
In the dynamic world of customer service, call centers are evolving rapidly, thanks to advanced analytics and machine learning. These technologies are not just buzzwords but powerful tools reshaping how call centers operate and interact with customers.
This article delves into the transformative impact of these technologies on call centers, using specific examples and scenarios to illustrate their benefits.
Learn how Convin can help you transform customer service using ML and Advanced Analytics.
What is the Difference Between Advanced Analytics and Machine Learning?
Navigating the realms of data-driven decision-making involves understanding the nuanced distinctions between advanced analytics and machine learning. While both are cornerstones of modern data analysis, they serve different purposes and offer unique insights within the vast information processing landscape.
Advanced analytics, focusing on predictive models and intricate data examination, offers a broad perspective on future trends and behaviors. In contrast, machine learning delves deeper, leveraging algorithms to learn from data and improve outcomes autonomously.

This table highlights the distinct roles and applications of advanced analytics and machine learning in call centers, emphasizing their complementary nature. While advanced analytics helps understand and predict customer behavior, machine learning continuously improves customer interaction and operational efficiency.
Utilizing Advanced Analytics and Machine Learning in Call Centers!
Advanced analytics and machine learning are revolutionizing the call center landscape, offering unprecedented opportunities for enhancing customer experience and operational efficiency. Here's an in-depth look at how these technologies are being utilized in call centers.
1. Advanced Analytics in Call Centers

As the digital revolution continues to redefine industry landscapes, advanced analytics has emerged as a cornerstone in the transformation of call centers. This innovative approach leverages deep data insights to understand customer behavior in unprecedented ways forecast future trends, optimize operations, and personalize customer interactions.
Within this realm, various types of advanced analytics, predictive, prescriptive, and descriptive, each uniquely enhances the call center experience. By embracing these diverse analytical strategies
a. Predictive Analytics
- Usage: Predictive analytics in call centers involves analyzing historical data to forecast future trends and events. This includes predicting customer call patterns, aiding workforce optimization, and resource allocation.
- Impact
- ~Workforce Optimization: Call centers can ensure appropriate staffing by predicting high-traffic periods, and reducing wait times.
- ~Resource Allocation: Allocates resources efficiently to handle anticipated call volumes, improving operational efficiency.
- Advanced Analytics Example: A call center may use predictive analytics to forecast increased call volumes during promotional or seasonal spikes, allowing it to adjust staffing levels and training schedules accordingly.
b. Prescriptive Analytics
- Usage: This type of analytics provides recommendations based on data analysis for optimal outcomes. In call centers, it can guide agents with the best responses or solutions to customer issues based on past interactions and outcomes.
- Impact
- ~Improved Customer Resolutions: Offers real-time suggestions to agents for handling customer queries, leading to more effective problem-solving.
- ~Strategic Decision Making: Helps formulate strategies for customer engagement and service improvements.
- Advanced Analytics Example: An algorithm might analyze customer interaction history and current context to recommend personalized offers or solutions, enhancing customer satisfaction and potential upselling opportunities.
c. Descriptive Analytics
- Usage: Involves the analysis of past performance data to identify trends, patterns, and insights. This encompasses call durations, customer satisfaction scores, and agent performance metrics.
- Impact
- ~Performance Improvement: Identifies performance gaps and training needs for agents, improving overall call center efficiency.
- ~Customer Insight: Gathers customer behavior and preferences insights, aiding in better service delivery.
- Advanced Analytics Example: Review call data to identify common customer concerns or questions, which can be addressed through targeted training programs or updates to FAQs and automated response systems.
2. Machine Learning Applications in Call Centers
The advent of machine learning applications in call centers heralds a new era of innovation and efficiency, revolutionizing how customer interactions are handled, analyzed, and leveraged for business growth.
By integrating machine learning technologies, call centers can predict customer needs, personalize interactions, and streamline operations with unprecedented precision.
a. Automated Customer Interaction
- Usage: Machine learning powers chatbots and voice recognition systems for handling routine customer queries, enabling human agents to focus on more complex issues.
- Impact
- ~Efficiency: Reduces agent workload and provides faster response times.
- ~Availability: Offers 24/7 customer service capabilities.
- Example: A chatbot, trained with thousands of customer service transcripts, can effectively handle basic inquiries like billing questions, and appointment scheduling, and provide account updates.
b. Sentiment Analysis
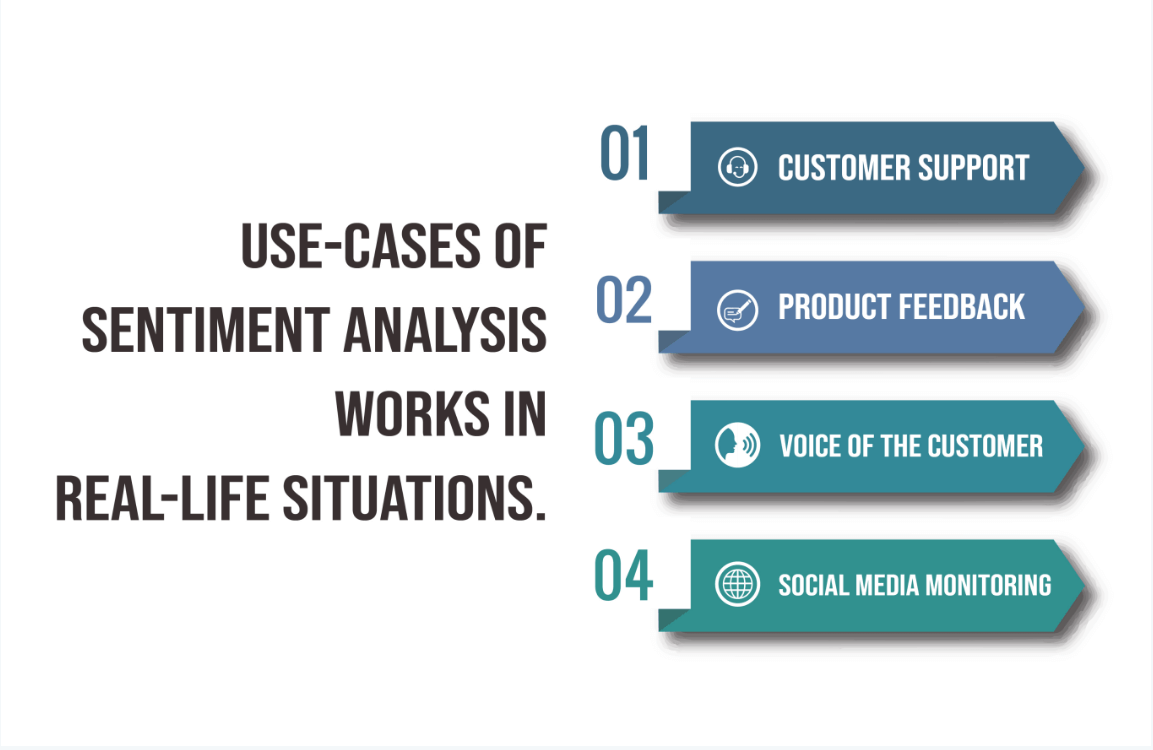
- Usage: Machine Learning algorithms analyze customer conversations' sentiment, mood, and context to gauge satisfaction and agent performance.
- Impact
- ~Personalized Service: Allows for more tailored customer service based on emotional cues.
- ~Proactive Intervention: Alerts supervisors to intervene in calls requiring additional support or escalation.
- Example: Real-time sentiment analysis tools can detect frustration or confusion in a customer’s voice or text, signaling the agent to adjust their approach or escalate the call to a supervisor if necessary.
By leveraging advanced analytics and machine learning, call centers can transform their operations, enhancing customer service quality, operational efficiency, and overall business performance.
Results first, payment later
Transformation in Call Centers through Advanced Analytics: Enhancing Customer Service with Analytics!
Integrating advanced analytics into call center operations marks a pivotal transformation in the rapidly evolving customer service landscape. This strategic fusion not only elevates the efficiency of call centers but fundamentally enhances how customer interactions are managed and optimized.
1. Use Case Example: Predictive Analytics for Staffing Optimization
Scenario: A call center experiences fluctuating call volumes, leading to periods of high customer wait times and agent idle times.
Advanced Analytics Application: The call center implements predictive analytics, a form of advanced analytics, to anticipate call volume fluctuations.
Data Utilization: Historical call data, including time, duration, and frequency, are analyzed to identify patterns and trends.
Machine Learning Integration: Machine learning algorithms refine these predictions by learning from ongoing data, improving accuracy over time.
Outcome: The call center can now predict high call volume periods and adjust staffing levels accordingly.
2. Reduced Wait Times and More Efficient Customer Service
a. Customer Experience Enhancement
- Machine Learning for Dynamic Staffing Adjustments
- ~Machine learning algorithms continuously learn from real-time data, allowing for dynamic adjustments in staffing.
- ~This adaptability ensures that unexpected surges in call volume are promptly addressed
- ~Example: An ML model might detect an unexpected spike in calls due to a product recall and immediately suggest increasing staffing levels.
- Impact on Customer Wait Times
- ~These technologies significantly reduce the time customers spend waiting in the queue.
- ~Faster response times lead to improved customer satisfaction and perception of the brand.
b. Improved Customer Satisfaction as Calls are Handled More Efficiently
- Enhanced Agent Performance with Analytics
- ~Advanced analytics provide insights into the most common customer queries and effective response strategies.
- ~Call center agents can be better prepared to handle inquiries efficiently, reducing call handling time.
- ~Example: Analytics might reveal that a simple script adjustment could quickly solve a common customer issue.
- Personalized Customer Interactions through Machine Learning
- ~Machine learning algorithms can analyze past interactions to offer personalized service to repeat customers.
- ~This tailored approach makes customers feel valued and understood, enhancing their overall experience.
- ~Example: An ML system could suggest personalized product recommendations based on a customer’s purchase history.
- Overall Impact on Customer Service
- ~The reduced wait times and improved call handling resulted in a significantly enhanced customer experience.
- ~Customers are likely to have their issues resolved more quickly and with more personalization.
- ~This leads to higher customer satisfaction scores and potentially increases customer loyalty and repeat business.
Integrating advanced analytics and machine learning in call centers optimizes operational efficiency and plays a crucial role in elevating the customer experience. By predicting call volumes and enhancing agent performance, these technologies ensure that customers receive timely and effective service, which is essential in today's competitive market.
3. Operational Efficiency in Call Centers through Predictive Analytics
Predictive analytics, a key component of advanced analytics, significantly enhances operational efficiency in call centers.
a. Optimal Use of Resources Reduces Operational Costs
- Resource Allocation: Predictive analytics enables call centers to allocate resources, such as manpower and technology, more efficiently by predicting call volumes.
- Cost Reduction: Call centers can significantly reduce labor costs by avoiding overstaffing during low-volume periods and understaffing during peak times.
- Efficient Use of Technology: Advanced analytics helps determine the best times to perform system maintenance and upgrades, ensuring maximum availability during high-demand periods.
b. Predictive Analytics for Staff Planning

- Training Scheduling: Call centers can schedule training sessions without impacting customer service levels by anticipating quieter periods. This ensures continuous professional development without incurring extra overtime or temporary staffing costs.
- Break and Shift Management: Predictive analytics aids in planning breaks and shifts for agents, ensuring that the call center is adequately staffed during peak times while allowing for rest periods during less busy times.
c. Data-Driven Decision Making
- Strategic Planning: Utilizing data from predictive analytics, call center managers can make informed decisions about long-term strategies, such as expansion, scaling, or implementing new technologies.
- Performance Management: Analytics provide insights into peak performance times and areas for improvement, enabling more targeted management of agent performance.
d. Predictive Maintenance
Predictive analytics can also forecast potential system issues, allowing for preemptive maintenance and reducing downtime.
e. Dynamic Scheduling
Leveraging machine learning, dynamic scheduling systems can adapt to real-time changes in call volume, further enhancing efficiency.
f. Financial Planning
Call centers can better plan their budgets and financial forecasts with accurate call volumes and resource requirements predictions.
g. Quality Assurance
By predicting high-risk calls or periods, quality assurance teams can focus their efforts more effectively, improving overall service quality.
Incorporating advanced analytics and machine learning into call center operations streamlines resource utilization and enhances the overall efficiency and effectiveness of the services provided.
4. Enhancing Employee Satisfaction in Call Centers through Advanced Analytics and Machine Learning
a. Better Work Distribution Leads to Reduced Stress
- Application of Analytics: Advanced analytics, particularly workforce analytics, helps understand and predict call volumes, enabling call centers to allocate work more evenly among agents.
- Stress Reduction: When work is evenly distributed, agents are less likely to experience the stress of unexpectedly high call volumes. This leads to a more manageable workload and reduces burnout.
- Machine Learning Role: Machine learning algorithms can predict busy periods and schedule breaks, ensuring agents are not overwhelmed.
b. Increased Job Satisfaction Among Call Center Agents
- Personalized Performance Feedback: Machine learning tools can provide agents with customized feedback based on their call handling, leading to targeted improvements and skill development.
- Recognition and Reward Systems: Advanced analytics can identify top-performing agents, allowing management to recognize and reward these employees, which boosts morale and job satisfaction.
- Career Development Opportunities: With insights from analytics, call centers can offer tailored training and career development opportunities, aligning with the agents’ strengths and career aspirations.
c. Predictive Scheduling for Better Work-Life Balance
- Predictive Analytics for Scheduling: Using predictive analytics, call centers can forecast call volumes and schedule agents accordingly, ensuring that there are enough staff during peak times and no excess during off-peak times.
- Consistent Work Hours: This leads to more consistent and predictable work hours for agents, which is crucial for maintaining a healthy work-life balance.
- Employee Autonomy: Some advanced scheduling tools also give agents more control over their schedules, allowing them to balance personal commitments with work responsibilities.
d. Impact on Call Center Operations
- Reduced Turnover: Improved job satisfaction and work-life balance can lead to lower turnover rates, saving costs related to hiring and training new staff.
- Enhanced Employee Engagement: Satisfied and engaged employees are more likely to be committed to their work, leading to better customer service and higher productivity.
The use of advanced analytics and machine learning in call centers enhances operational efficiency and customer service and plays a crucial role in improving employee satisfaction. These technologies enable better workload distribution, provide recognition and personal growth opportunities, and offer more balanced and flexible work schedules.
As a result, call centers can benefit from a more motivated and stable workforce, which is essential for long-term success. Tools like Convin's AI-backed software are instrumental in achieving these benefits by leveraging conversation intelligence and automated coaching tailored to the business's and its employees' needs.
How does Convin Transform Call Center Operations with Advanced Analytics and Machine Learning?
The customer service landscape is ever-growing and evolving; Convin stands at the forefront of innovation, revolutionizing call center operations by strategically integrating advanced analytics and machine learning.
By harnessing the power of these cutting-edge technologies, Convin not only elevates the efficiency and effectiveness of call center workflows but also significantly enhances the customer experience. This transformation goes beyond mere operational improvements, ushering in a new era of data-driven insights and personalized service delivery that sets new benchmarks in the industry.
1. Automated Quality Management

- Comprehensive Interaction Review: This feature scrutinizes every customer interaction across multiple channels, employing custom auditing templates that identify performance blockers and customer dissatisfaction points.
- CSAT Score: CSAT Score is a measure of customer satisfaction with a product, service, or interaction. It is typically collected through post-interaction surveys, where customers rate their satisfaction on a scale, often from 1 to 5 or 1 to 7.
- Lead Score: Convin employs advanced Machine Learning algorithms to analyze customer interactions and engagement data comprehensively. This analysis includes reviewing conversation patterns, customer queries, and response times to assess a potential customer's interest level and likelihood to convert into a sale.
- Sentiment Analysis: Sentiment analysis, also known as sentimental analysis, is the process of determining and understanding the emotional tone and attitude conveyed within text data. It involves assessing whether a piece of text expresses positive, negative, neutral, or other sentiment categories. Sentiment analysis can be applied to various text types, including customer reviews, social media posts, survey responses, and more.
- Collection Score: Convin’s ML models and analytics are adept at assessing the likelihood of debt repayment by analyzing past interaction history, payment behaviors, and sentiment analysis during calls. This comprehensive evaluation results in a collection score that predicts how likely an individual is to fulfill their payment obligations.
- Machine Learning Integration: Leveraging advanced analytics and machine learning, the system discerns patterns and anomalies in data, enhancing the accuracy of quality assessments and identifying areas for agent improvement.
- Operational Impact: Convin significantly reduces the manual effort required for call audits by automating the quality management process, leading to more consistent and objective quality control measures. This automation helps pinpoint specific training needs for agents and improves overall customer service quality.
2. Conversation Behavior Analysis
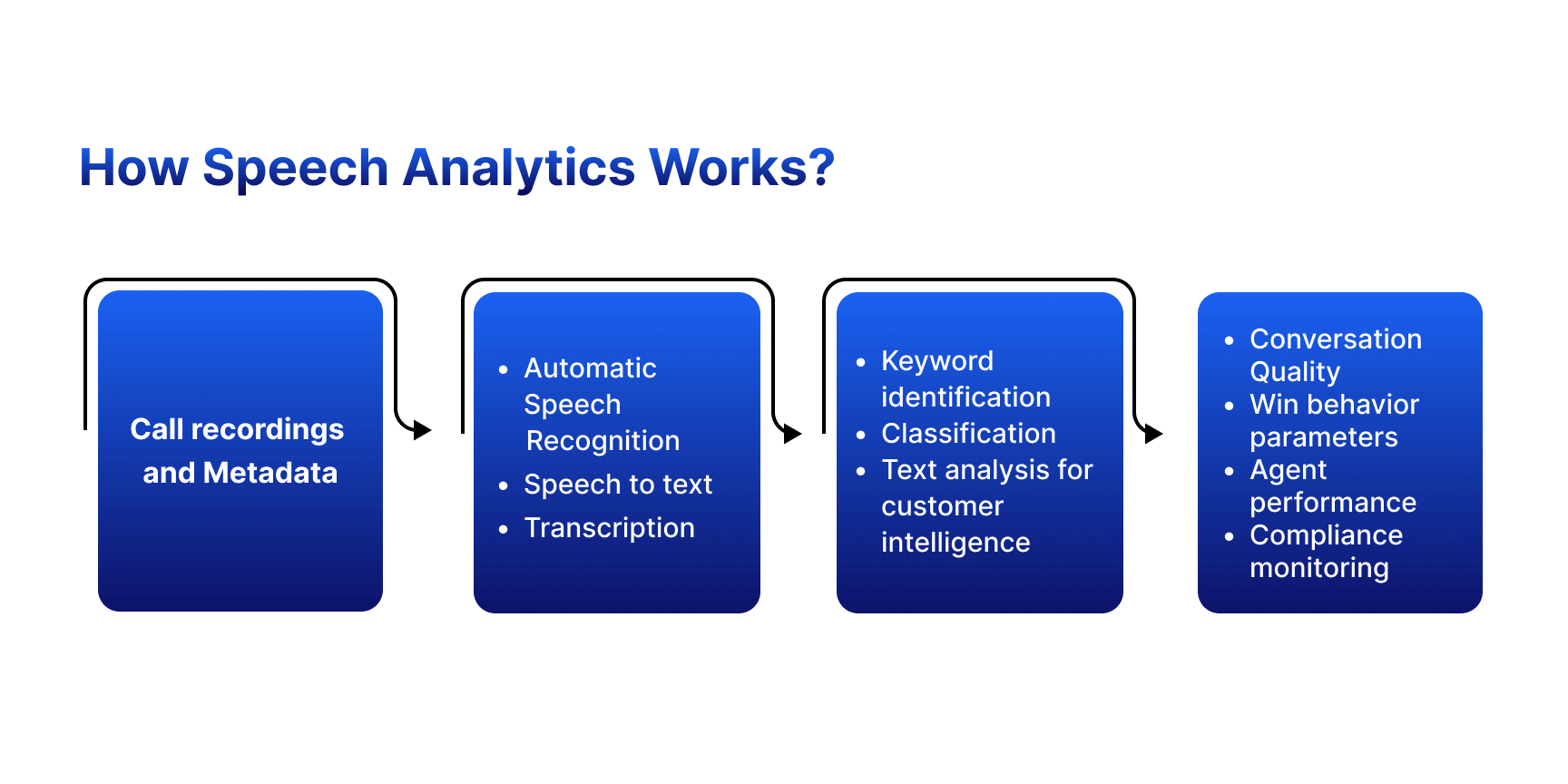
- Intelligent Parameter Identification: Utilizes advanced analytics to analyze conversation data, identifying key behaviors that lead to successful outcomes and potential customer service threats.
- Predictive Insights: The system uses machine learning to predict future customer issues and sentiments, allowing call centers to prevent customer dissatisfaction proactively.
- Strategic Advantage: This capability enables call centers to understand the deeper nuances of customer interactions, tailor services to meet customer needs more effectively, and enhance the customer journey through personalized experiences.
3. Automated Agent Coaching

- Performance-Based Coaching: By analyzing agent interactions through machine learning algorithms, Convin identifies specific coaching opportunities, focusing on areas where agents can improve their performance.
- Peer-to-Peer Learning: Incorporates examples from top performers into coaching sessions, facilitating peer learning and encouraging best practice sharing among agents.
- Developmental Benefits: This targeted approach to coaching ensures agents receive personalized feedback and training, directly impacting their ability to handle calls more effectively, enhancing customer satisfaction and reducing the need for supervisory intervention.
4. Real-Time Agent Assist
.avif)
- Live Guidance: Leveraging NLP and machine learning, Agent Assist provides agents with real-time suggestions, prompts, and alerts during customer interactions, ensuring adherence to best practices and procedural compliance.
- Dynamic Support Tools: Includes guided scripts, proactive alerts, and dynamic battle cards that adapt to the context of the conversation, aiding agents in navigating complex customer queries.
- Customer Service Excellence: This instant support improves the accuracy and quality of responses, significantly enhancing the customer experience by providing timely and practical solutions to their problems.
5. AI Feedback
- AI Feedback for Call Center Agents is a powerful tool that leverages AI and natural language processing to provide valuable insights and guidance to call center representatives. This innovative solution is designed to enhance agent performance, improve customer interactions, and ultimately boost overall call center efficiency.
- AI Feedback for Call Center Agents is a game-changing solution that empowers call center representatives to deliver exceptional service. By leveraging AI-driven insights and real-time feedback, call centers can enhance agent performance, boost customer satisfaction, and streamline operations for improved overall outcomes.
By integrating these advanced functions into its platform, Convin addresses the immediate operational needs of call centers and lays the groundwork for sustained improvement and innovation in customer service practices.
Through advanced analytics and machine learning, Convin empowers call centers to optimize their operations, improve agent performance, and deliver exceptional customer service, thereby transforming the traditional call center into a dynamic, data-driven environment.
Schedule a call with us to explore Convin's innovative solutions and take the first step toward redefining the role of call centers by utilizing advanced analytics and machine learning.
FAQs
1. Why is call center analytics important?
Call center analytics is crucial for understanding customer interactions and improving service delivery, allowing businesses to make data-driven decisions that enhance customer satisfaction and operational efficiency.
2. How artificial intelligence is transforming contact centers?
Artificial intelligence is revolutionizing contact centers by automating responses, personalizing customer interactions, and providing real-time insights, significantly improving efficiency and customer experience.
3. What are the benefits of AI in call center?
AI in call centers enhances customer service through personalized interactions, reduces operational costs by automating routine tasks, and improves decision-making with actionable insights from data analysis.
4. What are some of the ways in which call center operations can benefit from deeper analysis analytics?
Deeper analytics can uncover trends and patterns in customer behavior, optimize workforce management, and identify areas for improvement in service quality, leading to enhanced customer satisfaction and operational performance.
5. What are the three C's in call center?
The three C's in call center typically refer to Customer Satisfaction (CSAT), Cost Reduction, and Compliance, which are key metrics for measuring the success and efficiency of call center operations.
6. What is analytics call center?
An analytics call center utilizes data analysis tools and techniques to evaluate and improve call center performance, focusing on customer interactions, agent efficiency, and overall service quality.