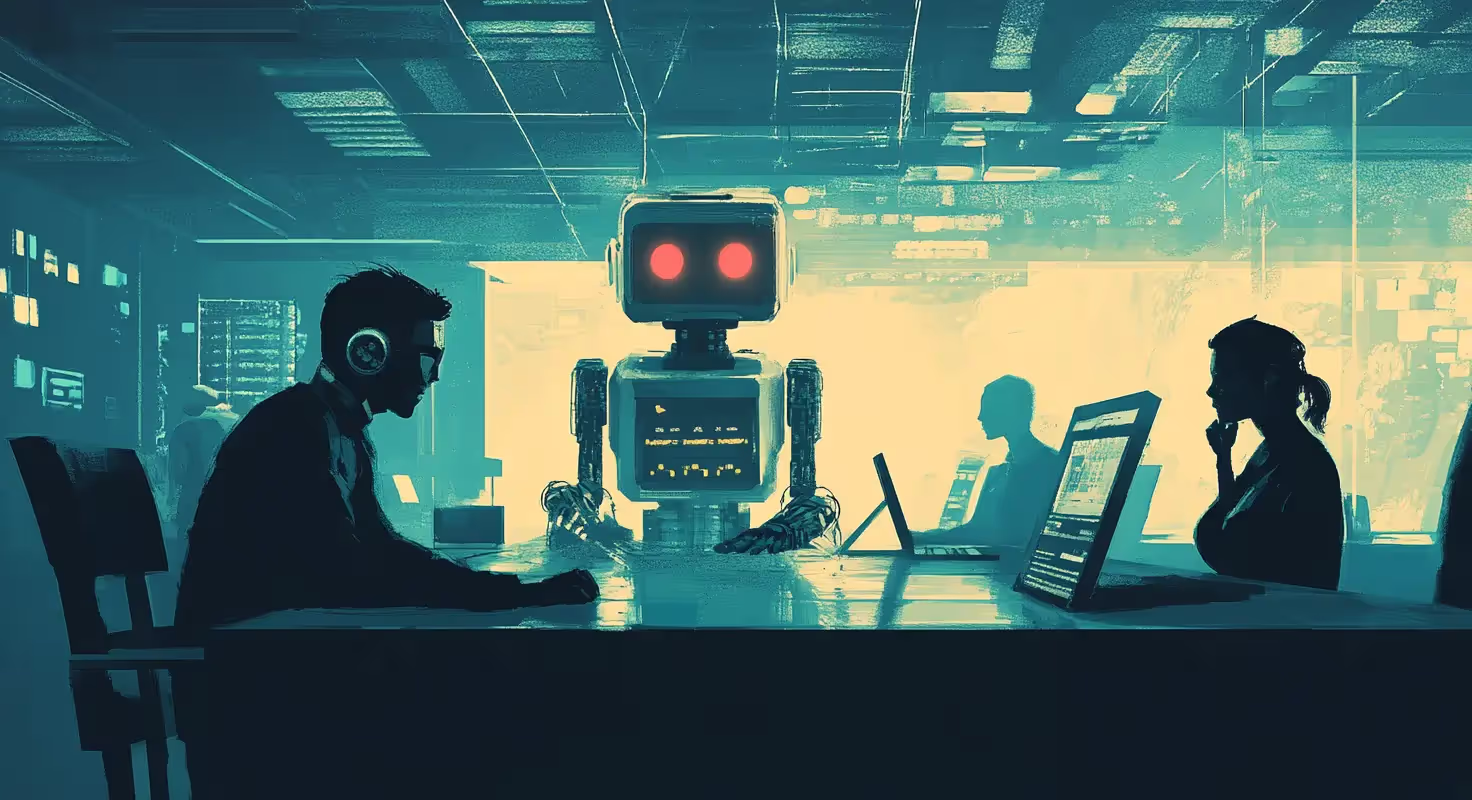
Training conversational AI is becoming essential for businesses that want to improve customer communication. Call centers, sales teams, and support departments face growing pressure to automate conversations while delivering high-quality service.
Conversational AI training teaches AI to understand and respond to human language naturally. This process involves gathering and labeling conversation data and then using it to train the AI. Proper training helps businesses automate customer support, sales, and other services without sacrificing the quality of those interactions.
According to a 2023 Gartner case study, companies that introduced conversational AI into their customer service saw a 25% drop in response times and a 40% rise in customer satisfaction.
In this blog, we’ll walk you through the steps to train conversational AI, explain why quality data is critical, and explain how it’s used in real-world call center and sales settings. Want to know how AI can transform your business? Keep reading to find out more.
Automate 1000s of calls at once with Convin. Book a demo to see how.
What are Conversational AI Datasets?
Conversational AI datasets are carefully curated collections of human interactions, often in text or voice format, used to train AI models. These datasets must cover various conversation types, from casual inquiries to more complex problem-solving scenarios. The AI uses these datasets to learn how to interpret human language, recognize intent, and provide accurate responses.
Types of Conversational AI Datasets
- Text-based datasets include chat logs, emails, and transcripts of written conversations. They help in training AI models for chatbots or text-based systems.
- Voice datasets: These are recordings of spoken interactions. Voice datasets are particularly important for virtual assistants and voice-based AI systems. They allow the AI to understand accents, tones, and natural speech variations.
Importance of Quality Datasets
Quality is critical when selecting conversational AI datasets. A dataset filled with unclear or irrelevant conversations will result in an AI model that needs help to deliver meaningful interactions. High-quality datasets must be:
- Diverse: To handle different languages, accents, and industries.
- Accurate: Properly labeled to ensure the AI understands intent.
- Balanced: Including a range of simple and complex conversations for broad applicability.
Conversational AI systems will underperform without a solid dataset, leading to ineffective customer interactions.
Steps to Train a Conversational AI Model
Training a conversational AI model is a multi-step process. Each stage ensures the AI understands human language and can deliver contextually relevant responses. Below are the essential steps involved:
Step 1: Collecting Conversational AI Training Data
The first step in training conversational AI is gathering a wide range of conversation data. This could include historical chat logs, recorded phone calls, or other interactions between agents and customers. The goal is to capture a variety of conversational tones, styles, and contexts to ensure the AI can handle real-world scenarios.
Data diversity is crucial. A well-trained AI must be able to interact with customers of different backgrounds, speaking different languages or dialects. For example, an AI in a global support center must understand American English and regional variations and accents. The more diverse the dataset, the more robust the AI becomes.
Step 2: Data Labeling and Annotation
Once the data is collected, it must be labeled and annotated. This process involves tagging each part of the conversation with its corresponding intent or meaning. For example, if a customer asks for a product price, the label might be "price inquiry." This labeling is critical because it helps the AI understand what customers are asking for.
Annotation adds further depth to the data. Sentiment analysis is often included at this stage, helping the AI recognize if a customer is frustrated, neutral, or satisfied. Accurate annotation allows the AI to respond appropriately, improving customer satisfaction.
Step 3: Preprocessing the Conversational AI Datasets
Preprocessing is a crucial step in which the collected data is cleaned and prepared for training. This includes removing noise from voice data, standardizing text formats, and ensuring that only relevant parts of conversations are used. Preprocessing might involve filtering background noise or adjusting volume levels in voice data.
Another part of preprocessing is normalizing the language used in conversations. Slang, abbreviations, and informal language must be translated into formats the AI can recognize and learn from. This step ensures that the AI model isn’t confused by colloquial language or regional slang.
Step 4: Training the Conversational AI Model
Once the data is prepared, it's time to train the model. The AI is fed these labeled datasets and uses them to learn how to interpret and respond to similar conversations in the future. Deep learning models, neural networks, or other algorithms are used during this phase to fine-tune the AI's responses.
The training process involves teaching the AI to recognize what customers are asking and the context behind their questions. For instance, if a customer asks, "Can I get a refund?" rather than "Can I get more information on returns?" the AI must differentiate between a direct request and an inquiry for details.
Step 5: Fine-Tuning and Continuous Learning
After the initial training, the AI model must be continuously fine-tuned based on real-world interactions. This stage is critical because language and customer expectations evolve. Regular updates and retraining using new data help the AI improve its performance and accuracy.
For example, if the AI frequently misinterprets a certain query type, additional training can correct these errors. Continuous learning ensures the AI remains relevant and accurate, providing better customer experiences over time.
Step 6: Testing and Validation of AI Models
Before deploying the AI system, it must be tested and validated. This phase involves running the AI through various simulated customer interactions to check its performance. Testing ensures the AI responds appropriately to queries and can handle complex conversations.
Validation also involves stress-testing the AI under high-volume scenarios, ensuring it can maintain accuracy and speed even during peak times. Identifying weak spots in the AI’s understanding at this stage allows developers to retrain and improve the model before it goes live.
Results first, payment later
This blog is just the start.
Unlock the power of Convin’s AI with a live demo.

Real-World Applications of Conversational AI in Sales and Support
Conversational AI offers many benefits in both sales and support environments. By automating routine interactions, AI frees human agents to focus on high-priority tasks, increasing productivity and customer satisfaction.
1. Sales Training for Real Estate Agents Using Conversational AI
AI can qualify leads in real estate by gathering initial information from potential buyers. By automating these early interactions, agents save time and focus on serious prospects. AI-driven systems can ask key questions to understand customer needs, schedule property viewings, and follow up automatically. This saves agents hours of manual work while improving the customer journey.
2. Inbound Sales Training with AI-Powered Systems
Conversational AI can efficiently handle the influx of inquiries for inbound sales teams. By responding to basic queries about products or services, AI enables sales teams to prioritize their focus on high-value opportunities. This approach is especially useful for teams dealing with large volumes of incoming inquiries where time is of the essence.
3. The Impact of Conversational AI in Inbound Enquiry Sales Training
Conversational AI streamlines inbound inquiry processes by automating responses to common customer questions. It helps ensure that potential sales opportunities are not missed due to human response delays. With AI handling routine inquiries, sales agents can concentrate on nurturing high-potential leads and converting them into customers.
Convin’s AI Phone Calls: A Game Changer in Call Centers
Convin’s AI-powered VoiceBot is redefining customer interaction in call centers. It enables businesses to automate sales, support, and follow-up calls at scale. By integrating AI-driven voice agents, businesses can significantly improve productivity and customer engagement.
Key Stats About Convin’s AI Virtual Agent:
- 100% call automation: Convin’s AI virtual agent automates the entire call process, handling inbound and outbound calls without human intervention.
- 60% increase in sales-qualified leads: AI improves lead qualification accuracy, ensuring only high-quality leads are passed to sales teams.
- 50% reduction in communication errors: Automated systems reduce human mistakes, providing more consistent and accurate responses.
Convin’s virtual agent excels in delivering personalized, multilingual experiences, tailoring its responses based on customer location, language, and preferences. Its ability to handle multiple calls simultaneously ensures no customer is left waiting, improving overall call center efficiency.
Convin reduces errors by 50%. Get your personalized demo now.
Conversational AI: The Key to Scaling Call Center Operations
Training conversational AI is an intricate process that requires careful planning, from data collection to continuous learning. High-quality, diverse datasets form the backbone of an effective conversational AI model, ensuring it performs well in various real-world scenarios. As businesses continue to leverage AI, the potential for automation in call centers and sales teams will only grow, allowing for improved efficiency and customer experiences.