interactions and feedback, allowing businesses to address potential issues proactively.
- Real-Time Feedback Interpretation: Generative AI enables interpreting customer feedback in real-time, providing immediate insights into customer satisfaction levels.
c. Generative AI for Collection Score Improvement
- Customer Payment Behavior Analysis: AI algorithms can analyze historical payment data and interaction patterns to score customers' likelihood of making timely payments.
- Risk Assessment and Management: Businesses can proactively manage risks and focus their collection efforts more effectively by predicting which customers might default or delay payments.
Convin’s use of generative AI, deep learning, and NLP is central to providing more accurate, efficient, and personalized customer service experiences in all these applications. The platform's ability to analyze large datasets, learn from interactions, and predict future behaviors and preferences makes it a powerful tool for businesses looking to enhance customer relationship management and service strategies.
By leveraging the power of deep learning, and generative AI, Convin can significantly enhance the quality of customer service across various industries. This integration improves efficiency and customer satisfaction and gives businesses deeper insights into their customer base, enabling them to make more informed decisions and offer personalized services.
Schedule a call with us to explore Convin's innovative solutions and take the first step toward redefining your customer experience space. Elevate your customer experience to new heights with Convin!
FAQs
1. What are the three types of deep learning?
Three Types of Deep Learning
- Supervised Learning: Involves training models on labeled data.
- Unsupervised Learning: Uses unlabeled data to find patterns or structures.
- Reinforcement Learning: Trains models to make sequences of decisions based on rewards.
2. What is the difference between machine learning and deep learning?
- Machine Learning: Involves algorithms that learn from and make predictions on data.
- Deep Learning: A subset of ML using neural networks with multiple layers to learn from large amounts of data.
3. How AI is transforming the customer experience?
AI personalizes customer interactions and automates responses, enhancing efficiency and satisfaction.
4. How can machine learning change customer experience?
ML analyzes customer data to predict behavior and personalize experiences, improving engagement and loyalty.
5. How does digital transformation improve customer experience?
Digital transformation streamlines processes and integrates technologies, making customer interactions more efficient and personalized.
6. How generative AI could revolutionize your customer experience?
Generative AI can create personalized content and responses, significantly enhancing interaction quality and relevance.