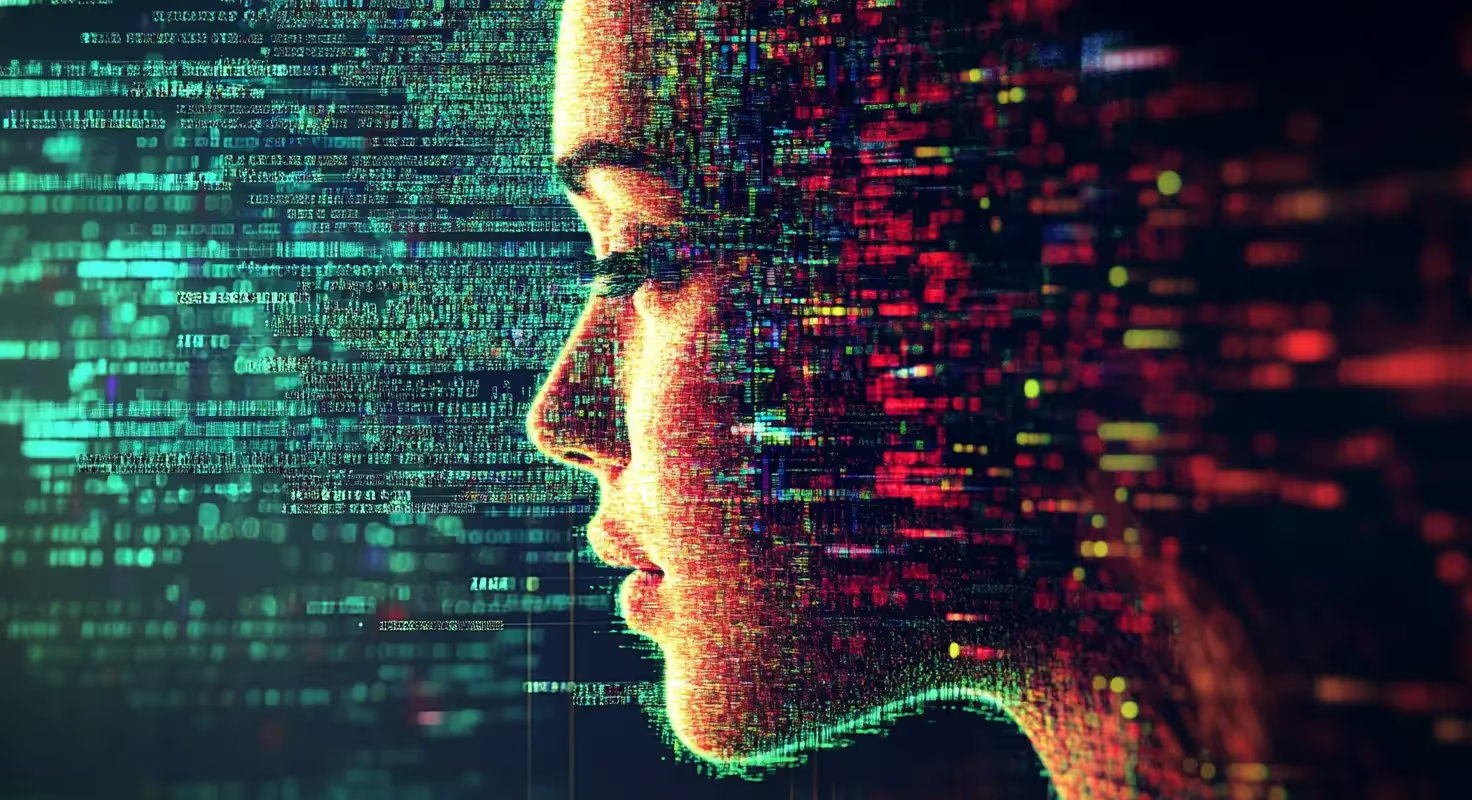
Ever wonder why some of the most ambitious businesses are reluctant to develop artificial intelligence solutions? Building a custom generative AI solution for conversation intelligence is the ultimate way to tailor technology to your business’s needs.
Developing a bespoke generative AI for conversation intelligence that perfectly aligns with your business needs sounds like a dream. Complete control over insights from sales conversations, seamless integration with your systems, and customized conversation analytics—what could go wrong?
A lot.
Imagine having complete control over the technology that powers your sales conversations, tailored to the last detail. While the self-sufficient route promises flexibility, it also brings significant challenges, from high costs to complex integrations and the constant need for updates.
Before building a custom AI tool for conversation intelligence, it’s crucial to understand why this seemingly innovative and cost-effective path could lead to more setbacks than success.
In this blog, we’ll uncover why creating your AI for conversation intelligence is much more complex than it seems and why opting for proven solutions might be the more brilliant choice.
Automate, analyze, and elevate customer interactions with Convin’s conversation intelligence.
Introduction to Conversation Intelligence and Generative AI
Conversation intelligence has revolutionized how businesses handle customer interactions, particularly within enterprise call centers.
By using advanced technologies to capture, analyze, and derive insights from customer conversations, businesses can make more informed decisions, better coach their agents, and significantly improve customer satisfaction.
The potential impact becomes even more significant when you throw generative AI into the mix.
But what exactly is conversation intelligence, and how does generative AI fit into the picture?
More importantly, why are some companies tempted to build their solutions?
What Is Conversation Intelligence?
Conversation intelligence is the technology that listens to and analyzes customer interactions—whether through calls, chats, or emails—capturing valuable insights that can improve business operations. This process involves conversation analytics, which digs deep into customer behavior, emotional cues, and conversation trends to identify key insights.
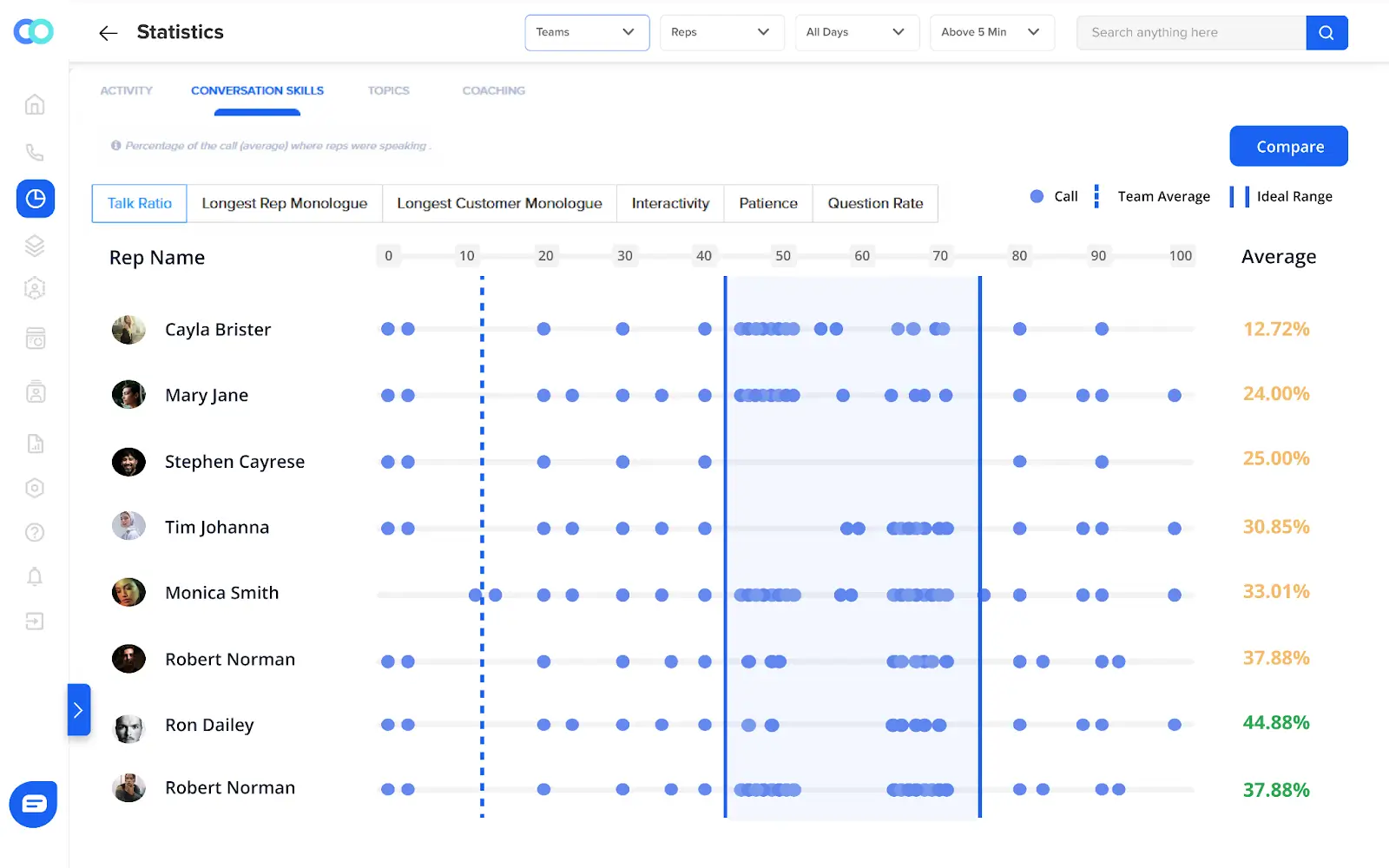
For instance, using conversation analytics software, you can:
- Track customer sentiment throughout a call
- Identify the most successful phrases in sales conversations
- Flag issues that lead to missed sales opportunities
- Analyze agent performance in real-time
- Monitor compliance issues
These insights are crucial, particularly for large organizations that rely on enterprise call center software to manage high volumes of interactions.
According to a 2022 report by McKinsey, companies using conversation intelligence tools have seen a 15–40% improvement in sales productivity, mainly due to the real-time insights they gain.
Conversation intelligence in sales enhances businesses' understanding of customer interactions. Businesses can optimize sales processes and improve conversion rates by analyzing key questions, responses, and strategies.
Conversation Intelligence and Generative AI's Role
While conversation intelligence software offers valuable post-call analysis, generative AI takes things further by actively participating in the conversation.
Imagine this: As a sales agent is on a call, the AI listens and provides real-time suggestions.
It can recommend the best follow-up question, suggest a more compelling response, or warn the agent when they’ve veered off-script—all in real-time.
Generative AI doesn’t just analyze what has been said; it predicts what should come next. In sales conversations, this can be a game-changer.
For example, the AI could suggest offering discounts or emphasizing product benefits if a customer seems uncertain. AI can also real-timely nudge agents to mention key selling points, enhancing efficiency in enterprise call center software environments.
Integrating generative AI with a conversation intelligence tool ensures agent accuracy and customer satisfaction.
According to the Demand Gen Report, AI-driven sales conversations can increase lead conversion rates by 20%, thanks to real-time guidance and improved decision-making.
Many businesses consider building in-house AI tools for complete control over their conversation intelligence and sales conversations. Custom conversation analytics software promises flexibility and seamless integration with systems like Microsoft call center software.
However, the reality involves high costs, specialized talent, and continuous updates to keep up with evolving customer interactions. While the idea is appealing, managing data, accuracy, and constant retraining can turn this into a costly, time-consuming effort. For many, opting for proven conversation intelligence tools offers a more efficient and scalable solution.
So, why would a company consider building its generative AI solution for conversation intelligence when ready-made tools are available?
It boils down to three key reasons:
- Customization and Control: A custom-built AI solution can be tailored to the company’s unique business processes and sales conversations. This allows for flexibility in designing features and functionalities that may not be available in off-the-shelf conversation intelligence tools.
- Data Privacy and Security: Building an in-house AI ensures complete control over data management for companies dealing with sensitive data, reducing the risks of sharing proprietary customer information with third-party vendors.
- Long-Term Cost Efficiency: Although the upfront costs are high, some companies believe that developing their conversation intelligence software will eliminate ongoing licensing fees, making it more cost-effective in the long run, particularly for enterprises with large volumes of customer interactions.
While building a custom generative AI solution offers control and customization, the significant costs, expertise, and time required often outweigh the benefits. For most companies, leveraging proven, ready-made conversation intelligence tools is a more efficient and cost-effective path forward.
Unlock the power of AI for smarter, faster conversation analytics
The Complexity of Developing a Homegrown Generative AI
Building your generative AI for conversation intelligence can sound like a dream. You'll have total control, customized features, and no third-party costs while boosting your sales conversations with cutting-edge technology.

But there is a condition: developing a homegrown AI solution isn’t just challenging—it’s a massive undertaking that demands serious resources, specialized skills, and continuous upkeep.
Let’s explore why this process is far more complex than it might seem.
1. High Costs and Resource Requirements
One of the first significant hurdles companies face when developing their own generative AI for conversation intelligence is the unexpectedly high costs involved. While the idea of building in-house to avoid subscription fees may seem appealing, the reality is that the initial and ongoing expenses—from infrastructure to talent—quickly add up.
But the reality is far different.
Think about it: creating an AI system that can handle and analyze enterprise call center software data requires high-end computing infrastructure. Whether you’re using managed cloud services or setting up on-premise servers, the costs for managing the sheer volume of data involved in sales conversations can skyrocket.
Then, there’s the expense of purchasing or developing conversation analytics software to process and analyze the data.
And don’t forget, the spending doesn’t stop after the initial setup. AI models require ongoing maintenance, which involves regular updates and improvements to keep up with changing customer behaviors.
Gartner found that AI projects often overrun their budgets by 30%, as companies typically underestimate the complexity of deployment and upkeep.
So, while building your system may look cheaper on paper, the hidden costs can spiral out of control.
2. The Need for Specialized Talent
Building an AI solution isn’t like your typical software project—it demands highly specialized skills. You’ll need a team of top-tier data scientists, machine learning experts, and AI developers who know the ins and outs of conversation analytics.
These professionals must design algorithms, structure customer interaction data, and fine-tune the AI models to deliver meaningful insights from your conversation intelligence tools.
The real challenge comes here: this talent is rare and expensive. Machine learning engineers and AI specialists are some of the most sought-after professionals in the tech world, and the competition is fierce.
Tech giants like Google and Amazon constantly seek these skills, making it incredibly tough—and costly—to attract and retain the talent needed for your project.
The challenge continues, even if you assemble a strong team. You’ll need them long-term to train and tweak your conversation intelligence in sales models as new trends, customer behaviors, and data emerge.
According to LinkedIn’s Emerging Jobs Report, machine learning and AI-related roles are snowballing, but finding and retaining these professionals can be a significant bottleneck for most businesses.
3. Managing Large-Scale Data and Training Models
Data is the fuel that powers your conversation intelligence software. But managing that data, especially on a large scale, is daunting.
You need massive amounts of quality data from various sales conversations and customer interactions to develop AI that can provide value.
This means handling countless hours of call recordings, chat logs, and email transcripts—often messy and unstructured data.
But it’s not just about having enough data. You need high-quality, clean data to train your AI models properly. Without it, your AI’s insights will be inaccurate or incomplete.
Then there’s the issue of keeping your models up to date. AI models need continuous training to stay relevant. As customer behaviors evolve and new trends in conversation intelligence emerge, your system must learn and adapt.
This isn’t a “set it and forget it” situation. You’ll need to retrain the AI regularly, which takes time, effort, and, once again, specialized expertise.
As data grows, so does the demand for computational power. Scaling your AI infrastructure to handle increasing data while maintaining speed and accuracy becomes a significant challenge.
In fact, McKinsey’s 2022 report highlights that only 20% of AI projects manage to scale fully due to issues with managing and processing large datasets.
Building a custom generative AI solution for conversation intelligence is complex due to high infrastructure costs, talent acquisition, data management, and model training. Businesses should significantly weigh these hurdles against benefits when ready-made tools simplify the process.
Results first, payment later
This blog is just the start.
Unlock the power of Convin’s AI with a live demo.

Technical Challenges in Building Custom AI for Conversation Analytics
Custom AI conversation analytics solutions can be challenging due to technical hurdles, including ensuring accuracy and seamless integration with call center software.

Let's break down the key technical challenges you’ll face when developing your AI for conversation intelligence.
1. Ensuring High Accuracy in Transcriptions and Analytics
In conversation intelligence, accuracy is everything. AI systems must transcribe and analyze conversations with high precision to deliver valuable insights. Any transcription error can lead to flawed analysis, impacting areas like:
- Customer sentiment detection
- Key phrase identification
- Compliance tracking
Real-world complexities in sales conversations are far from uniform.
Customers:
- Have different accents
- Use slang
- Switch between languages
- Speak in noisy environments
Training AI to handle these variations takes extensive time and vast data.
Established industry leaders in large-scale conversation analytics software providers, like Convin, have refined their algorithms over the years, boasting ideal transcription accuracy rates. Achieving this level of precision requires:
- Access to large, diverse datasets
- Continuous algorithm improvements
Creating high accuracy can be challenging for a company building AI from scratch. More nuanced conversations increase the likelihood of transcription errors. Even minor mistakes in sales conversations—like misinterpreting a key phrase—can lead to:
- Missed sales opportunities
- Inaccurate insights
2. Integrating AI with Existing Enterprise Call Center Software
A significant hurdle in developing custom AI for conversation intelligence is ensuring smooth integration with existing systems like Microsoft call center software, Salesforce, and CRMs.
The conversation intelligence tool must seamlessly connect with the following:
- Telephony systems: Capture customer calls
- CRM systems: Sync customer data
- Analytics platforms: Process and report on conversations
Each connection introduces potential compatibility issues, requiring significant customization to ensure seamless operation. If the AI doesn't integrate well, it can:
- Disrupt workflows
- Slow down operations
- Create data gaps
Custom AI integration with Microsoft call center software can significantly extend timelines, necessitating continuous updates and technical expertise to ensure smooth operation.
AI systems used for conversation intelligence in sales must stay updated on the following:
- Emerging sales techniques
- Customer objections
- Competitor mentions
Failing to update will lead to outdated suggestions and missed crucial sales opportunities. If poorly maintained, AI models can degrade over time, and poor-quality or inconsistent data can further diminish performance.
Custom AI for conversation analytics presents technical challenges like transcription accuracy, seamless integration, and continuous learning. Businesses must weigh the complexities against the reliability of conversation intelligence tools’ existence.
A 2020 report by O’Reilly found that only 22% of companies feel confident in their ability to maintain and update their AI systems effectively, leading to underperforming models.
What Makes Convin the Solution to Generative AI Challenges in Conversation Intelligence?
Navigating the technical hurdles of developing generative AI for conversation intelligence can be overwhelming, but Convin offers a solution to simplify and optimize the entire process.
Convin’s platform addresses businesses' common roadblocks—from ensuring accuracy in sales conversations to seamless integration with systems like Microsoft call center software.
Here’s how Convin tackles these challenges and provides a more brilliant, efficient alternative.
- Accuracy in Transcriptions and Analytics:
- Convin’s conversation analytics software ensures high transcription accuracy for sales conversations.
- Advanced AI models handle diverse accents, languages, and noisy environments, reducing errors.
- Tracks customer sentiment and compliance with conversation intelligence rules.
- Businesses save time using Convin’s pre-trained models, bypassing the need for years of refinement.

- Seamless Integration with Enterprise Call Center Software:
- Convin integrates effortlessly with enterprise call center software like Microsoft call center software and popular CRMs such as Salesforce.
- Sync call data from telephony systems and customer data from CRM platforms for smoother workflows.
- No operational disruptions, providing instant access to insights from conversation intelligence tools.
- Continuous Learning and Model Updates:
- Convin’s AI models automatically retrain with new data, keeping up with changes in conversation intelligence in sales and customer behavior.
- Built-in feedback loops ensure constant improvement and relevance.
- Removes the burden of manual updates, allowing businesses to focus on strategy rather than maintenance.
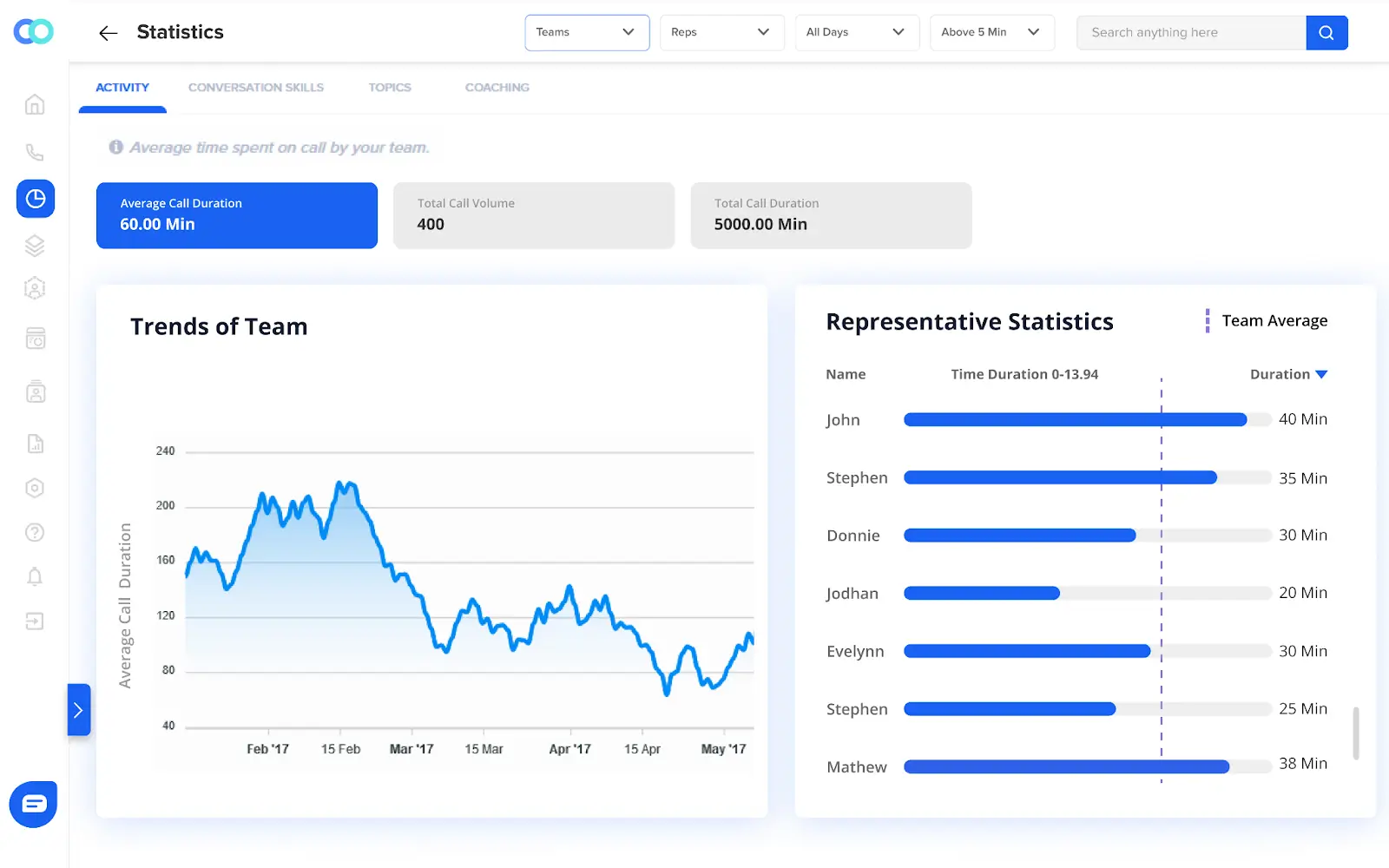
Convin addresses significant technical challenges, offering businesses a seamless, accurate, and continuously learning conversation intelligence software solution without the complexity of a custom-built system.
Record and analyze 100% of conversations with AI-driven precision.
Final Thoughts: Boost Your Call Center’s Performance with AI—Skip the Custom Build!
While building a custom generative AI for conversation intelligence offers control and customization, the high costs, long development timelines, and technical challenges make it daunting. DIY solutions often need help maintaining accuracy, integrating with systems like Microsoft call center software, and updating models.
On the other hand, established conversation intelligence platforms like Convin provide a ready-to-use solution with refined conversation analytics, seamless integration, and automatic updates. Convin’stools allow businesses to focus on enhancing sales conversations and customer interactions without the complexities of managing custom AI infrastructure.
Are you prepared to use AI to transform your call center?
Stop struggling with DIY solutions. Discover Convin's conversation intelligence tools with our interactive demo and get started to transform your sales conversations today!
Frequently Asked Questions
1. What are some of the challenges faced in generative AI?
Generative AI faces challenges like high costs, resource requirements, accuracy in handling complex sales conversations, managing large datasets, and continuous model updates in conversation intelligence tools.
2. What is the difference between generative AI and conversational AI?
Generative AI creates new content like text or images, while conversational AI focuses on understanding and responding to human conversations. It is often used in conversation analytics software and enterprise call center software.
3. What is the difference between normal AI and generative AI?
Normal AI processes data and provides insights or decisions, while generative AI creates new, original data based on input, making it useful for tasks like conversation intelligence in sales.
4. Why is conversational AI a preferred use of AI?
Conversational AI is preferred for improving customer experiences, driving insights from sales conversations, and automating responses, making it highly valuable in enterprise call center software.
5. What is the future of conversational AI?
The future of conversational AI lies in enhanced real-time interactions, seamless integration with conversation intelligence tools, and continuous learning, which will lead to smarter conversation analytics and improved customer engagement.