In the fast-paced digital era, understanding human emotions and opinions is paramount for businesses striving to enhance customer satisfaction, tailor products and services, and make informed decisions.Â
Sentiment analysis, a process of gauging sentiments expressed in textual data, has emerged as a vital tool. With the advent of Generation AI (Gen AI), leveraging advanced AI techniques has redefined sentiment analysis, making it more scalable, accurate, and personalized than ever before.Â
Using AI and machine learning, sentiment analysis automates the process of analyzing vast textual data and identifying customers' positive, negative, and neutral sentiments, thereby enhancing the customer experience.Â
Let's explore how Gen AI is reshaping sentiment analysis across various domains.
Understand and translate natural interactions using Gen AI in sentiment analysis.
What is Sentiment Analysis?
Sentiment analysis, also known as opinion mining, is a process that involves using natural language processing (NLP) and machine learning techniques to analyze and interpret textual data to determine the sentiment expressed within it.Â
The primary goal of sentiment analysis is to understand whether the sentiment conveyed in the text is positive, negative, or neutral. This analysis helps businesses and organizations gain insights into public opinion, customer feedback, market trends, and brand reputation, enabling them to make informed decisions and take appropriate actions.

How can Sentiment Analysis be Classified?
Sentiment analysis encompasses various types, including polarity-based analysis for classifying sentiments as positive, negative, or neutral and aspect-based analysis for discerning sentiments related to specific attributes or aspects within the text.
Polarity-Based: Polarity-based sentiment analysis is one of the most common approaches, where the text is classified into positive, negative, or neutral sentiments. This method focuses on determining the overall sentiment expressed in the text without delving into specific aspects or emotions.Â
For instance, in customer reviews, polarity-based analysis can quickly identify whether the review praises the product (positive), criticizes it (negative), or provides a neutral opinion.
Utilizing sentiment analysis tools powered by advanced analytics and machine learning algorithms enables scalability in processing large volumes of textual data in real time, facilitating predictive analytics to anticipate customer reactions and personalize responses.
Aspect-Based: Aspect-based sentiment analysis goes beyond overall sentiment classification and identifies sentiments related to specific aspects or attributes within the text. For example, a product review can determine sentiments towards performance, design, usability, etc.Â
This granular analysis provides deeper insights into customer preferences and pain points, enabling businesses to tailor their products and services accordingly.
By integrating sentiment analysis with conversational AI and speech recognition technologies, companies can automate aspect-based sentiment analysis in call center analytics, allowing personalized responses to customer queries and issues.
Emotion Detection: Emotion detection focuses on recognizing and categorizing emotions expressed in the text, such as joy, anger, sadness, etc. Unlike polarity-based analysis, which merely classifies sentiments as positive, negative, or neutral, emotion detection provides a more nuanced understanding of the underlying emotions driving the sentiment.Â
This can be particularly valuable in understanding customer satisfaction levels or identifying potential escalations in customer interactions.
Employing adaptive learning techniques in sentiment analysis models enables continuous improvement and refinement based on new data, enhancing accuracy in emotion detection and providing more personalized customer support through generative AI.
Intent Analysis: Intent analysis aims to determine the intention behind the expressed sentiment in the text. It goes beyond identifying whether the sentiment is positive or negative and delves into understanding the underlying purpose or goal of the communication.Â
For instance, in social media comments, intent analysis can distinguish between genuine feedback, sarcasm, complaints, or inquiries, providing businesses with actionable insights for tailored responses.
Incorporating sentiment analysis into online platforms facilitates real-time monitoring of customer sentiments, allowing businesses to swiftly address issues, mitigate risks, and capitalize on opportunities.
These types of sentiment analysis techniques offer varied granularity and insights into textual data, empowering businesses across industries to make data-driven decisions, enhance customer experiences, and drive business growth.
This blog is just the start.
Unlock the power of Convin’s AI with a live demo.

What is the Process of Sentiment Analysis Using AI?
AI-based sentiment analysis uses natural language processing (NLP) and machine learning algorithms to analyze textual data and determine its sentiment.Â
Here's a brief overview of the process:
- Data Collection: Textual data is collected from various sources, such as social media, customer reviews, surveys, etc.
- Preprocessing: The collected text data undergoes preprocessing, which involves tasks like removing irrelevant information, tokenization (breaking text into individual words or phrases), and handling stopwords (commonly occurring words like "the," "and," etc.).
- Feature Extraction: Relevant features are extracted from the preprocessed text data. These features could include words, phrases, or even syntactic patterns indicative of sentiment.
- Sentiment Classification: Machine learning models are trained on labeled datasets to classify text into different sentiment categories, such as positive, negative, or neutral. During training, the models learn to recognize patterns in the extracted features associated with specific sentiments.
- Evaluation and Iteration: The performance of the sentiment analysis model is evaluated using metrics like accuracy, precision, recall, etc. The model is then iteratively refined and improved based on feedback and new data to enhance its accuracy and generalization capabilities.
- Deployment: Once trained and evaluated, the sentiment analysis model is deployed to analyze new textual data in real-time. It processes incoming text, predicts its associated sentiment, and provides actionable insights for decision-making.
In general, AI-based sentiment analysis automates the process of understanding and categorizing sentiments expressed in textual data, enabling businesses to gain valuable insights into customer opinions, market trends, and brand reputation.
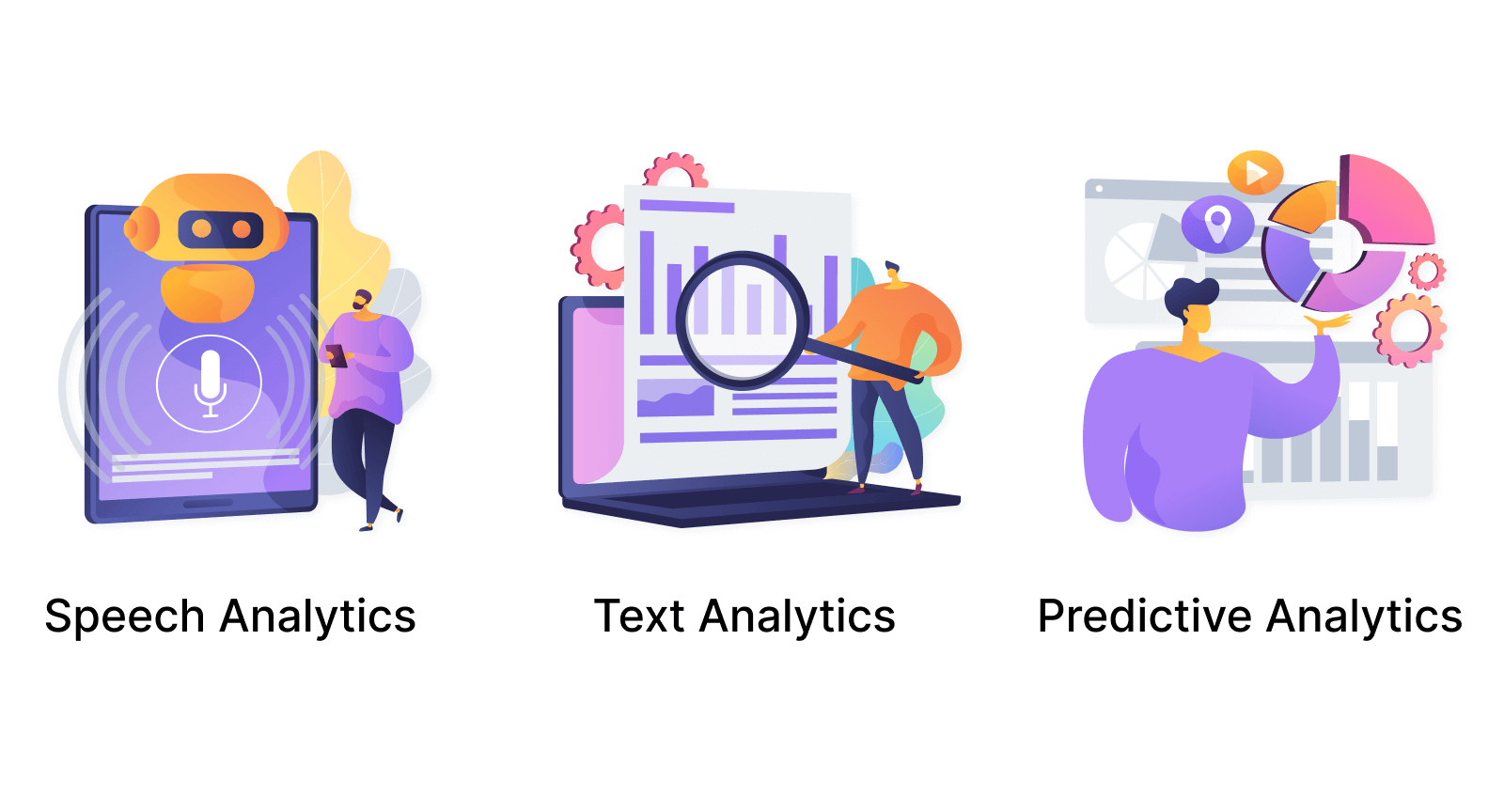
Key Applications of AI-based Sentiment Analysis
The primary uses of AI-based sentiment analysis include:
- Customer Feedback Analysis: Businesses use sentiment analysis to analyze customer reviews, feedback, and social media posts to understand customer sentiment towards products and services. This helps in identifying areas for improvement and enhancing customer satisfaction.
- Brand Monitoring: Sentiment analysis tracks brand mentions online and evaluates public perception. Businesses can effectively manage their brand reputation and promptly respond to negative sentiment by monitoring sentiments expressed on social media, news articles, and forums.
- Market Research: Sentiment analysis enables organizations to analyze market trends, competitor sentiment, and consumer preferences. By understanding sentiment toward products, brands, and market events, businesses can make data-driven decisions and gain a competitive edge.
- Product Development: Businesses leverage sentiment analysis to gather insights from user feedback and reviews to improve existing products or develop new ones tailored to customer needs and preferences. This helps in enhancing product quality and driving innovation.
- Risk Management: Sentiment analysis is used to identify potential risks and issues by monitoring sentiments expressed by stakeholders, investors, or employees. Organizations can anticipate and mitigate risks by analyzing sentiment in financial news, social media, and market data.
- Customer Support: Sentiment analysis is integrated into customer support systems to analyze customer queries and real-time feedback. By understanding the sentiment behind customer interactions, businesses can provide personalized responses, address issues promptly, and enhance the overall customer experience.
These applications demonstrate how AI-based sentiment analysis can be applied across various domains to gain valuable insights, make informed decisions, and drive business success.

Benefits of AI-based Sentiment Analysis
AI-based sentiment analysis is manifold and contributes significantly to various aspects of business operations.Â
Here are some key advantages:
- Informed Decision Making: Provides data-driven insights for strategic decisions.
- Enhanced Customer Experience: Enables proactive responses to customer needs and feedback.
- Practical Reputation Management: Identifies and addresses negative sentiment promptly.
- Cost and Time Efficiency: Automates analysis processes, saving time and resources.
- Competitive Advantage: Keeps businesses ahead by understanding market trends and competitor sentiment.
- Personalized Marketing: Tailors content and offerings to individual preferences.
- Risk Mitigation: Identifies potential risks and compliance issues, facilitating proactive management.
Thus, AI-based sentiment analysis empowers businesses to optimize operations, improve customer satisfaction, and stay competitive.
Uses of AI-powered Sentiment Analysis Across a Range of Industries
AI-enabled sentiment analysis finds application across diverse industry verticals:
- Retail: Analyzing customer reviews to improve product offerings and enhance shopping experiences.
- Finance: Monitoring market sentiment to inform investment decisions and manage risks.
- Healthcare: Extracting patient feedback to enhance care quality and patient satisfaction.
- Hospitality: Analyzing guest feedback to improve service standards and guest experiences.
- Telecommunications: Monitoring customer sentiment to enhance service offerings and address issues promptly.
- E-commerce: Tailoring marketing strategies based on sentiment analysis of customer interactions and reviews.
- Automotive: Analyzing sentiment in customer feedback to enhance product design and features.
These use cases demonstrate the versatility of AI-enabled sentiment analysis in driving improvements across various industries, ultimately leading to better customer experiences and business outcomes.
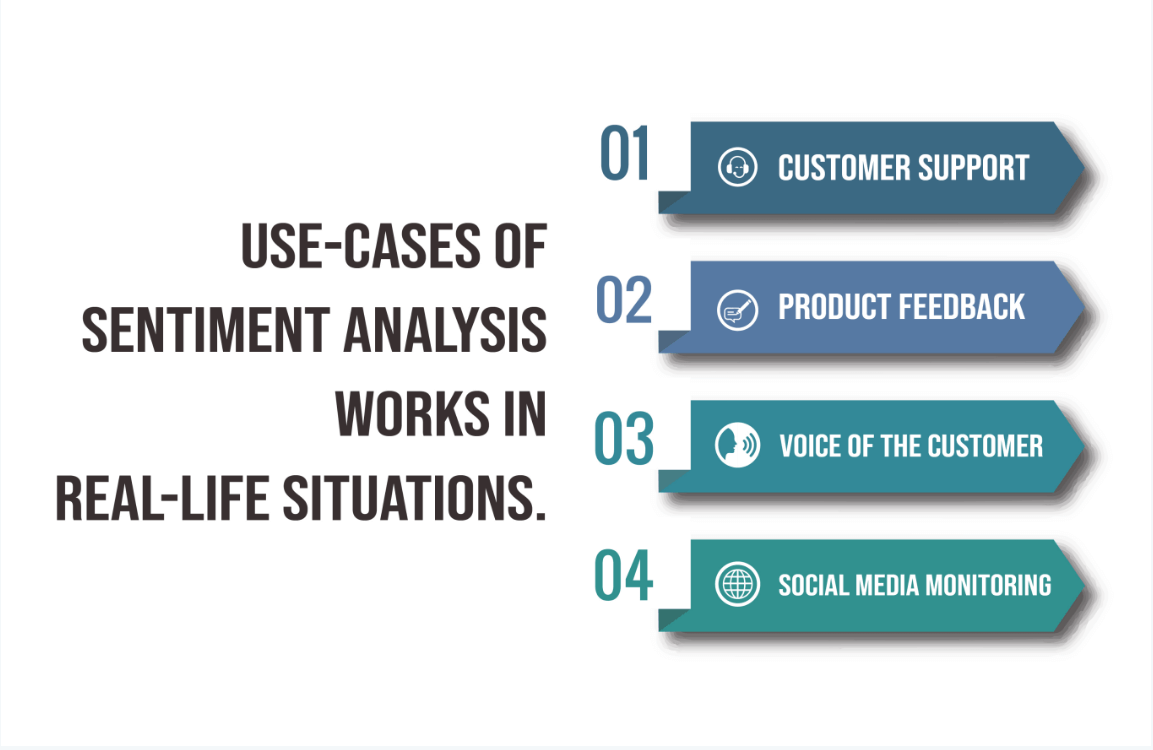
Unleashing the Power of AI-Enabled Sentiment Analysis
In conclusion, AI-enabled sentiment analysis has emerged as a transformative tool across industries, offering invaluable insights into customer sentiment, market trends, and brand perception.Â
By harnessing advanced analytics and machine learning algorithms, businesses can make informed decisions, enhance customer experiences, and gain a competitive edge in today's dynamic landscape.
Convin plays a crucial role in Gen AI used in sentiment analysis by providing advanced conversational AI solutions that enhance the effectiveness and efficiency of sentiment analysis processes.Â
Through sophisticated natural language processing (NLP) algorithms and machine learning techniques, Convin's conversational AI technology can accurately interpret and analyze textual data to discern underlying sentiments.
Convin is responsible for:
- Natural Language Understanding (NLU): Convin's NLU capabilities enable it to comprehend the nuances of human language, including colloquialisms, slang, and context-specific expressions. This allows it to interpret sentiments expressed in text data accurately.
- Sentiment Analysis Integration: Convin integrates sentiment analysis functionalities into its conversational AI platforms, enabling real-time analysis of text inputs from users. By understanding the sentiments conveyed in user interactions, Convin can tailor its responses accordingly, leading to more personalization and contextually relevant interactions.
- Contextual Understanding: Convin goes beyond fundamental sentiment analysis by considering the broader context of conversations. It considers previous interactions, user history, and contextual cues to provide more accurate sentiment analysis results and responses.
- Adaptive Learning: Convin continuously learns and improves its sentiment analysis capabilities through adaptive learning techniques. By analyzing user feedback and interaction patterns, Convin refines its models over time, leading to better accuracy and performance in sentiment analysis tasks.
- Enhanced Customer Engagement: By accurately gauging user sentiments and responding appropriately, Convin enhances customer engagement and satisfaction. Whether addressing customer concerns, providing recommendations, or delivering personalized experiences, Convin's role in sentiment analysis contributes to overall positive customer experiences.
Convin's integration of conversational AI technology with sentiment analysis capabilities empowers businesses to gain deeper insights into customer sentiments, drive more personalized interactions, and ultimately improve overall customer satisfaction and engagement.
To witness the capabilities of AI-based sentiment analysis in action, why not view a demo of our pioneering sentiment analysis platform? Experience how it can revolutionize your business by unlocking real-time actionable insights from textual data.
FAQs
1. How is AI used in sentiment analysis?
AI processes vast amounts of textual data to classify sentiments as positive, negative, or neutral, enhancing accuracy and efficiency in sentiment analysis.
2. How is NLP used in sentiment analysis?
NLP techniques enable the interpretation of language nuances, facilitating the understanding of sentiments expressed in textual data for sentiment analysis.
3. How is machine learning used in sentiment analysis?
Machine learning algorithms analyze patterns in text data to classify sentiments and continuously improve accuracy through iterative learning processes.
4. How does Gen AI work?
Gen AI utilizes advanced AI technologies, including machine learning and NLP, to understand and interpret human-like interactions, enabling sophisticated applications such as sentiment analysis to provide insights and drive decision-making.