In today’s fast-paced world, contact centers are under constant pressure to improve efficiency and customer satisfaction. One of the most promising technologies for achieving this is multimodal AI. However, many businesses are still grappling with understanding how to implement and optimize it effectively.
Multimodal AI refers to artificial intelligence systems that can process and analyze data from multiple sources, such as text, speech, and images, to provide smarter, more contextual interactions. As contact centers struggle with improving response times and personalization, multimodal AI offers a solution by seamlessly integrating various data streams.
So, how can contact centers leverage multimodal AI to enhance their operations? Let’s explore the potential of this transformative technology and how it can revolutionize your contact center performance. Keep reading to discover how multimodal AI works.
Explore Convin AI’s multimodal capabilities! Schedule your demo today.
What is Multimodal AI and How Does It Work?
Multimodal AI is an advanced artificial intelligence technology that integrates multiple data types—text, voice, images, and video—to analyze and respond to customer interactions.Â
Unlike traditional AI models, which focus on just one form of data, multimodal AI processes combine these different data sources for a more accurate and nuanced understanding. This capability enables systems to interpret human interactions across various channels, providing a more seamless and comprehensive experience for customers and agents alike.
The true power of multimodal AI lies in its ability to understand and respond to a range of customer inputs, regardless of the medium. Merging information from various sources in real-time offers more relevant and context-aware solutions, enhancing the overall customer experience.
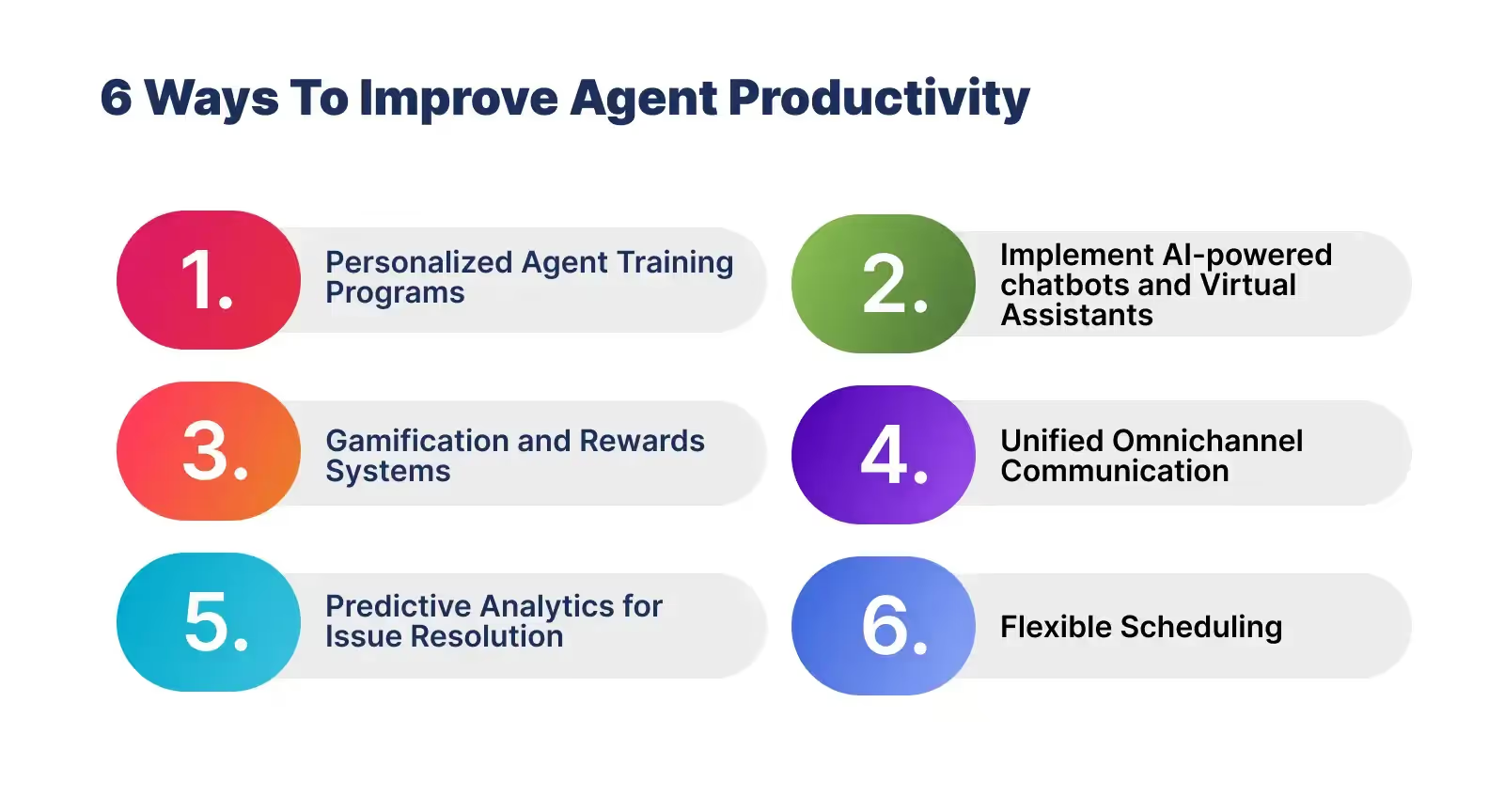
Multimodal AI Models and Their Key Components
To grasp the full potential of multimodal AI, it’s essential to understand the components that make up these models.Â
Multimodal AI typically involves four primary components: speech, text, vision, and audio. Each component processes data differently, but when integrated, they work harmoniously to improve customer interaction and agent performance.
- Speech Recognition: Converts voice interactions into text, allowing AI systems to understand spoken language.
- Text Processing: Analyzes written content, including chats and emails, to derive meaning.
- Visual Data: Image and video analysis, often used in advanced customer support scenarios like visual assistance.
- Audio Processing: Analyzes tone, sentiment, and speech patterns, offering more profound insights into customer emotions.
These components combine to form multimodal AI models that enable contact centers to handle complex interactions more efficiently.Â
By integrating and analyzing data from multiple sources, these models can offer real-time support that’s more personalized and contextually aware.
Concepts of Multimodality
The concepts of multimodality refer to integrating multiple forms of data (e.g., text, speech, visuals) in communication systems. Some of the essential aspects of multimodality include:
- Interconnectedness: Different modalities (like voice and text) work together to enhance understanding and user experience.
- Data Fusion: Combines multiple data types to provide a more holistic interpretation of information.
- Context Awareness: The concepts of multimodality enable systems to understand and respond to context more effectively.
- User Engagement: By using varied modalities, systems can cater to diverse user preferences, improving overall interaction.
Multimodal Generative AI
Multimodal generative AI combines multiple data types to create new outputs, such as text, images, or speech. Some of the features include:
- Capabilities: It can generate human-like responses across various media, improving customer interaction quality.
- Real-time Applications: Multimodal generative AI generates personalized content, from chatbot responses to tailored email replies.Â
- Integration: It integrates text, voice, and image data to create more context-aware and dynamic interactions.
- Efficiency Boost: By automating content creation, multimodal generative AI can enhance agent productivity and reduce response times.
Now that we've explored multimodal AI's core components, let's dive into how it contrasts with multimodal interfaces and their unique roles.
Power collection calls with tone and script with Convin AI!
Multimodal AI vs. Multimodal Interfaces: Key Differences
While both multimodal AI and multimodal interfaces involve multiple forms of data, the key difference lies in their functionality and application.Â
- Multimodal AI is the underlying model that processes and analyzes various data types to drive decision-making and improve outcomes.Â
- On the other hand, multimodal interfaces are platforms or systems that allow users to interact with these AI models.
- Multimodal AI models focus on improving the backend processes of contact centers by enhancing AI’s ability to understand and respond to diverse input.Â
- In contrast, multimodal interfaces enable users—agents and customers alike—to engage with AI through various channels, such as voice, text, or video.Â
While the interface is the medium for interaction, the AI model handles the complexity of understanding and processing the data.
The distinction between these two elements is crucial for contact center leaders, as both play a role in ensuring that customers receive timely and accurate assistance, but their focus and implementation differ significantly.
Multimodal Pipeline
A multimodal pipeline processes and integrates multiple types of data for analysis. Here are some other important factors of multimodal pipeline:
- Workflow: The pipeline handles speech, text, and visual data in parallel, ensuring seamless processing.Â
- Efficiency: It automates data flow, reducing manual intervention and speeding up customer service operations.
- Real-time Insights: The multimodal pipeline provides real-time analysis, improving decision-making and agent performance.Â
- Application: Used in customer support to analyze and respond to queries faster by combining data sources.
To better understand how multimodal AI and interfaces work together, it's important to explore their distinct roles in improving customer and agent experiences.
Experience next-gen customer support with Convin AI!
‍
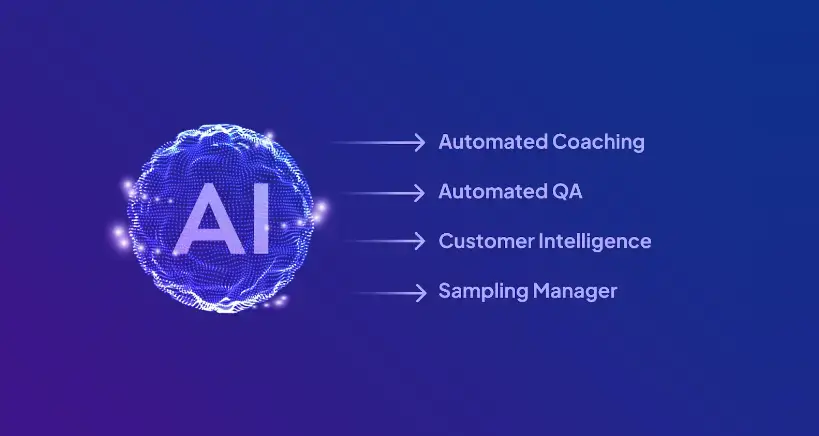
This blog is just the start.
Unlock the power of Convin’s AI with a live demo.

Impact of Multimodal AI on CX and Support
Multimodal AI is making significant strides in enhancing the customer experience by enabling smarter, more personalized support. Combining data from different modalities offers a level of context that traditional AI systems can't match.Â
This ability allows for faster issue resolution, a more personalized service, and a smoother customer experience across multiple channels.
For instance, multimodal AI models can analyze customer tone, words, and facial expressions during calls or video chats to assess sentiment and tailor responses accordingly. This results in more empathetic and efficient customer support and higher customer satisfaction.Â
By interpreting diverse data sources simultaneously, multimodal AI ensures no critical information is overlooked, leading to a more accurate understanding of each customer’s needs.
As the demand for personalized customer support grows, multimodal AI will become even more indispensable in contact centers, driving better outcomes.
Multimodal AI Models Enhance Agent Performance
Multimodal AI is not only beneficial for customers but also plays a crucial role in improving agent performance. By providing real-time guidance and insights, multimodal AI models assist agents in making more informed decisions during interactions.
This helps reduce errors, enhance productivity, and ensure that agents have the necessary tools to resolve customer issues effectively.
For example, multimodal AI can analyze a customer’s speech patterns, emotions, and query context to provide agents with relevant suggestions or prompts. This reduces the need for extensive training, as agents can rely on the AI to guide them through complex interactions.Â
Furthermore, by automating specific tasks, multimodal AI models allow agents to focus on more meaningful and high-impact aspects of customer service.
Ultimately, this results in quicker response times, better problem-solving, and a more efficient workforce, which is critical for customer and business success in the contact center space.
Deliver seamless CX using Convin’s AI-powered voice and chat! Book demo.
Wrapping Up Multimodal AI
Multimodal AI revolutionizes contact centers' operations, enhancing customer and agent experiences. By integrating multiple data sources and offering real-time support, multimodal AI models provide actionable insights that drive better decision-making and improve efficiency.Â
As this technology evolves, contact center leaders can expect further advancements to transform customer support delivery. Whether through better customer personalization, more efficient agent performance, or streamlined operations, multimodal AI is shaping the future of contact centers.
Explore how multimodal AI drives performance with Convin AI! Schedule a demo now.
FAQs
1. How does Multimodal AI work?
‍Multimodal AI combines multiple data types—text, speech, images, and video—to create a unified understanding of a situation. Processing and analyzing these different modalities enables smarter, more accurate interactions in applications like customer support, chatbots, and virtual assistants.
2. How do multimodal neural networks work?
‍Multimodal neural networks integrate data from different sources, such as images, text, and sound, into a single model. These networks are trained to understand and process these diverse inputs, improving the AI’s ability to make predictions, recognize patterns, and provide contextually relevant responses across multiple platforms.
3. What is the cognitive theory that supports multimodal learning?
‍The cognitive theory supporting multimodal learning is based on the idea that humans process information through different sensory channels. Learners can better understand, retain, and apply information by engaging visual, auditory, and kinesthetic senses. This theory is key in developing multimodal AI and enhancing user interaction and learning.
4. Is GPT-4 multimodal?
‍Yes, GPT-4 is multimodal. It can process text and image inputs, allowing for more dynamic and versatile interactions. This enables GPT-4 to handle a broader range of tasks, such as interpreting and generating content based on visual and textual information.