Imagine your business is running smoothly, but behind the scenes, a handful of employees divert payments to personal accounts, costing you millions. You don’t discover the fraud until it’s too late.
Unfortunately, this scenario is becoming more common as fraudsters use advanced methods to exploit vulnerabilities in financial systems.
But what is fraud detection? Fraud detection, identifying and preventing unauthorized activities that lead to financial loss, has become a critical priority for businesses. More than relying on traditional methods is required. Advanced tactics are evolving rapidly. Modern fraud detection systems leverage AI and machine learning to detect suspicious activities in real-time.
According to a 2023 Association of Certified Fraud Examiners (ACFE) report, businesses globally lose an estimated 5% of their annual revenues to fraud.
Below, we’ll see how fraud detection has changed and discuss the need to implement robust systems to safeguard your company from financial risks.
Tired of missing fraud signals? AI-Auditing can help—Experience Convin’s solution.
Advanced Fraud Detection with Convin's AI-Powered Solution
A leading fintech company that partnered with Convin faced a complex challenge–unable to detect fraud within its outsourced tele-collections team.
Some agents instructed customers to make payments to personal UPI accounts instead of the company’s, risking a potential loss of ₹30 lakh.
Convin's fraud detection mechanism revolutionized its ability to detect and prevent fraud by running post-call analysis. The software flagged unusual agent behavior and conversations by leveraging advanced fraud detection techniques like data analysis and AI-driven call analysis, providing early warnings and enabling quick action.
Fraud Detection Using AI and Machine Learning
Convin's fraud detection system utilized machine learning algorithms to analyze vast amounts of call data and look for anomalies. Machine learning is particularly effective in fraud detection in financial transactions, where even slight deviations in agent scripts or behaviors can signal potential fraud. By implementing this fraud detection system, the company significantly reduced its risk of fraud, preventing losses while improving operational transparency.
Moreover, fraud detection using generative AI enhanced the system's capability by simulating potential fraud scenarios and adapting to new fraudulent tactics, ensuring that no suspicious activity went undetected. This technology automatically flagged conversations where agents gave instructions that deviated from standard procedures, providing actionable insights to the company’s compliance team.
Detection and Prevention of Fraud Through Advanced Techniques
Convin’s solution goes beyond basic fraud detection techniques. Its financial fraud detection mechanism analyzed calls using predictive analytics, determining the likelihood of future fraudulent behavior. This was instrumental in cases where agents redirected payments to personal accounts, a scenario that standard fraud detection tools often miss.
Convin's approach enabled the company to:
- Identify and prevent ₹30 lakh worth of potential fraud.
- Implement a fraud detection system that monitors conversations for suspicious activities.
- Provide fraud detection using machine learning to recognize patterns and instantly offer alerts, unlike manual monitoring systems prone to delays.
- Use fraud detection software to automate compliance processes, flag risky conversations, and create a dashboard that provides insights into agents’ activities.
Fraud Detection in Financial Transactions and Payment Systems
The company's reliance on online payment fraud detection was critical, as payments were frequently made over the phone or via online methods. With payment fraud detection being a major challenge in the fintech industry, using AI-enabled tools to monitor payment instructions in real-time allowed for early identification of fraudulent patterns.
The AI flagged conversations where agents attempted to redirect payments, ensuring the company could take immediate corrective action.
Credit card and check fraud detection have become essential as financial fraud evolves. Convin’s technology was instrumental in safeguarding sensitive customer payment data and securing transactions.
Key Metrics and Results
Implementing Convin's fraud detection and prevention system gave the company robust solutions for mitigating financial fraud. Significant improvements through the real-time alert system included:
- Prevention of ₹30 lakh in fraud losses, enhancing the company's financial integrity.
- Early identification of risky behaviors allows for proactive fraud prevention strategies.
- Real-time fraud alerts detect suspicious activities during customer interactions, allowing businesses to intervene immediately to prevent fraudulent transactions from escalating.
The Rising Threat of Financial Fraud
Financial fraud is becoming an ever-growing concern for businesses globally, especially with the rapid advancement of technology. Fraudsters are no longer using simple tactics; instead, they are deploying highly sophisticated strategies that target digital and financial transactions across industries.
Financial Fraud in the Digital Age
Financial fraud is more advanced than ever, with scammers utilizing digital platforms and sophisticated tools to execute large-scale fraud. These days, it isn’t just about stealing credit card information; fraudsters exploit loopholes in financial systems, often going unnoticed for months.
Online payment fraud detection has become vital as e-commerce and digital payments surge. Fraudsters use online platforms where security measures may lag behind the latest fraud tactics.
Fraud detection techniques must adapt quickly to identify patterns and anomalies that indicate fraud. AI and fraud detection using machine learning have become essential in this fight, as they can process vast amounts of data to spot potential red flags in real-time.
According to a 2022 PwC report, 51% of businesses globally were affected by fraud, resulting in over $42 billionin financial losses.
The Growing Complexity of Fraud Tactics
Fraud schemes are becoming increasingly sophisticated, making fraud detection in financial transactions more challenging. Scammers use AI and machine learning to replicate legitimate behaviors, allowing them to slip through traditional detection systems unnoticed.
Credit card fraud detection is a key focus area. Criminals use stolen credit card data or create fake cards to make unauthorized purchases. Financial institutions now use fraud detection software to analyze transaction data and block suspicious activities in real-time.

Latest Axis Bank Trading Scam: A Case in Point
In recent news, the Axis Bank trading scam highlighted the importance of timely fraud detection. The ₹97 crore Axis Bank scam in Bengaluru involved a branch manager and three sales executives. They were arrested for illegally manipulating customers' demat accounts to conduct unauthorized stock market trades.
The fraudsters exploited these accounts for their financial gains, leading to substantial losses for the victims. The case is under investigation, with authorities tracking additional suspects involved in the more extensive network behind this operation.
Had a robust fraud detection system existed, the breach could have been identified and stopped in real-time, saving Axis Bank and its stakeholders millions. This case is a stark reminder of how financial fraud detection software can prevent such monumental losses.
Common Types of Fraud and Detection Techniques
There are several types of fraud that businesses face today, each requiring advanced fraud detection techniques:
- Payment Fraud: Includes unauthorized transactions like identity theft and credit card fraud. Payment fraud detection systems flag unusual transactions in real-time.
- Credit Card Fraud: A common financial scam involving stolen or cloned credit cards. Credit card fraud detection software uses AI to monitor spending patterns for anomalies.
- Check Fraud: Involves altering checks or forging signatures. Check fraud detection systems track irregularities in issued checks.
- Internal Fraud: Occurs within companies, often involving employees manipulating data or finances. Fraud detection and prevention software monitors internal operations for suspicious activity.
- Online Fraud: Includes phishing, identity theft, and fraudulent digital payments. Online payment fraud detection systems safeguard digital transactions.
All these require robust fraud detection systems powered by AI to protect businesses from financial losses.
The Cost of Failing to Detect Financial Fraud
The financial and reputational damage caused by undetected fraud can be staggering. Businesses that don’t have proper fraud detection systems in place risk suffering significant losses, leading to a loss of customer trust and potential legal consequences.
Payment fraud detection has become increasingly critical as businesses adopt digital payment platforms. These platforms are vulnerable to hacking and fraudulent transactions without robust fraud detection and prevention measures.
If fraud detection in financial transactions fails, the consequences can be disastrous. Fraud detection software prevents financial losses and strengthens the overall security of a company’s financial systems.
Is your fraud detection system proactive? See how Convin's AI detects and prevents fraud.
This blog is just the start.
Unlock the power of Convin’s AI with a live demo.

How Modern Fraud Detection Techniques Combat New Threats Beyond Payment Transactions
As fraud evolves, businesses must rely on advanced detection techniques to tackle new threats across various areas, not just payments. The rise of AI, machine learning, and real-time monitoring has reshaped fraud detection systems, making them more effective in identifying and preventing fraudulent activities in financial transactions and beyond.
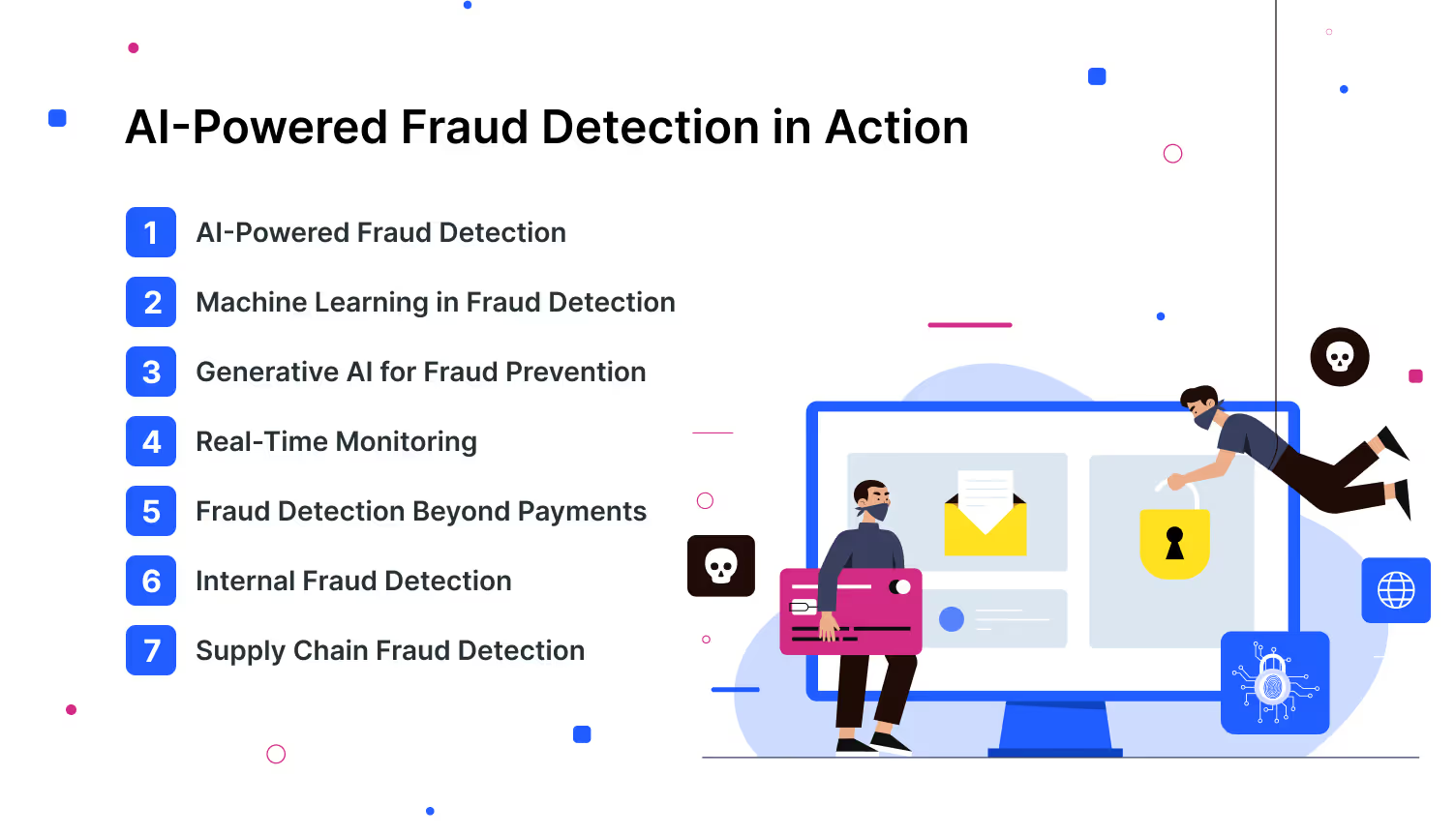
Why Businesses Must Invest in AI-Driven Fraud Detection Systems
AI-driven fraud detection and prevention systems can adapt to constantly changing fraud tactics. Traditional methods are not agile enough to detect new fraud patterns in time. However, fraud detection using machine learning can continuously learn from past transactions and refine its detection algorithms.
- Fraud detection using generative AI is a newer approach. Systems can create multiple fraud scenarios and prepare defenses accordingly. This proactive approach allows businesses to avoid fraudsters by predicting their next moves.
- For fraud detection in banking, AI can monitor many transactions, flagging any suspicious behavior in real-time. This is crucial, given the volume of transactions in the financial sector.
The main challenges of fraud detection Cost management To keep up with the ever-expanding litany of fraud techniques and typologies, businesses may need to invest more.
AI-Powered Fraud Detection and Prevention
Integrating AI in fraud detection software has transformed how businesses combat fraud. Fraud detection using machine learning enables systems to analyze massive amounts of data, learning from patterns and detecting anomalies in real-time.
- AI-driven credit card fraud detection flags unauthorized transactions instantly, preventing significant financial losses.
- Payment fraud detection has become more efficient, with AI monitoring every transaction, identifying unusual behaviors, and taking immediate action.
Two methods for building fraud models are supervised and unsupervised; both can be used to detect fraud. Unsupervised learning for fraud models Unsupervised methods use fraud modeling to detect abnormal events.
As per the Deloitte report, deepfake incidents increased 700% in fintech in 2023
Extending Fraud Detection Beyond Transactions
Fraud doesn’t stop at payments—it can infiltrate multiple aspects of a business. Fraud detection in financial transactions covers payment systems, but companies must also protect other areas, such as data security, identity protection, and supply chains.
Fraud detection techniques now focus on preventing identity theft. AI monitors user behavior for suspicious activities like unusual login attempts. Fraud detection using generative AI helps companies proactively defend against future fraud attempts by simulating different fraud scenarios.
Real-Time Monitoring for Comprehensive Fraud Prevention
Real-time fraud detection systems allow businesses to catch fraud as it happens, both in online payment fraud detection and internal operations. This capability is crucial for detecting fraudulent activities beyond financial transactions.
Check fraud detection and supply chain monitoring are becoming more critical as businesses manage various points of vulnerability. AI systems monitor transactions and interactions within the supply chain to prevent fraudulent invoicing or product manipulation.
By extending fraud detection software capabilities beyond payments, businesses can safeguard every corner of their operations from fraud.
As fraudsters evolve, businesses must stay ahead by using comprehensive fraud detection and prevention tools that safeguard their operations from financial fraud and internal threats.
Convin’s AI-powered call auditing caught a ₹30L fraud. Know how?
AI Call Auditing and Real-Time Alerts: A Winning Combo for Fraud Prevention
AI-powered call auditing has become a revolutionary tool in fraud detection and prevention, especially compared to traditional post-call analysis. While post-call analysis offers valuable insights, it often happens too late to prevent fraud.
In contrast, AI-driven real-time fraud detection techniques allow businesses to detect fraud as it unfolds, making immediate intervention possible. This shift from reactive to proactive fraud prevention has benefited financial fraud detection in sectors like banking and e-commerce.
Why Real-Time Call Auditing is Superior to Post-Call Analysis?
Real-time call auditing offers several advantages over post-call analysis:
- Immediate Fraud Detection: AI can detect suspicious activities during customer interactions, enabling financial institutions to take action before a significant loss occurs, unlike post-call analysis, which may uncover fraudulent behavior after damage.
- AI-Powered Fraud Detection Software: AI and machine learning analyze ongoing calls, detecting anomalies and patterns that indicate potential fraud, from phishing attempts to unauthorized payments.
However, post-call analysis is still valuable for identifying long-term trends and refining fraud detection techniques. The key downside is its reactive nature, which doesn’t offer the immediacy needed to stop fraud in real-time.
According to Gartner, by 2025, 30% of all enterprise call audits will be automated through AI, significantly improving fraud detection and operational efficiency.
Pros and Cons of Real-Time Call Auditing vs. Post-Call Analysis

How Machine Learning Transforms Real-Time Monitoring
Fraud detection using machine learning has revolutionized how businesses approach fraud detection and prevention. Machine learning algorithms can analyze vast amounts of transactional data and recognize patterns that human analysts might miss. Fraud detection systems using these technologies can adapt to new fraud techniques, constantly evolving to provide better protection.
Similarly, fraud detection using generative AI has brought a new layer of sophistication. Generative AI can predict fraudulent activities before they happen by creating simulations of possible fraud scenarios. This proactive approach enhances the detection and prevention of fraud, especially in complex financial transactions.
Fraud Detection Techniques: How They Work
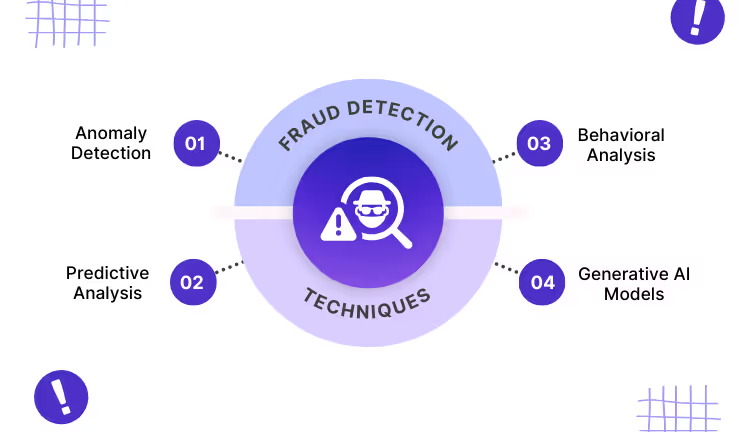
Several fraud detection techniques are employed by fraud detection and prevention systems. These include:
- Anomaly Detection: This technique identifies unusual behavior in data patterns.
For example, a sudden surge in financial transactions from a normally inactive account would trigger an alert.
- Predictive Analysis: Fraud detection systems leverage historical data to predict future fraudulent activities, especially online payment fraud detection.
- Behavioral Analysis: AI-driven systems can detect deviations in user behavior, such as accessing accounts from unfamiliar devices or IP addresses, alerting businesses of possible fraud attempts.
- Generative AI Models: These AI models simulate possible fraud tactics to help businesses build robust preventive measures.
Fraud Detection in Banking: A Critical Need
Banking fraud is one of the most damaging forms of financial fraud. Banks need robust fraud detection systems, whether credit card fraud or check fraud detection.
The financial impact of banking fraud can be staggering. In India alone, the Reserve Bank of India reported that banks lost over ₹1.85 lakh crore due to fraud in the fiscal year 2020-2021. With the rise of digital banking, the demand for fraud detection in banking has never been higher.
Key Components of Financial Fraud Detection Software
- Machine Learning Algorithms: These algorithms analyze historical and real-time data to detect anomalies in banking transactions.
- Fraud Detection System: A comprehensive system that monitors various points of vulnerability in financial institutions, from payment processing to customer account access.
- Real-Time Alerts: Immediate notifications of suspicious activity allow financial institutions to take action before any loss occurs.
Fraud is not limited to banking; every industry can benefit from AI-powered fraud detection software. In retail, online payment fraud detection systems can monitor purchases, flagging unusual buying patterns.
In healthcare, fraud detection systems can identify fraudulent insurance claims by analyzing billing patterns. AI allows these industries to implement scalable fraud detection systems, minimizing the risk of fraud across different sectors.
Ultimately, fraud detection using AI, machine learning, and real-time monitoring represents the future of fraud prevention. By integrating these technologies into existing systems, businesses across industries can significantly reduce their risk of falling victim to fraud.
Fraud threats are evolving—so should your defenses. Learn how with Convin’s AI.
Secure Your Business with AI-Driven Fraud Detection
Convin’s AI-powered fraud detection software equips businesses with the tools to detect and prevent fraud in real-time. With advanced fraud detection techniques like machine learning and call auditing, Convin enables companies to monitor financial transactions, customer interactions, and internal processes, ensuring they catch fraud early.
AI-driven systems adapt to new fraud patterns, making them essential for detecting credit card fraud and payment fraud and preventing financial crimes before they escalate.
As fraud tactics grow more sophisticated, relying on manual or outdated methods isn’t enough. Convin’s fraud detection and prevention solutions ensure businesses stay ahead of threats with real-time monitoring and proactive alerts.
Ready to protect your business from evolving fraud? How can Convin’s AI systems help prevent fraud before it impacts your bottom line? Experience our free demo to see how we can safeguard your financial future!
Frequently Asked Questions
1. What industries benefit the most from AI-driven fraud detection systems?
AI-driven fraud detection is especially beneficial for industries like banking, e-commerce, insurance, healthcare, and fintech, where financial transactions and sensitive data are at high risk.
2. How does AI help in detecting insider fraud?
AI monitors employee behavior in real-time, flagging unusual patterns such as unauthorized data access or suspicious transaction activities, which helps detect insider fraud.
3. Can fraud detection software integrate with existing payment systems?
Yes, most fraud detection software is designed to integrate seamlessly with payment gateways, CRMs, and other financial transaction systems for real-time monitoring.
4. How accurate is AI in preventing false positives in fraud detection?
Machine learning algorithms improve accuracy over time by learning from data, reducing false positives as they adapt to legitimate transaction patterns.
5. What is the cost of implementing AI-powered fraud detection software?
Costs vary depending on the software’s complexity, scalability, and the business's specific needs. Initial setup can be high, but the ROI often outweighs the upfront investment due to fraud prevention.