
Despite a decade invested in digital transformation, financial institutions face significant challenges in a fiercely competitive landscape. These challenges manifest as direct threats from competitors and high customer churn rates.
Recent discussions with several BFSI organizations revealed two key insights: customer expectations are evolving rapidly, and the pressure on established financial institutions intensifies as customers become more accustomed to new technologies and market players. For instance, generative AI contact center customers now demand faster and more personalized service, expecting accurate resolutions within a single interaction.
Compounding these issues is a prevalent industry problem: misselling. This non-compliant behavior remains common and needs to be addressed. Regulatory bodies may penalize institutions caught in such practices, but these actions often come too late to repair the damage to the brand's reputation.
Thus, in addition to technological advancements and competitive pressures, misselling and non-compliance further increase customer churn in the financial sector.
What Has Changed for Financial Institutions in the Last Few Years?
In recent years, the financial sector has undergone significant transformations, driven primarily by advancements in artificial intelligence and machine learning. The introduction and rapid adoption of ChatGPT and other large language models (LLMs) have catalyzed a shift towards AI-driven solutions within banks and financial institutions.
According to a Gartner report, 45% of executive leaders acknowledge that the publicity around ChatGPT has motivated them to explore AI applications within their companies.
The emergence of Generative AI to enable BFSI to address long-standing challenges has been particularly impactful.
Fintech companies investing in Gen AI now produce deeper customer insights, utilizing advanced LLMs to analyze customer interactions and transactional data. These models extract actionable insights, helping organizations make informed decisions and enhance customer service.
A Statista report indicates that in 2023, two-thirds of companies in the financial sector invested in Gen AI for data analytics, recognizing it as the most profitable AI application in financial services.

The integration of machine learning, natural language processing, and speech analytics has allowed financial institutions in the contact center to access and analyze customer data with unprecedented granularity.
This transformation has improved operational efficiency and enabled more personalized and accurate customer interactions, reducing churn and enhancing customer satisfaction. Financial institutions now leverage these technologies to predict customer needs, detect fraudulent activities, and ensure compliance, fundamentally changing how they operate and compete in the market.
Let’s throw some light on how LLM extracts and analyzes BFSI call center data.
Discover how AI drives the BFSI sector forward.
How do Convin LLM and Generative AI work?
Convin's Generative AI leverages a large language model (LLM) to create insightful text, visual, and audio content tailored for the BFSI sector. By analyzing customer and transactional data, Convin LLM helps financial institutions extract granular customer insights, enhancing their operational efficiency and AI for customer service.
The Process Behind Convin LLM–A step-by-step breakdown:

1. Identify Goal
The process begins by identifying the specific use case or goal that needs to be addressed. Based on this, relevant data sources are selected.
2. Data Collection and Preprocessing
Convin collects proprietary and open-source domain-specific data to create an extensive training set. This data undergoes cleaning and preprocessing to remove noise and ensure high quality.
Quality filtering can help eliminate low-quality or spammy content, deduplication techniques remove duplicate entries, and all Personally Identifiable Information (PII) is removed.
The text is then broken down into smaller units called tokens through tokenization, allowing the model to process the text effectively.
3. Pre-training
The cleaned and processed dataset is used to pre-train the Convin LLM. This pre-training phase helps the model develop a deep understanding of the language patterns and adapt to various languages and contexts.
4. Fine-tuning
Fine-tuning is an iterative process where the model is trained on task-specific labeled data. This step adjusts the model's parameters to predict labels accurately, guided by its pre-existing knowledge from pre-training.
Supervised fine-tuning enhances the model's performance on specific tasks, making it highly effective and efficient for customizing LLMs for BFSI applications.
By implementing Convin LLM, BFSI contact centers can harness the power of advanced language models and Generative AI for the transformation of their operations, enhance customer experience, and maintain a competitive edge in the market.
Results first, payment later
How are BFSI Call Centers Leveraging Generative AI?
Financial sector contact centers increasingly leverage Generative AI for gain actionable insights and provide automated feedback that enhances business outcomes.
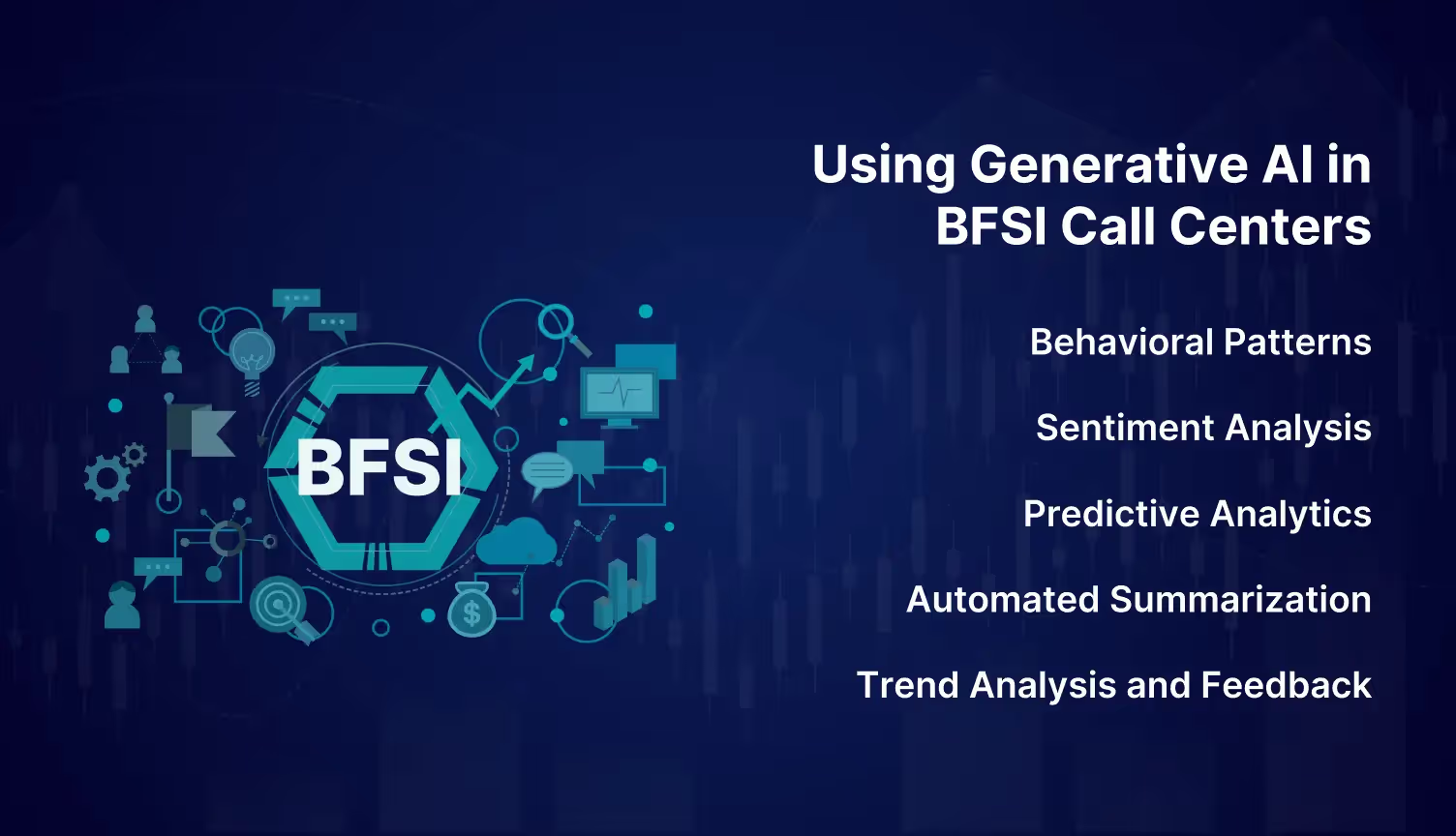
Here are some key techniques and applications:
- Behavioral Patterns
Generative AI extracts and analyzes customer behavior patterns, preferences, needs, and pain points. These behavioral insights enable personalized servicing and interactions, ensuring customers feel valued and understood.
- Sentiment Analysis
Generative AI analyzes customer interactions' tone, sentiment, and emotional aspects. By understanding these elements, the call center agents can tailor their responses, handling some conversations with empathy while maintaining assertiveness in others. This leads to improved customer satisfaction and more effective problem resolution.
- Predictive Analytics
Predictive analytics, such as win-loss analysis and lead interest assessment, allow financial services in the contact center to plan strategically. By focusing time, effort, and resources on valuable leads and customers, they can lower acquisition costs and foster repeat business, ultimately enhancing their competitive edge.
- Automated Summarization
Generative AI can automatically summarize customer interactions, saving agents significant time and ensuring no critical details are overlooked. It can also generate automated responses to common queries, provide summaries of previous complaints and resolutions, and suggest product recommendations. This automation improves efficiency and consistency in customer service.
- Trend Analysis and Feedback
Financial call centers benefit from Generative AI's ability to perform trend analysis, feedback analysis, and anomaly detection. These insights help identify emerging patterns and potential issues, allowing for proactive adjustments and continuous improvement in service quality.
By integrating these advanced AI techniques, financial sector contact centers can enhance their understanding of trends and customer behaviors, optimize operations, and deliver superior customer experiences. Convin's Generative AI from Convin and LLM technologies are at the forefront of this transformation, driving significant improvements in business outcomes for BFSI institutions.
Applications of Convin LLM in BFSI Call Centers
Convin LLM and Generative AI are equipped to perform various tasks that generate valuable customer intelligence for contact centers in BFSI:
- Enhanced Customer Support: Provides accurate and timely responses to customer queries, improving overall customer satisfaction.
- Fraud Detection: Analyzes patterns and behaviors to detect fraudulent activities, ensuring compliance and security.
- Predictive Analytics: Uses historical data to predict customer needs and behaviors, enabling proactive customer service.
- Sentiment Analysis: Gauges customer emotions in real-time, helping agents to adjust their approach for better interactions.

- Automated Summarization: Creates concise summaries of customer interactions, streamlining knowledge transfer and follow-up actions.
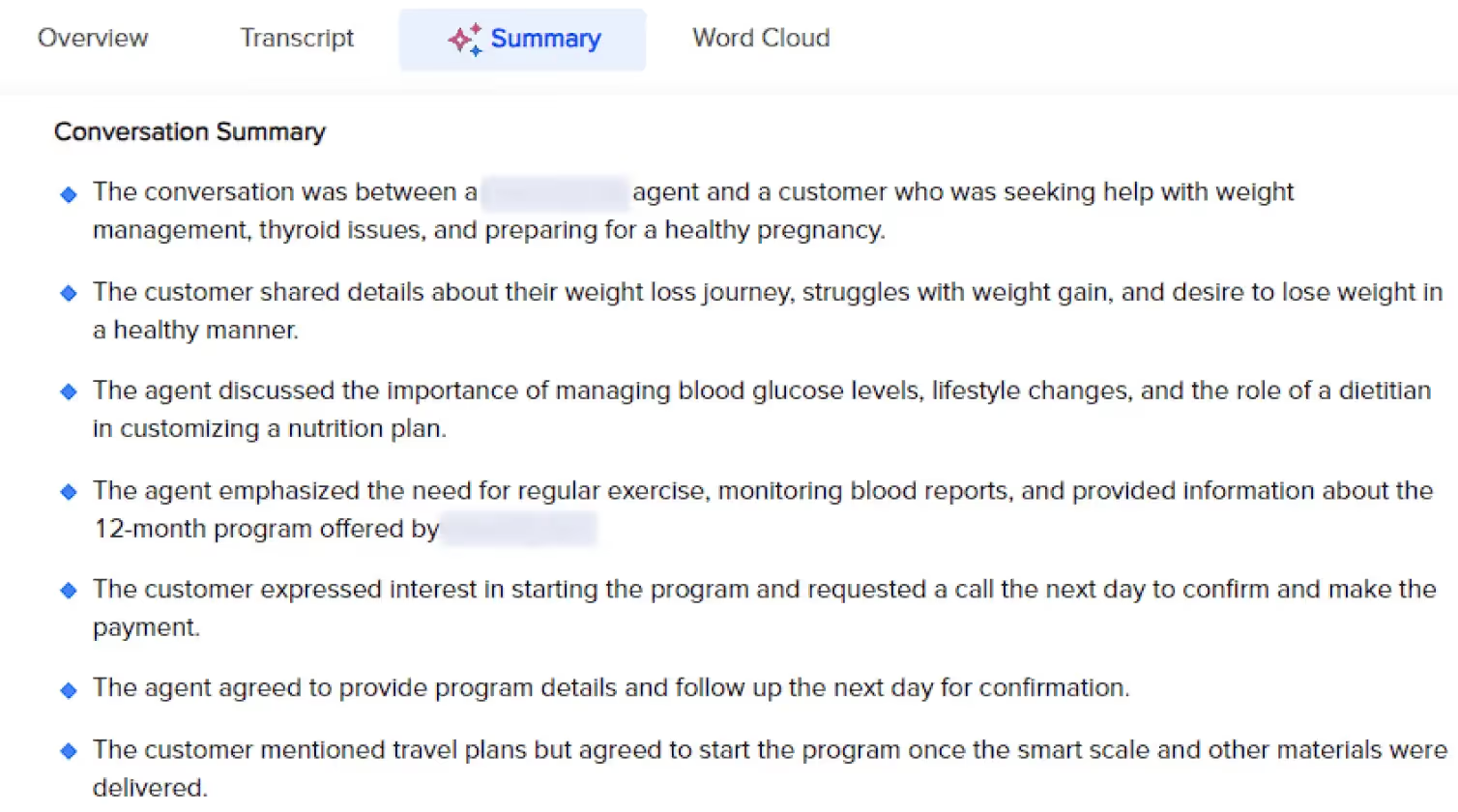
- Lead Scoring: Categorizes leads based on their interaction content, prioritizing follow-ups and optimizing sales efforts.
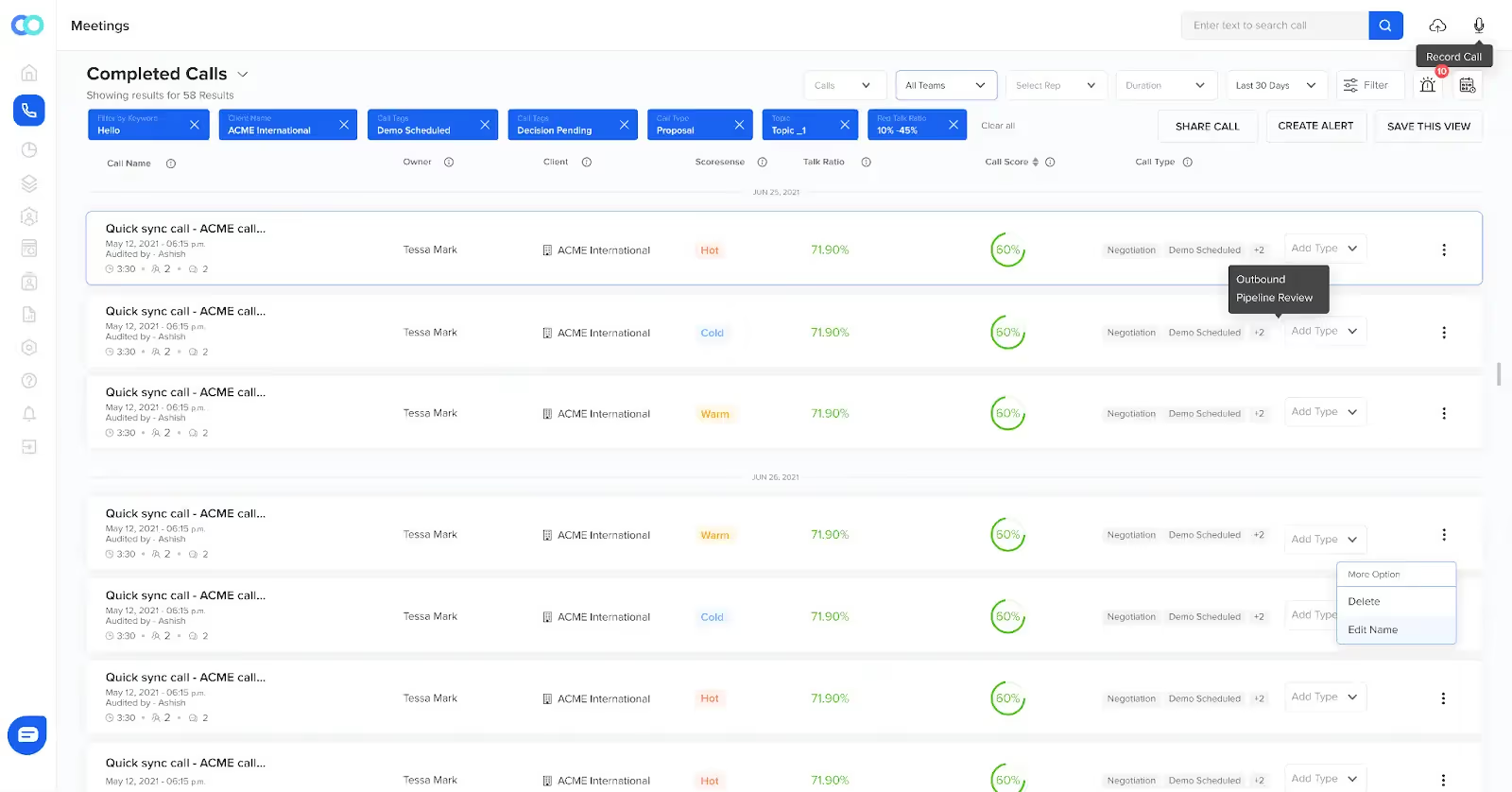
Call center leaders in the financial sectors have witnessed improved follow-ups, strategic investment plans, better financial services, increased first-call resolutions, and a lowering of misselling rates by at least 31%. Moreover, collection rates have increased by 17%, and sales business has improved by 21% in the financial sector call centers.
Watch out how AI boosts operational efficiency in financial services.
So, What's the Problem with Implementation?
While LLM models have significantly enhanced the financial industry's capabilities, they also introduce uncertainties, particularly in handling sensitive customer information.
Common concerns include ensuring data security, seamless integration with complex IT infrastructures and legacy systems, regulatory compliance, and the scalability of customer intelligence platforms.
How does Convin LLM solve data privacy and security issues?
- Data processing on a robust in-house infrastructure ensures complete control over sensitive information.
- Advanced data protection measures like industry-standard encryption, strict access controls, and regular security audits safeguard data.
- Proactive measures like input filtering, output sanitization, and data anonymization prevent data exposure or misuse.
Discussions around risk, ethics, privacy, and security will always persist in the financial services sector. The transformative impact of LLM models and generative AI technology is undeniable.
According to a Gartner survey 2023, 68% of executive leaders believe the benefits of generative AI will outweigh its associated risks. The primary focus is shifting towards enhancing the customer experience, surpassing cost optimization as the most significant advantage for global companies.
Future of AI in Financial Services Call Centers
As we look towards the future, the integration of Gen AI in financial services call centers is set to bring even more transformative changes.
Predictive Analytics
Predictive analytics will enable proactive customer service and personalized experiences, enhancing customer satisfaction and loyalty.
Advanced Natural Language Processing
Advanced natural language processing (NLP) will achieve human-like interactions and offer multilingual support, making customer interactions smoother and more inclusive.
AI-Driven Fraud Detection
AI-driven fraud detection systems will enhance security with real-time alerts and better fraud prevention.
Continuous Learning and Adaptation
Continuous learning and adaptation will allow AI models to self-improve and scale with growing data volumes and evolving business needs.
Integration with Emerging Technologies
Integrating emerging technologies like blockchain and IoT will further enhance security, transparency, and real-time management in financial services.
By staying ahead of these trends, financial institutions can continue delivering exceptional service and maintaining a competitive edge in an increasingly AI-driven landscape.
Ethical Considerations in AI Adoption
The rapid adoption of AI in financial services call centers brings forth ethical considerations that warrant attention. As AI systems become integral to customer interactions and decision-making processes, ensuring ethical practices is crucial for maintaining trust and transparency.
Fairness and Bias Mitigation
AI algorithms can inadvertently perpetuate biases in training data, leading to unfair outcomes for specific demographic groups. Financial institutions must implement measures to identify and mitigate bias in AI models. This includes using diverse datasets, regularly auditing algorithms, and implementing fairness-aware algorithms to ensure equitable treatment of all customers.
Transparency and Explainability
Customers and regulators alike demand transparency in AI-driven decisions. Financial institutions should prioritize developing AI systems that provide explanations for decisions made. Techniques such as model interpretability and explainable AI (XAI) can help customers understand how AI arrives at conclusions, thereby building trust and credibility with them.
Data Privacy and Security
The use of AI involves processing vast amounts of sensitive customer data. Financial institutions must adhere to stringent data privacy regulations (e.g., GDPR, CCPA) and implement robust security measures to protect customer information from unauthorized access and breaches. AI solutions should incorporate privacy-preserving techniques such as data anonymization and encryption to safeguard customer privacy.
Accountability and Governance
Establishing clear accountability for AI decisions and actions is essential. Financial institutions should define roles and responsibilities for AI oversight and governance. This includes creating policies and frameworks for ethical AI usage, conducting regular audits, and ensuring compliance with regulatory standards. Implementing ethical guidelines and codes of conduct for AI practitioners further reinforces responsible AI deployment.
Human-AI Collaboration
While AI enhances operational efficiency, human oversight remains indispensable. Financial services call centers should foster a culture of human-AI collaboration, where AI augments human capabilities rather than replacing them. Investing in employee training and upskilling to work alongside AI systems ensures that human judgment and empathy continue to play a pivotal role in customer interactions.
Public Trust and Communication
Building public trust in AI requires proactive communication and education. Financial institutions should engage with stakeholders—including customers, employees, and regulators—to explain AI capabilities, benefits, and ethical safeguards in place. Transparency about data usage, AI applications, and the measures taken to address moral concerns fosters trust and promotes responsible AI adoption.
Financial services call centers must prioritize ethical considerations in AI adoption to fully utilize AI's potential, build trust, and navigate the ethical landscape responsibly.
A New Era for Financial Services Call Centers
The growth potential for Gen AI-driven call centers is immense. There is a rising demand and adoption of AI-driven customer intelligence platforms, emphasizing the importance of integrating generative AI in your operations. Embrace the Gen AI revolution because AI is set to become an integral part of everything in the future.
Talk to our experts if you want to know more about Convin Contact Center LLM.
Frequently Asked Questions
1. How is GenAI Used in Financial Services?
Generative AI is used in financial services to enhance customer interactions, automate routine tasks, analyze large datasets for insights, and improve decision-making processes. It helps in fraud detection, risk management, personalized customer service, and financial forecasting.
2. What are the use cases for GenAI in fintech?
GenAI use cases in fintech include:
- Fraud Detection: Identifying suspicious activities and preventing fraud.
- Customer Service: Automating responses and providing personalized assistance.
- Risk Management: Analyzing data to predict and mitigate risks.
- Investment Analysis: Generating insights and recommendations for investments.
- Compliance: Ensuring adherence to regulations through automated monitoring.
3. What is GenAI for accounting, finance, and banking specializations?
In accounting, finance, and banking, GenAI specializes in automating data entry, reconciling accounts, generating financial reports, forecasting market trends, and ensuring compliance with financial regulations. It helps streamline operations, reduce errors, and provide actionable insights.
4. How does technology improve financial services?
Technology improves financial services by:
- Efficiency: Automating repetitive tasks to save time and reduce costs.
- Accuracy: Enhancing data analysis and reducing human error.
- Customer Experience: Offering personalized services and faster response times.
- Security: Strengthening fraud detection and cybersecurity measures.
- Accessibility: Making financial services more accessible to a wider audience through digital platforms.